Deep Deterministic Policy Gradient-Based Intelligent Task Offloading for Vehicular Computing With Priority Experience Playback
IEEE Transactions on Vehicular Technology(2024)
摘要
With the development of Internet of Vehicles (IoV) technology, users' demand for low latency and high-quality network services has increased. However, executing large computing tasks on vehicles with limited resources still faces significant challenges. To address these issues, users can offload computing tasks to nearby base stations or servers. Nevertheless, due to the dynamic and complex nature of the IoV environment, obtaining the optimal task offloading policy in real-time is a great challenge. In this work, first we establish a queuing model for the incoming task flow, then we propose a profit function for task computation and offloading that takes into account the delay, energy consumption, and the user's demand for quality of service (QoS). Considering the dynamic nature and the complexity of the IoV scenarios, we abstract the task offloading as a Markov Decision Process. We choose the Deep Deterministic Policy Gradient (DDPG) algorithm to make the task offloading decision and combine Priority Experience Playback (PER) mechanism to evaluate and select actions so as to output the optimal offloading policy. Experimental results indicate that the offloading decision in our proposed method converges more quickly compared to traditional algorithms.We also discussed the impact of priority experience replay mechanism, learning rate and other factors on reward values. Our proposed method can achieve the minimum latency and energy consumption, effectively adapt to environmental changes, and improve user service experience.
更多查看译文
关键词
Internet of Vehicles (IoV),deep reinforcement learning,task offloading,prioritized experience replay
AI 理解论文
溯源树
样例
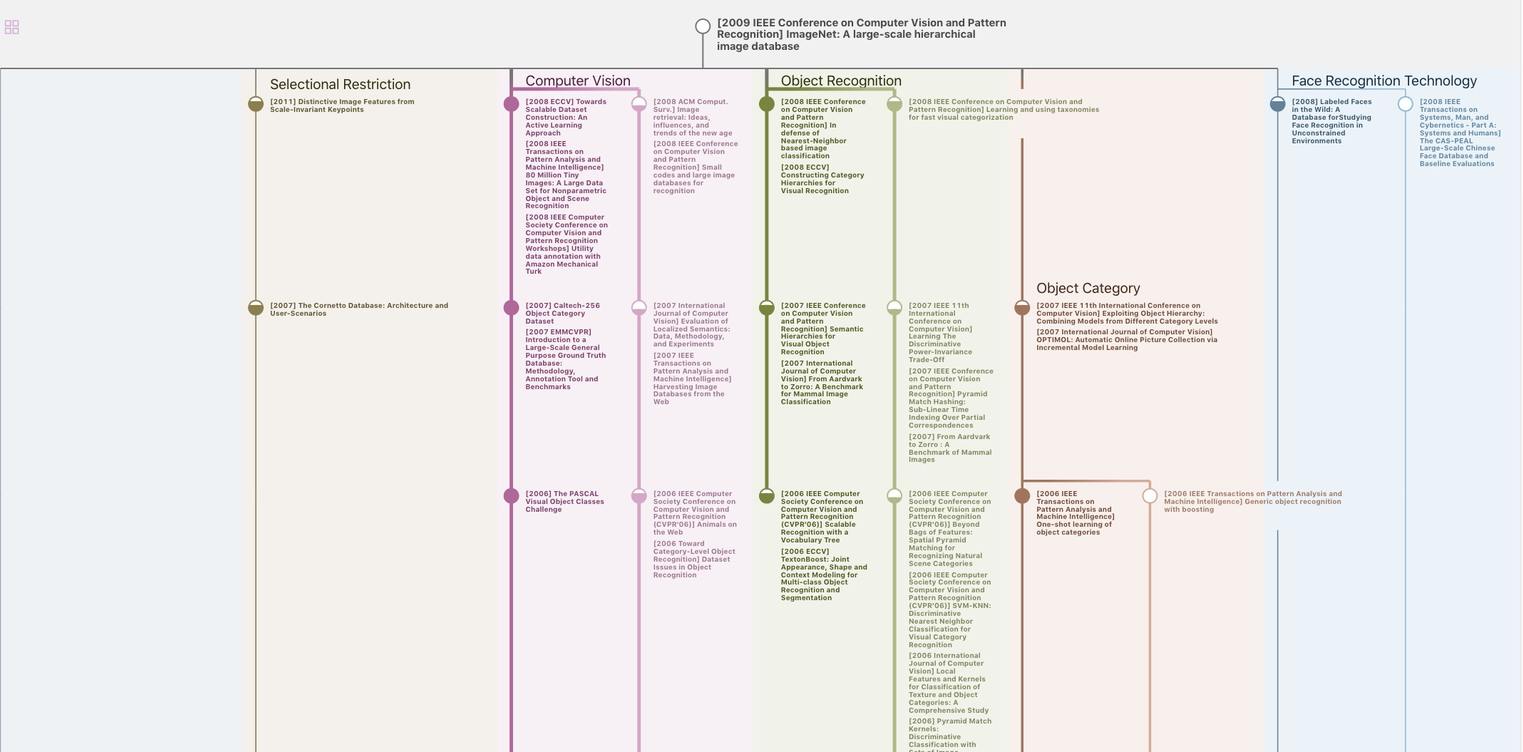
生成溯源树,研究论文发展脉络
Chat Paper
正在生成论文摘要