FusionFormer: A Concise Unified Feature Fusion Transformer for 3D Pose Estimation
AAAI 2024(2024)
摘要
Depth uncertainty is a core challenge in 3D human pose estimation, especially when the camera parameters are unknown. Previous methods try to reduce the impact of depth uncertainty by multi-view and/or multi-frame feature fusion to utilize more spatial and temporal information. However, they generally lead to marginal improvements and their performance still cannot match the camera-parameter-required methods. The reason is that their handcrafted fusion schemes cannot fuse the features flexibly, e.g., the multi-view and/or multi-frame features are fused separately. Moreover, the diverse and complicated fusion schemes make the principle for developing effective fusion schemes unclear and also raises an open problem that whether there exist more simple and elegant fusion schemes. To address these issues, this paper proposes an extremely concise unified feature fusion transformer (FusionFormer) with minimized handcrafted design for 3D pose estimation. FusionFormer fuses both the multi-view and multi-frame features in a unified fusion scheme, in which all the features are accessible to each other and thus can be fused flexibly. Experimental results on several mainstream datasets demonstrate that FusionFormer achieves state-of-the-art performance. To our best knowledge, this is the first camera-parameter-free method to outperform the existing camera-parameter-required methods, revealing the tremendous potential of camera-parameter-free models. These impressive experimental results together with our concise feature fusion scheme resolve the above open problem. Another appealing feature of FusionFormer we observe is that benefiting from its effective fusion scheme, we can achieve impressive performance with smaller model size and less FLOPs.
更多查看译文
关键词
CV: Biometrics, Face, Gesture & Pose,CV: 3D Computer Vision,CV: Motion & Tracking
AI 理解论文
溯源树
样例
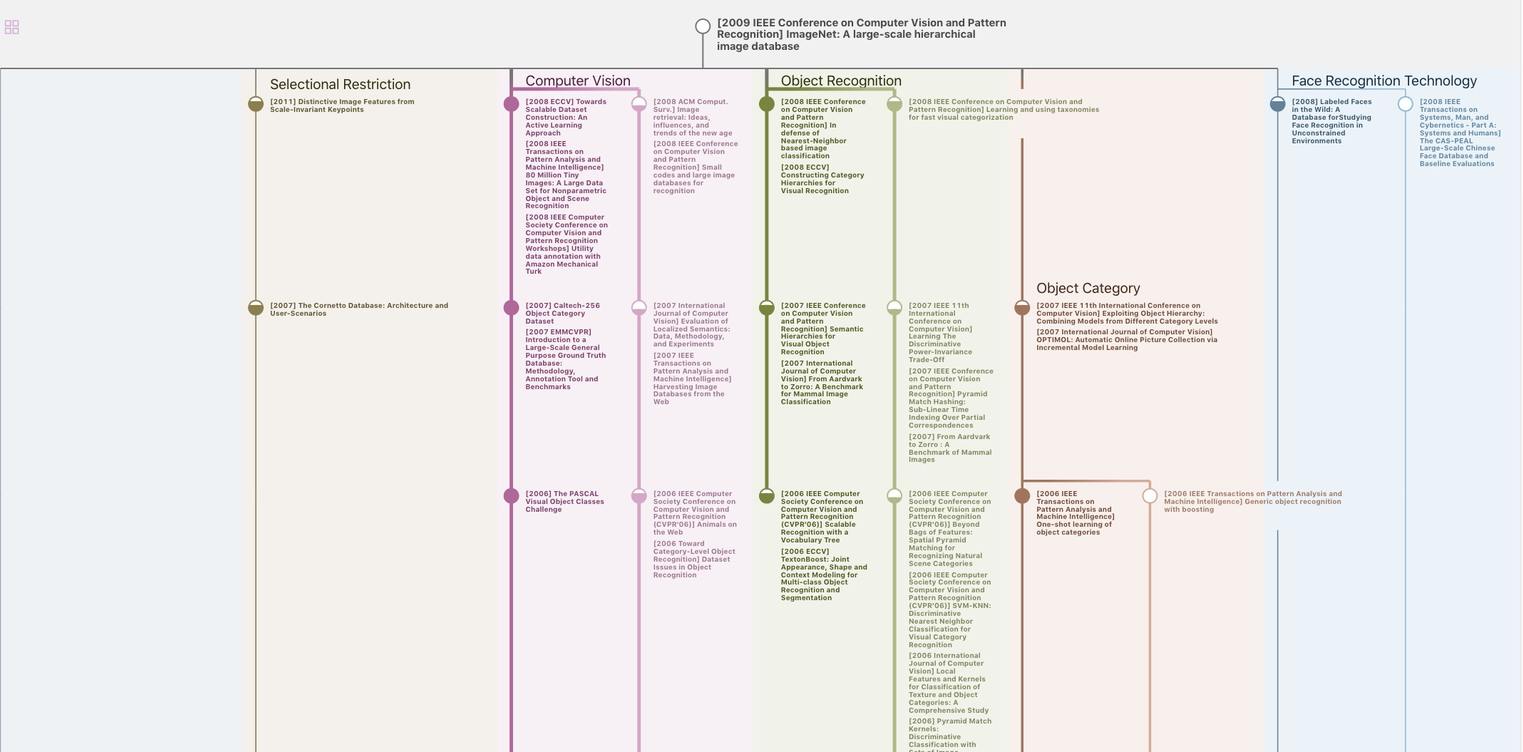
生成溯源树,研究论文发展脉络
Chat Paper
正在生成论文摘要