Limitations of Face Image Generation
AAAI 2024(2024)
Abstract
Text-to-image diffusion models have achieved widespread popularity due to their unprecedented image generation capability. In particular, their ability to synthesize and modify human faces has spurred research into using generated face images in both training data augmentation and model performance assessments. In this paper, we study the efficacy and shortcomings of generative models in the context of face generation. Utilizing a combination of qualitative and quantitative measures, including embedding-based metrics and user studies, we present a framework to audit the characteristics of generated faces conditioned on a set of social attributes. We applied our framework on faces generated through state-of-the-art text-to-image diffusion models. We identify several limitations of face image generation that include faithfulness to the text prompt, demographic disparities, and distributional shifts. Furthermore, we present an analytical model that provides insights into how training data selection contributes to the performance of generative models. Our survey data and analytics code can be found online at https://github.com/wi-pi/Limitations_of_Face_Generation
MoreTranslated text
Key words
ML: Ethics, Bias, and Fairness,CV: Bias, Fairness & Privacy,ML: Deep Generative Models & Autoencoders
AI Read Science
Must-Reading Tree
Example
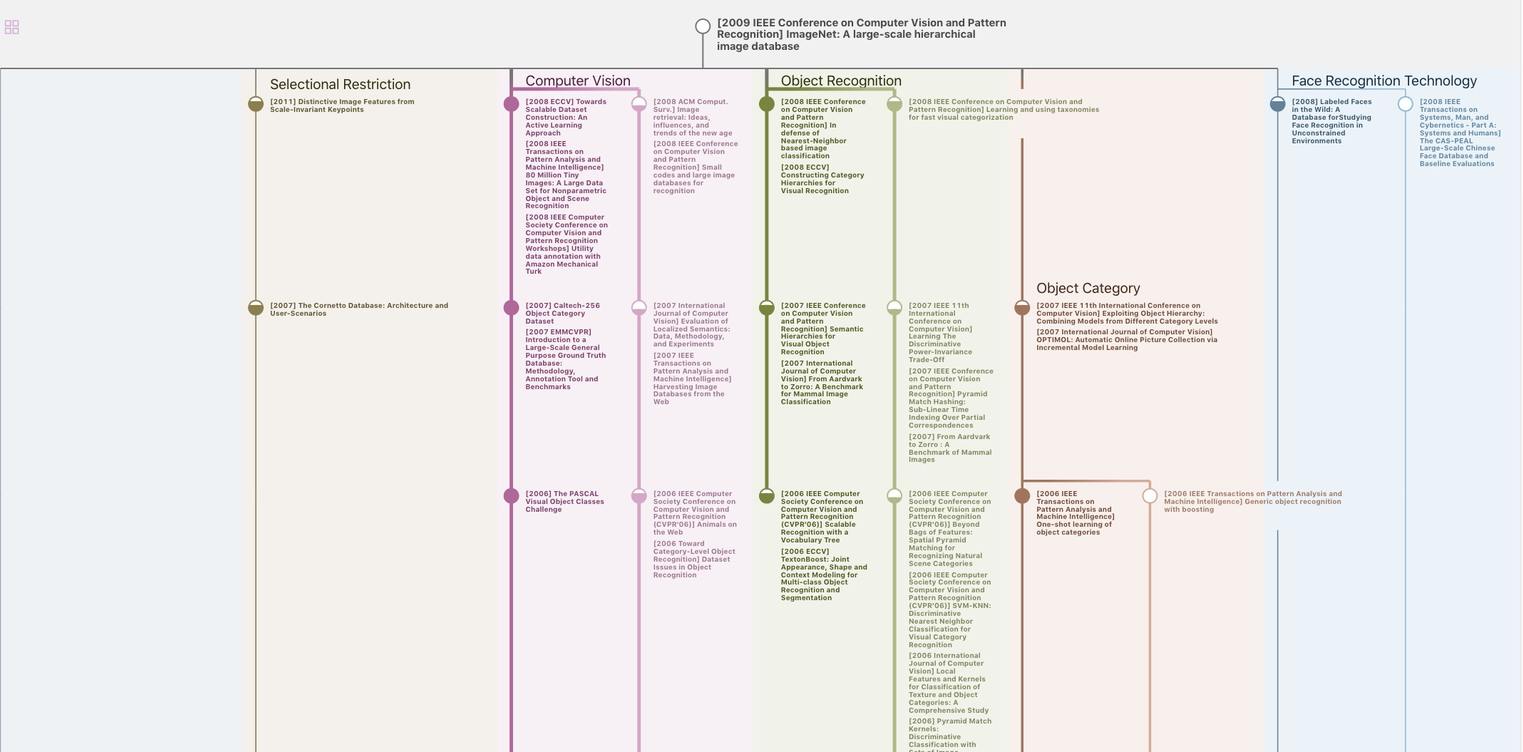
Generate MRT to find the research sequence of this paper
Chat Paper
Summary is being generated by the instructions you defined