Towards Making Learnware Specification and Market Evolvable
AAAI 2024(2024)
摘要
The learnware paradigm aims to establish a market of numerous well-performed machine learning models, enabling users to leverage existing helpful models for their tasks instead of starting from scratch. Each learnware in the market is a model submitted by its developer, associated with a specification generated with the help of learnware market, representing the model's specialty and utility and enabling it to be identified for new user tasks. As the market continuously scales up, accommodating an ever-increasing number of learnwares, the critical challenge of the learnware paradigm is to effectively and efficiently identify the most helpful learnware(s) for a new user task without accessing the user's raw data. In this paper, to achieve increasingly accurate learnware characterization and identification along with a growing number of learnwares in the market, we propose an approach called Evolvable Learnware Specification with Index (ELSI). Specifically, based on the key idea of leveraging the task information within learnware specifications, we tackle the challenge of ascertaining the capabilities of models beyond their original training tasks, thereby enabling learnware specifications and the entire market to evolve continuously. Furthermore, through organizing learnwares and constructing specification indexes, we design a practical procedure to accurately and efficiently identify helpful learnwares without examining the entire market. Theoretical analysis and extensive experiments on a learnware market prototype encompassing thousands of models and covering six real-world scenarios validate the effectiveness and efficiency of our approach.
更多查看译文
关键词
ML: Other Foundations of Machine Learning,ML: Scalability of ML Systems,ML: Classification and Regression,ML: Transfer, Domain Adaptation, Multi-Task Learning
AI 理解论文
溯源树
样例
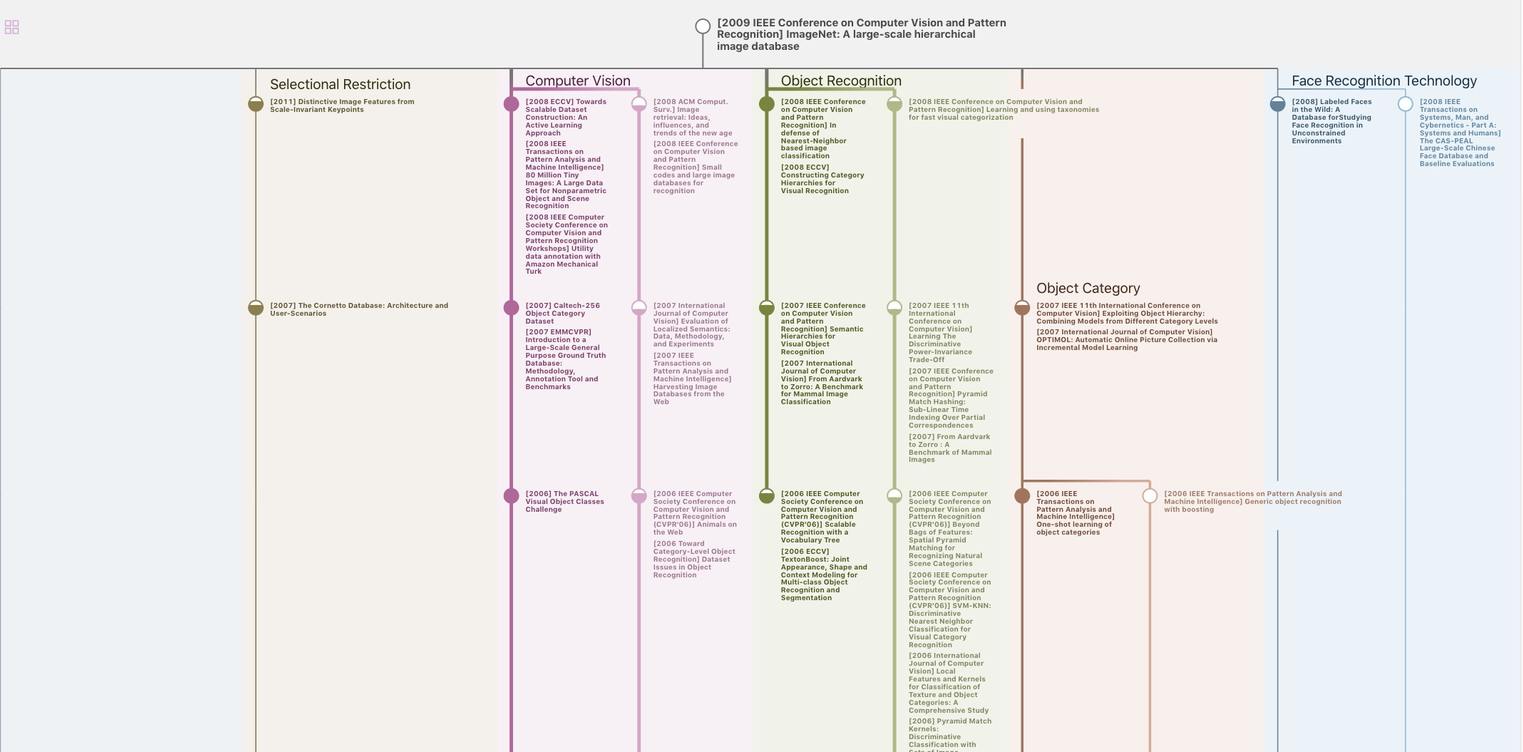
生成溯源树,研究论文发展脉络
Chat Paper
正在生成论文摘要