Data driven multi-objective design for low-carbon self-compacting concrete considering durability
JOURNAL OF CLEANER PRODUCTION(2024)
Abstract
Self-Compacting Concrete (SCC) offers remarkable benefits in modern engineering. However, traditional SCC design faces challenges, necessitating a reduction in carbon emissions for enhanced sustainability and a shift towards multi-performance collaborative design. This study proposed an intelligent and interpretable approach for multi-objective low-carbon SCC design. By combining machine learning and optimization algorithm, key factors including sustainability, rheology, workability, strength, durability, and cost were simultaneously addressed. Partial Dependence Plots was employed for model interpretation and feature impacts reveal. The proposed three-objective optimization exhibited superior efficiency, achieving comprehensive optimization efficiencies of 42.3%. In the context of meeting other performance requirements, C40 and C50 SCC optimized using this method exhibited a significant reduction of 18.9% and 10.1% in embodied carbon. This study aims to establish a versatile intelligent framework, rather than a specific model, capable of adapting to future iterations with evolving high-quality data, to achieve multi-objective SCC collaborative design. This advancement contributes to intelligent and low-carbon practices in concrete science and the construction industry.
MoreTranslated text
Key words
Low-carbon self-compacting concrete,Sustainability,Durability,Multi-objective design,Machine learning,Non-dominated sorting genetic algorithm
AI Read Science
Must-Reading Tree
Example
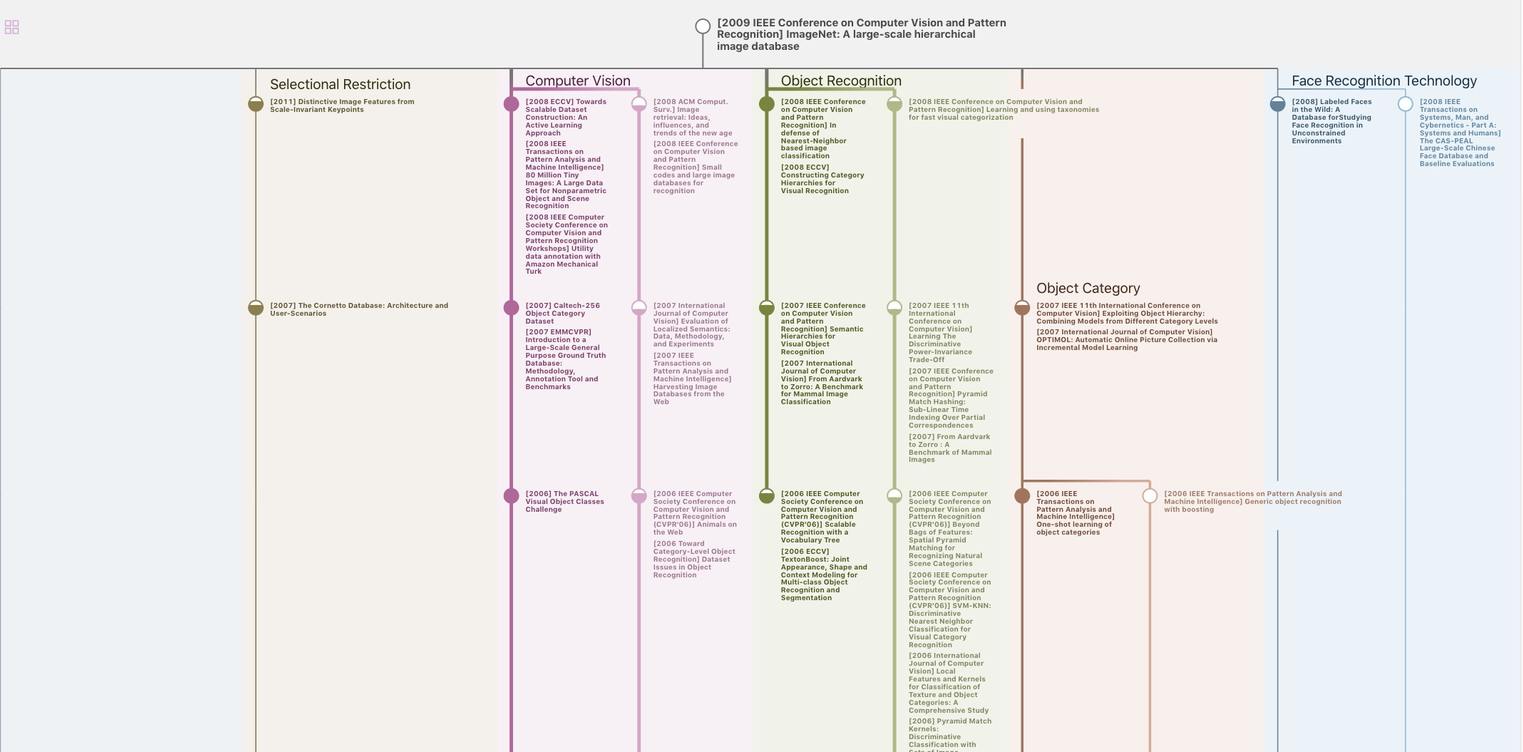
Generate MRT to find the research sequence of this paper
Chat Paper
Summary is being generated by the instructions you defined