SETEM: Self-ensemble training with Pre-trained Language Models for Entity Matching
Knowledge-Based Systems(2024)
摘要
Entity Matching (EM) aims to determine whether records in two datasets refer to the same real-world entity. Existing work often uses Pre-trained Language Models (PLMs) for feature representation, converting EM to a binary classification task. However, due to the dependence of PLMs on large labeled datasets and the overlap between train and test sets in current EM benchmarks, these methods often underperform in real-world scenarios (e.g., small data size, hard negative samples, and unseen entities). To address the limitations of existing methods, we propose SETEM, a self-ensemble training method leveraging the stability and strong generalization of ensemble models to tackle these challenges in real-world scenarios. Additionally, we develop a faster training method for low-resource applications. Experiments on benchmark datasets show that SETEM outperforms Ditto and HierGAT on the F1 score. In particular, SETEM shows the greatest advantage with small datasets and a high proportion of unseen entities in the test set, achieving up to a 9.61% F1 score increment over baselines on the WDC dataset.
更多查看译文
关键词
Entity Matching,Pre-trained Language Model,Self-ensemble,Knowledge distillation,Mixout
AI 理解论文
溯源树
样例
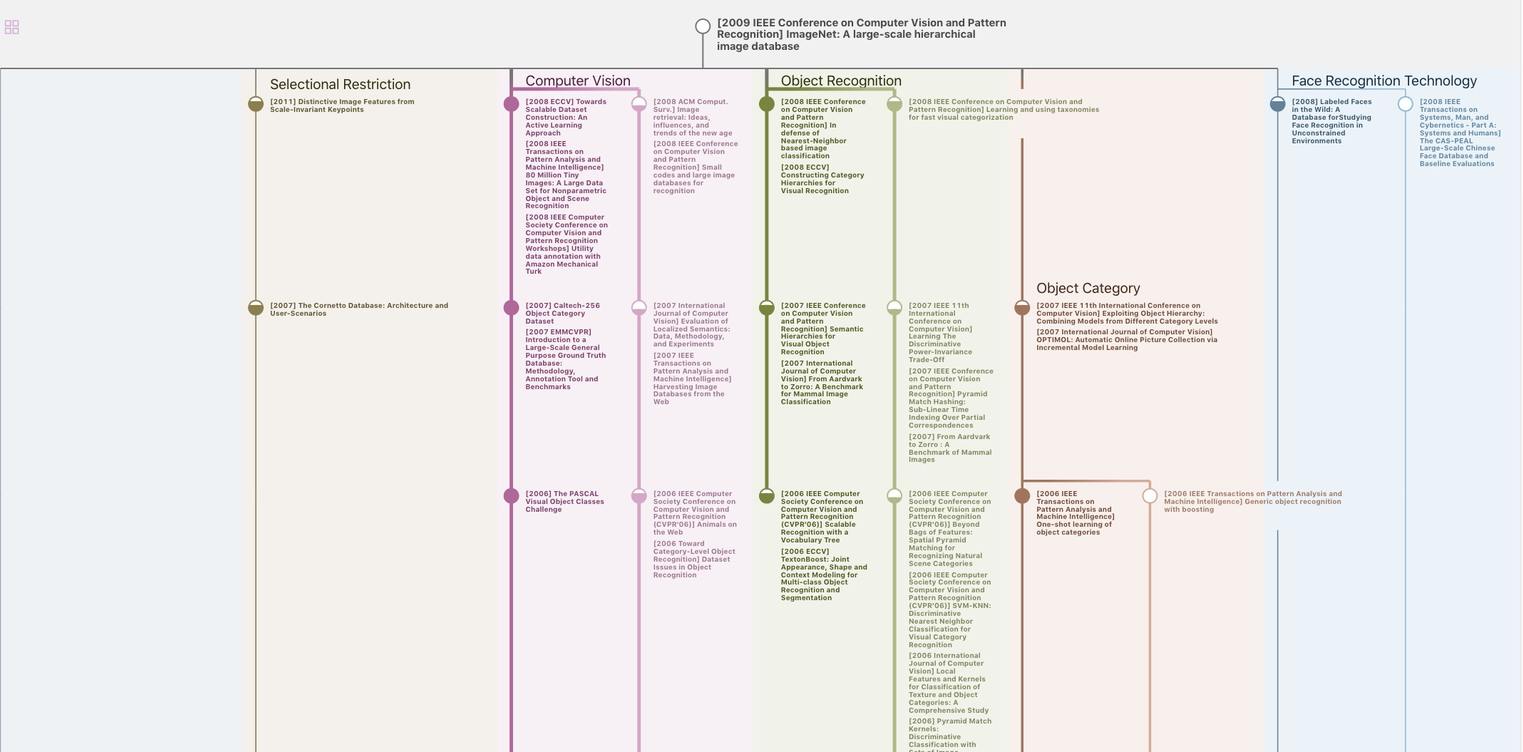
生成溯源树,研究论文发展脉络
Chat Paper
正在生成论文摘要