Enhancing Cross-Dataset EEG Emotion Recognition: A Novel Approach with Emotional EEG Style Transfer Network
CoRR(2024)
摘要
Recognizing the pivotal role of EEG emotion recognition in the development of
affective Brain-Computer Interfaces (aBCIs), considerable research efforts have
been dedicated to this field. While prior methods have demonstrated success in
intra-subject EEG emotion recognition, a critical challenge persists in
addressing the style mismatch between EEG signals from the source domain
(training data) and the target domain (test data). To tackle the significant
inter-domain differences in cross-dataset EEG emotion recognition, this paper
introduces an innovative solution known as the Emotional EEG Style Transfer
Network (E^2STN). The primary objective of this network is to effectively
capture content information from the source domain and the style
characteristics from the target domain, enabling the reconstruction of stylized
EEG emotion representations. These representations prove highly beneficial in
enhancing cross-dataset discriminative prediction. Concretely, E^2STN
consists of three key modules—transfer module, transfer evaluation
module, and discriminative prediction module—which address the
domain style transfer, transfer quality evaluation, and discriminative
prediction, respectively. Extensive experiments demonstrate that E^2STN
achieves state-of-the-art performance in cross-dataset EEG emotion recognition
tasks.
更多查看译文
AI 理解论文
溯源树
样例
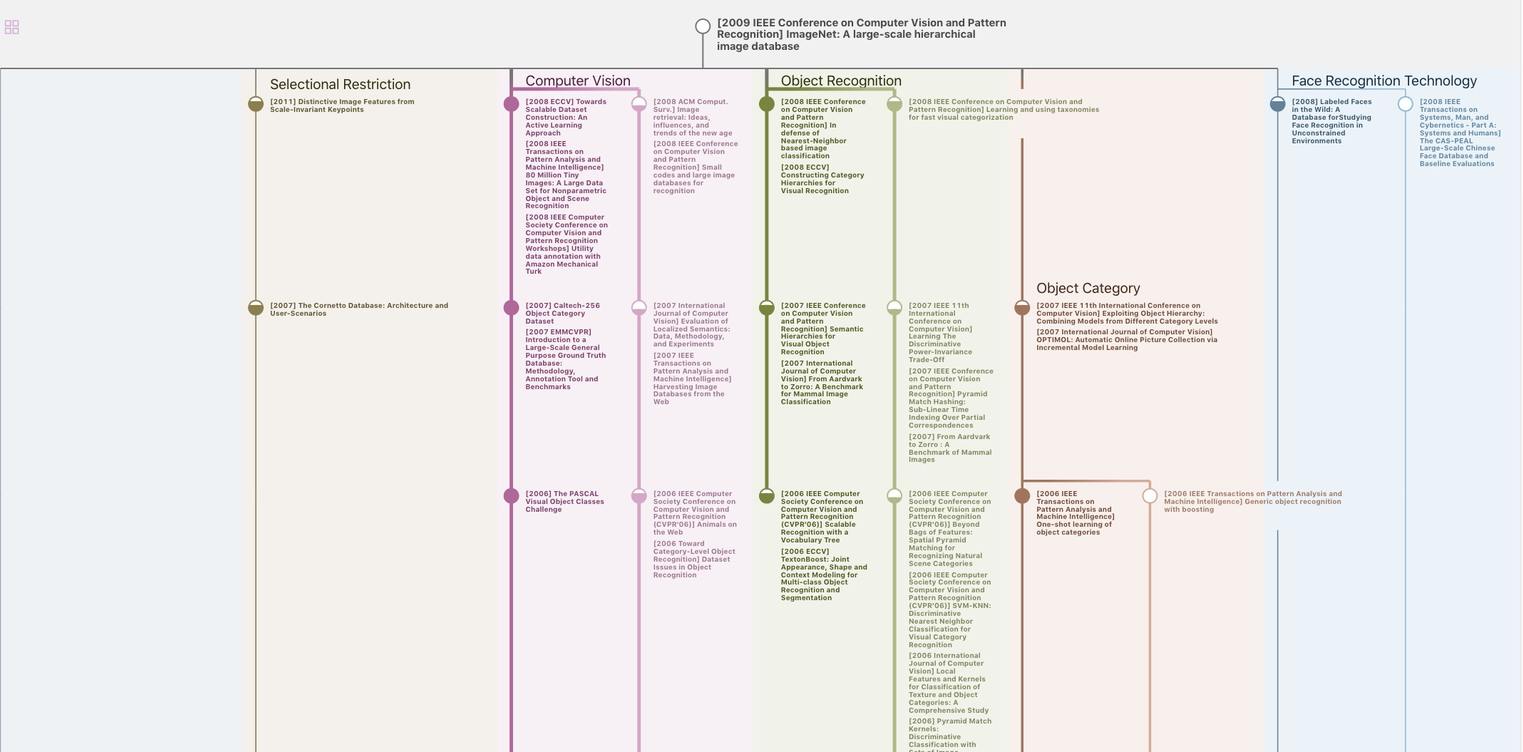
生成溯源树,研究论文发展脉络
Chat Paper
正在生成论文摘要