Exploit High-Dimensional RIS Information to Localization: What Is the Impact of Faulty Element?
arxiv(2024)
摘要
This paper proposes a novel localization algorithm using the reconfigurable
intelligent surface (RIS) received signal, i.e., RIS information. Compared with
BS received signal, i.e., BS information, RIS information offers higher
dimension and richer feature set, thereby providing an enhanced capacity to
distinguish positions of the mobile users (MUs). Additionally, we address a
practical scenario where RIS contains some unknown (number and places) faulty
elements that cannot receive signals. Initially, we employ transfer learning to
design a two-phase transfer learning (TPTL) algorithm, designed for accurate
detection of faulty elements. Then our objective is to regain the information
lost from the faulty elements and reconstruct the complete high-dimensional RIS
information for localization. To this end, we propose a transfer-enhanced
dual-stage (TEDS) algorithm. In Stage I, we integrate the CNN and
variational autoencoder (VAE) to obtain the RIS information, which in
Stage II, is input to the transferred DenseNet 121 to estimate the
location of the MU. To gain more insight, we propose an alternative algorithm
named transfer-enhanced direct fingerprint (TEDF) algorithm which only requires
the BS information. The comparison between TEDS and TEDF reveals the
effectiveness of faulty element detection and the benefits of utilizing the
high-dimensional RIS information for localization. Besides, our empirical
results demonstrate that the performance of the localization algorithm is
dominated by the high-dimensional RIS information and is robust to unoptimized
phase shifts and signal-to-noise ratio (SNR).
更多查看译文
AI 理解论文
溯源树
样例
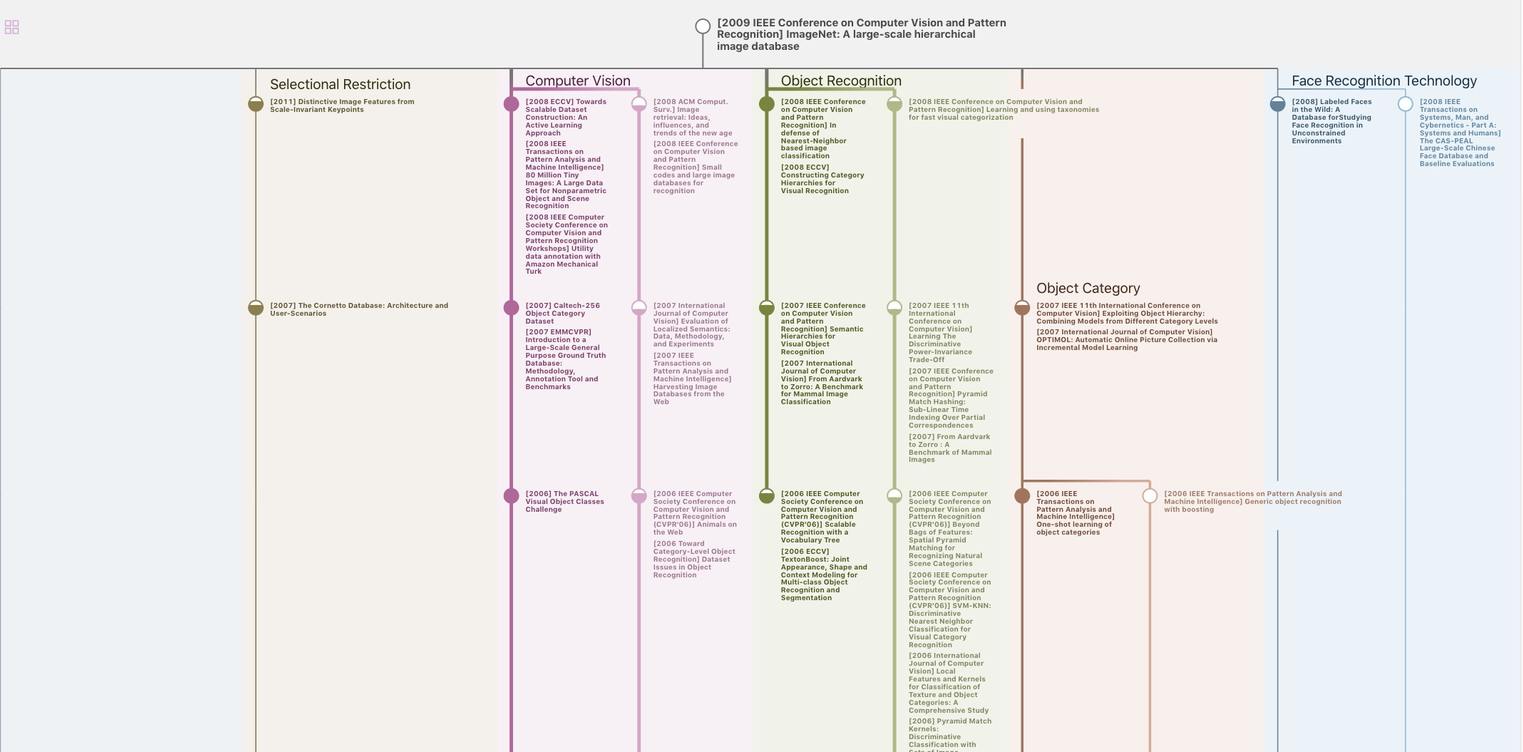
生成溯源树,研究论文发展脉络
Chat Paper
正在生成论文摘要