A Distributionally Robust Model Predictive Control for Static and Dynamic Uncertainties in Smart Grids
IEEE Transactions on Smart Grid(2024)
摘要
The integration of various power sources, including renewables and electric
vehicles, into smart grids is expanding, introducing uncertainties that can
result in issues like voltage imbalances, load fluctuations, and power losses.
These challenges negatively impact the reliability and stability of online
scheduling in smart grids. Existing research often addresses uncertainties
affecting current states but overlooks those that impact future states, such as
the unpredictable charging patterns of electric vehicles. To distinguish
between these, we term them static uncertainties and dynamic uncertainties,
respectively. This paper introduces WDR-MPC, a novel approach that stands for
two-stage Wasserstein-based Distributionally Robust (WDR) optimization within a
Model Predictive Control (MPC) framework, aimed at effectively managing both
types of uncertainties in smart grids. The dynamic uncertainties are first
reformulated into ambiguity tubes and then the distributionally robust bounds
of both dynamic and static uncertainties can be established using WDR
optimization. By employing ambiguity tubes and WDR optimization, the stochastic
MPC system is converted into a nominal one. Moreover, we develop a convex
reformulation method to speed up WDR computation during the two-stage
optimization. The distinctive contribution of this paper lies in its holistic
approach to both static and dynamic uncertainties in smart grids. Comprehensive
experiment results on IEEE 38-bus and 94-bus systems reveal the method's
superior performance and the potential to enhance grid stability and
reliability.
更多查看译文
关键词
Distributionally robust optimization,Wasserstein metric,tube-based stochastic model predictive control,static uncertainty,dynamic uncertainty,smart grid
AI 理解论文
溯源树
样例
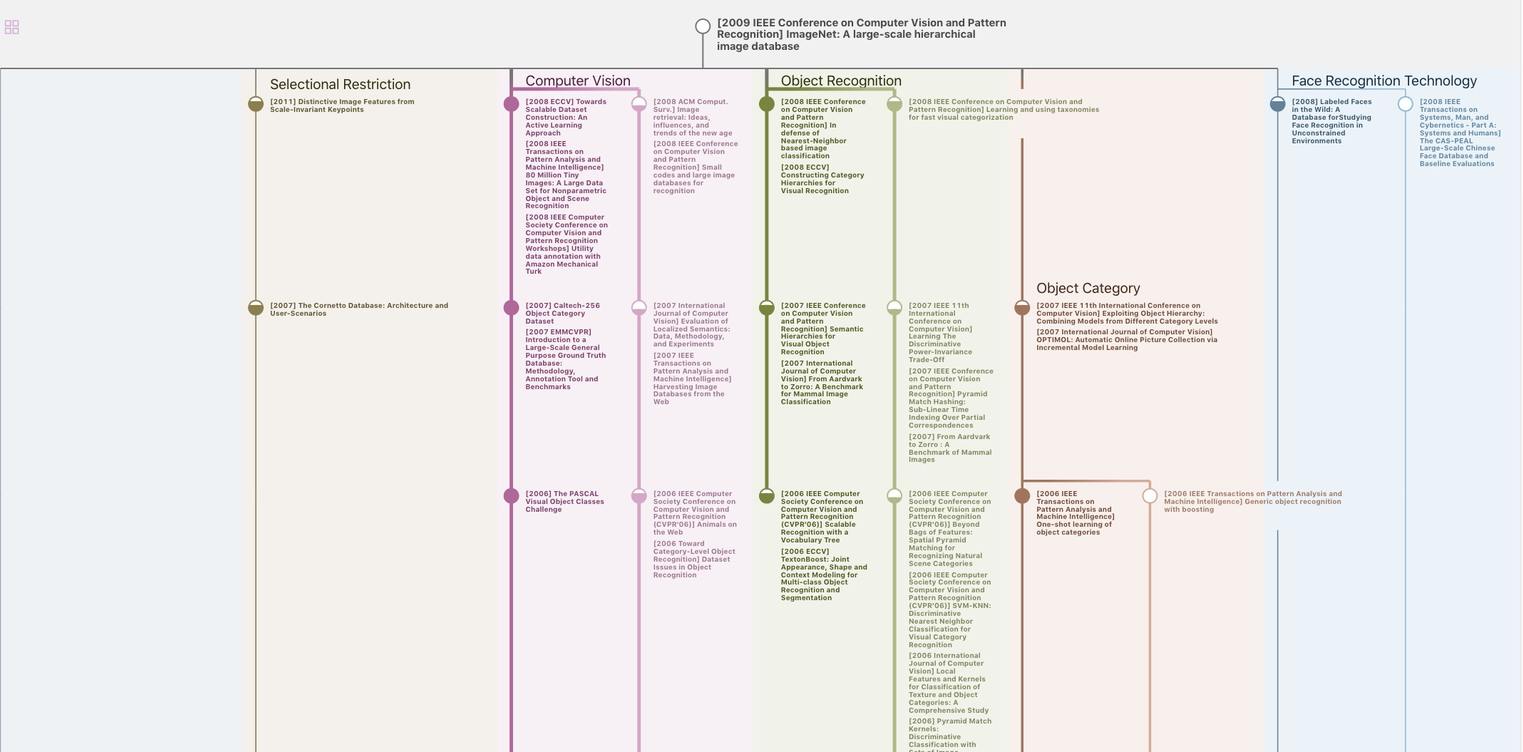
生成溯源树,研究论文发展脉络
Chat Paper
正在生成论文摘要