SQL-Encoder: Improving NL2SQL In-Context Learning Through a Context-Aware Encoder
CoRR(2024)
Abstract
Detecting structural similarity between queries is essential for selecting
examples in in-context learning models. However, assessing structural
similarity based solely on the natural language expressions of queries, without
considering SQL queries, presents a significant challenge. This paper explores
the significance of this similarity metric and proposes a model for accurately
estimating it. To achieve this, we leverage a dataset comprising 170k question
pairs, meticulously curated to train a similarity prediction model. Our
comprehensive evaluation demonstrates that the proposed model adeptly captures
the structural similarity between questions, as evidenced by improvements in
Kendall-Tau distance and precision@k metrics. Notably, our model outperforms
strong competitive embedding models from OpenAI and Cohere. Furthermore,
compared to these competitive models, our proposed encoder enhances the
downstream performance of NL2SQL models in 1-shot in-context learning scenarios
by 1-2% for GPT-3.5-turbo, 4-8% for CodeLlama-7B, and 2-3% for
CodeLlama-13B.
MoreTranslated text
AI Read Science
Must-Reading Tree
Example
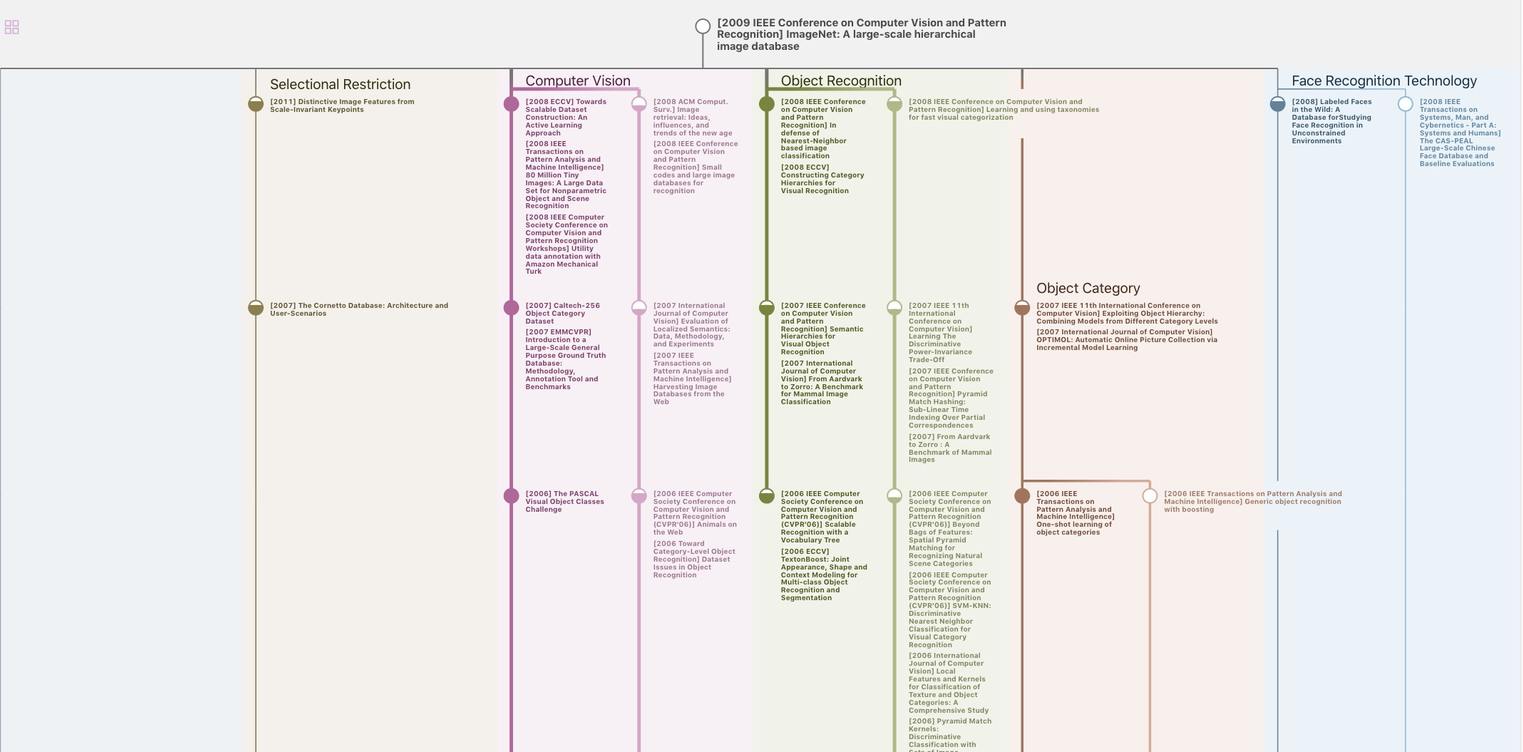
Generate MRT to find the research sequence of this paper
Chat Paper
Summary is being generated by the instructions you defined