CBGT-Net: A Neuromimetic Architecture for Robust Classification of Streaming Data
CoRR(2024)
Abstract
This paper describes CBGT-Net, a neural network model inspired by the
cortico-basal ganglia-thalamic (CBGT) circuits found in mammalian brains.
Unlike traditional neural network models, which either generate an output for
each provided input, or an output after a fixed sequence of inputs, the
CBGT-Net learns to produce an output after a sufficient criteria for evidence
is achieved from a stream of observed data. For each observation, the CBGT-Net
generates a vector that explicitly represents the amount of evidence the
observation provides for each potential decision, accumulates the evidence over
time, and generates a decision when the accumulated evidence exceeds a
pre-defined threshold. We evaluate the proposed model on two image
classification tasks, where models need to predict image categories based on a
stream of small patches extracted from the image. We show that the CBGT-Net
provides improved accuracy and robustness compared to models trained to
classify from a single patch, and models leveraging an LSTM layer to classify
from a fixed sequence length of patches.
MoreTranslated text
Key words
cortico-basal ganglia-thalamic circuit,neural network,neuro-mimicry,data stream
AI Read Science
Must-Reading Tree
Example
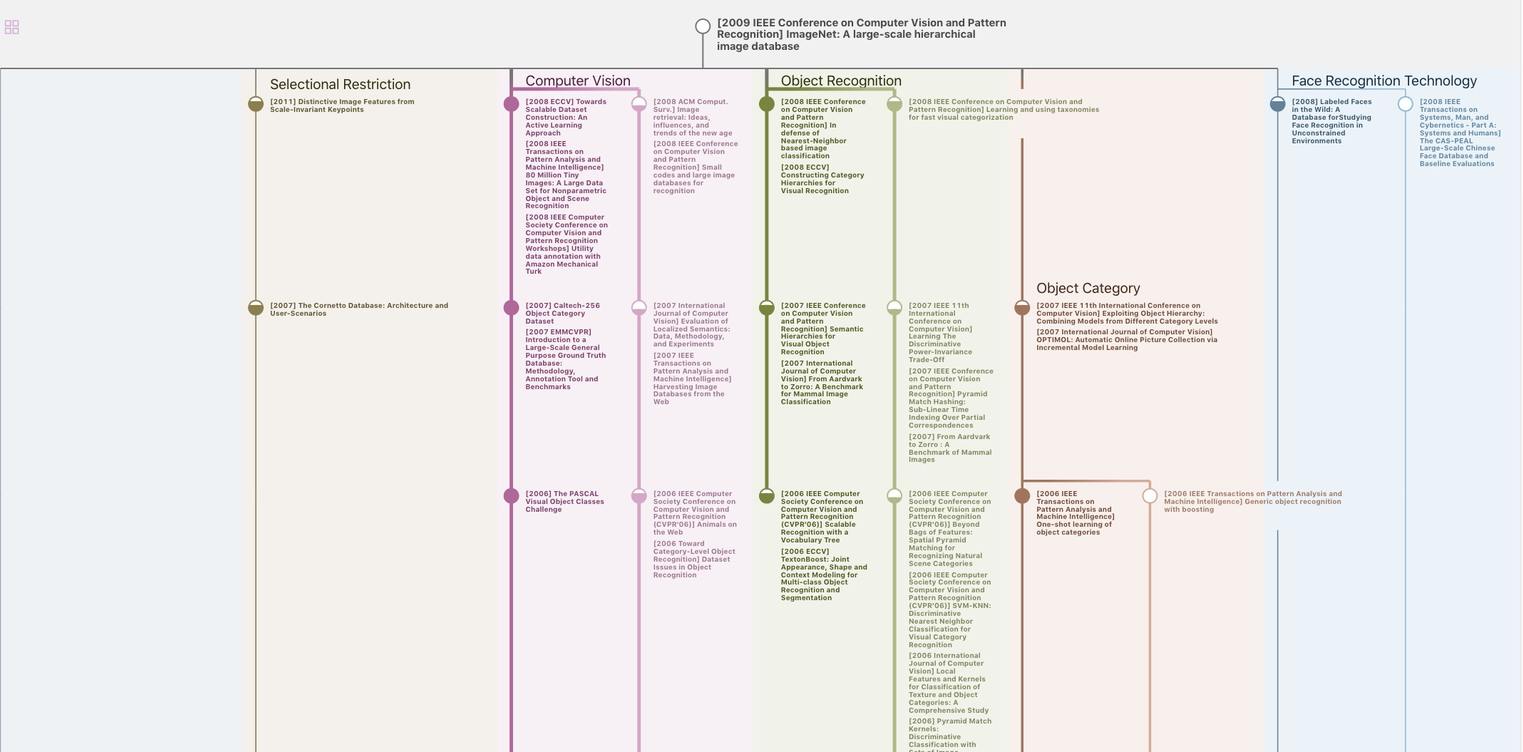
Generate MRT to find the research sequence of this paper
Chat Paper
Summary is being generated by the instructions you defined