A review of self-supervised, generative, and few-shot deep learning methods for data-limited magnetic resonance imaging segmentation
NMR in biomedicine(2024)
Abstract
Magnetic resonance imaging (MRI) is a ubiquitous medical imaging technology with applications in disease diagnostics, intervention, and treatment planning. Accurate MRI segmentation is critical for diagnosing abnormalities, monitoring diseases, and deciding on a course of treatment. With the advent of advanced deep learning frameworks, fully automated and accurate MRI segmentation is advancing. Traditional supervised deep learning techniques have advanced tremendously, reaching clinical-level accuracy in the field of segmentation. However, these algorithms still require a large amount of annotated data, which is oftentimes unavailable or impractical. One way to circumvent this issue is to utilize algorithms that exploit a limited amount of labeled data. This paper aims to review such state-of-the-art algorithms that use a limited number of annotated samples. We explain the fundamental principles of self-supervised learning, generative models, few-shot learning, and semi-supervised learning and summarize their applications in cardiac, abdomen, and brain MRI segmentation. Throughout this review, we highlight algorithms that can be employed based on the quantity of annotated data available. We also present a comprehensive list of notable publicly available MRI segmentation datasets. To conclude, we discuss possible future directions of the field-including emerging algorithms, such as contrastive language-image pretraining, and potential combinations across the methods discussed-that can further increase the efficacy of image segmentation with limited labels. This paper reviews state-of-the-art algorithms for MRI segmentation using limited annotated samples, focusing on self-supervised learning, generative models, few-shot learning, and semi-supervised learning, and discusses future directions in the field. image
MoreTranslated text
Key words
few-shot learning,GAN,MRI segmentation,self-supervised learning,semi-supervised learning
AI Read Science
Must-Reading Tree
Example
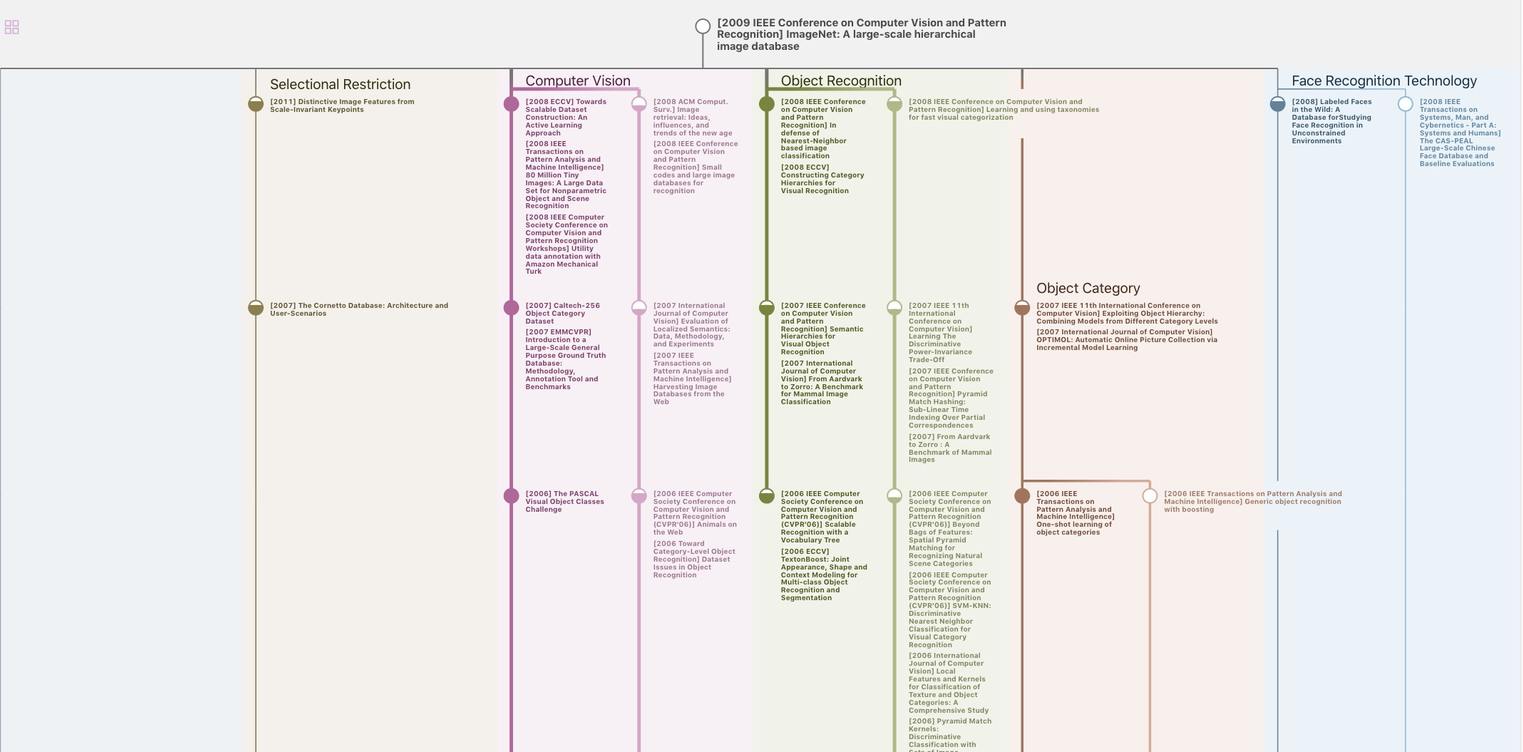
Generate MRT to find the research sequence of this paper
Chat Paper
Summary is being generated by the instructions you defined