Anchor graph-based multiview spectral clustering
Neurocomputing(2024)
摘要
Significant advances in graph-oriented clustering methods can be attributed to their effectiveness in leveraging relationships and complex structures within multiview data. However, several limitations persist in most existing graph-based multiview clustering approaches. First, quadratic or cubic complexity is required for graph construction or eigendecomposition of the Laplacian matrix in many existing methods. Second, certain methods overlook the differences between views and employ an identical indicator matrix, which can lead to over-learning in practical scenarios. Third, existing methods often neglect spatial structure and complementary information, focusing primarily on calculating error feature-by-feature using different norms. In order to tackle these drawbacks, we propose a new multiview spectral clustering model called Anchor Graph-based Multiview Spectral Clustering(AG-MSC). AG-MSC incorporates an adaptive weighting mechanism that assigns weights to each view, enhancing the robustness of the algorithm. Using a tensor Schatten p-norm constraint minimizes the discrepancy between indicator matrices obtained from different views, thereby preserving high-order information and spatial structure. To improve computational efficiency, we replace the full adjacency matrices of the corresponding views with anchor graphs. AG-MSC offers a distinct advantage over conventional spectral clustering by directly obtaining all sample categories without additional post-processing steps. We have validated the efficiency of our method through extensive experimental evaluations.
更多查看译文
关键词
Multiview learning,Tensor schatten p-norm,Spectral clustering
AI 理解论文
溯源树
样例
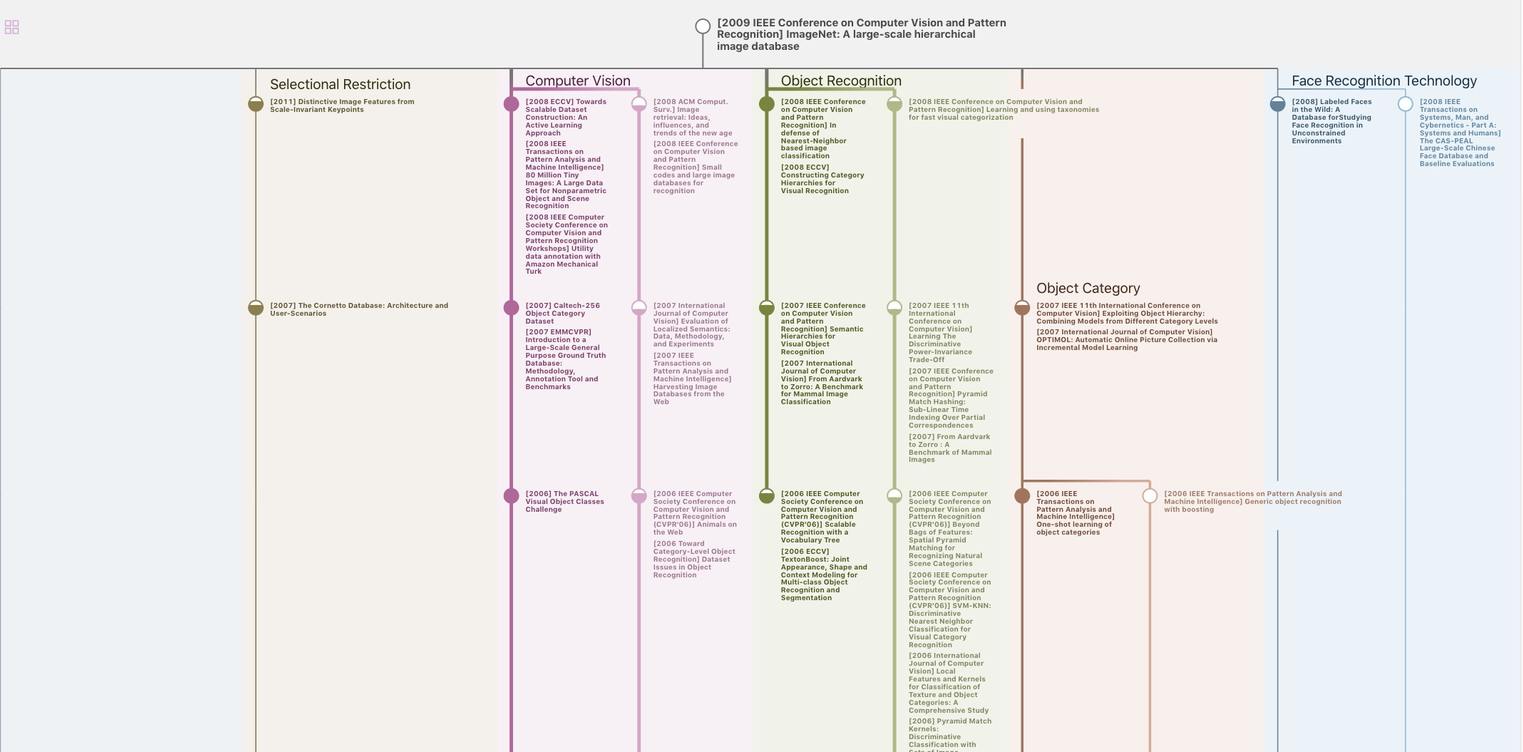
生成溯源树,研究论文发展脉络
Chat Paper
正在生成论文摘要