Single-atom catalysts property prediction via Supervised and Self-Supervised pre-training models
CHEMICAL ENGINEERING JOURNAL(2024)
Abstract
Machine learning, by virtue of its powerful predictive capabilities in discerning material properties, has emerged as an indispensable tool in the pursuit of new material discovery and design. Its specific impact on advanced catalyst design is unlocking breakthroughs across various scientific disciplines. However, the success of machine learning (ML) in predicting material properties is strongly influenced by the size of the dataset. Small datasets can lead to overfitting, resulting in suboptimal predictive performance. In this study, we compare various pretraining models that leverage expert knowledge with those based on large, mixed datasets for specific downstream property prediction tasks. We argue that transfer learning, particularly self-supervised learning based on large datasets, offers more generalizable, scalable, and accurate predictions than supervised pre-training models. Our analysis demonstrates the advantages of self-supervised pre-training, particularly in cases where expert knowledge is limited or insufficient. We evaluate the performance of these models on the challenging task of predicting the adsorption energy associated with CO 2 reduction by M -X -C single-atom catalysts. Using the optimal model, the self-supervised pre-training model, we conducted a screening of single-atom catalysts for CO 2 reduction to CO, leading to the discovery of two new, more efficient catalysts, namely Zn-NP 3 and Sn -P 4 . Our findings highlight the potential of self-supervised pre-training models in advancing material property prediction and guiding the discovery of novel materials.
MoreTranslated text
Key words
Transfer learning,Pre-training models,Supervised learning,Self-supervised learning,Single-atom catalysts
AI Read Science
Must-Reading Tree
Example
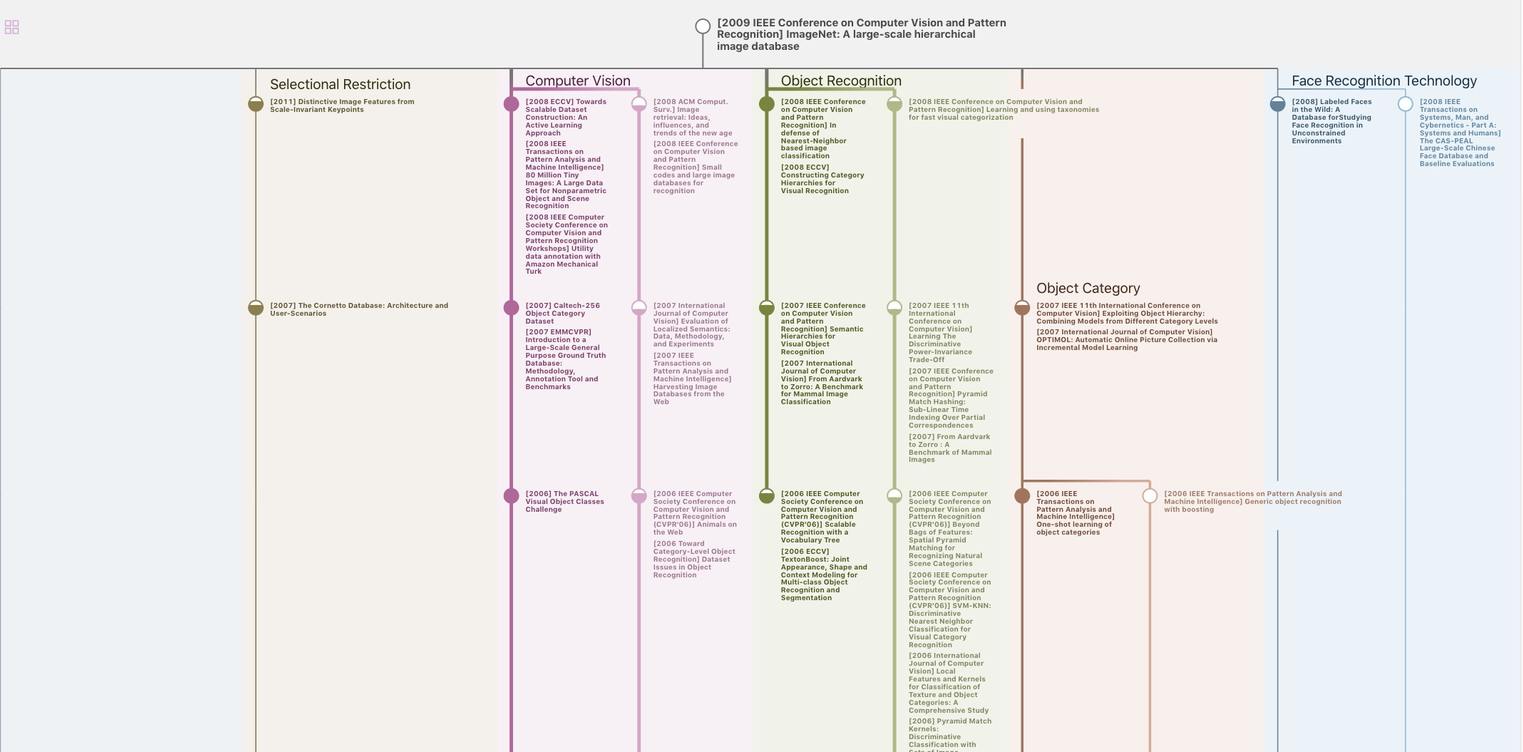
Generate MRT to find the research sequence of this paper
Chat Paper
Summary is being generated by the instructions you defined