Towards adaptive graph neural networks via solving prior-data conflicts
Frontiers of Information Technology & Electronic Engineering(2024)
Abstract
Graph neural networks (GNNs) have achieved remarkable performance in a variety of graph-related tasks. Recent evidence in the GNN community shows that such good performance can be attributed to the homophily prior; i.e., connected nodes tend to have similar features and labels. However, in heterophilic settings where the features of connected nodes may vary significantly, GNN models exhibit notable performance deterioration. In this work, we formulate this problem as prior-data conflict and propose a model called the mixture-prior graph neural network (MPGNN). First, to address the mismatch of homophily prior on heterophilic graphs, we introduce the non-informative prior, which makes no assumptions about the relationship between connected nodes and learns such relationship from the data. Second, to avoid performance degradation on homophilic graphs, we implement a soft switch to balance the effects of homophily prior and non-informative prior by learnable weights. We evaluate the performance of MPGNN on both synthetic and real-world graphs. Results show that MPGNN can effectively capture the relationship between connected nodes, while the soft switch helps select a suitable prior according to the graph characteristics. With these two designs, MPGNN outperforms state-of-the-art methods on heterophilic graphs without sacrificing performance on homophilic graphs.
MoreTranslated text
Key words
Graph neural networks,Heterophily,Prior-data conflict,图神经网络,异配性,先验数据冲突,TP18
AI Read Science
Must-Reading Tree
Example
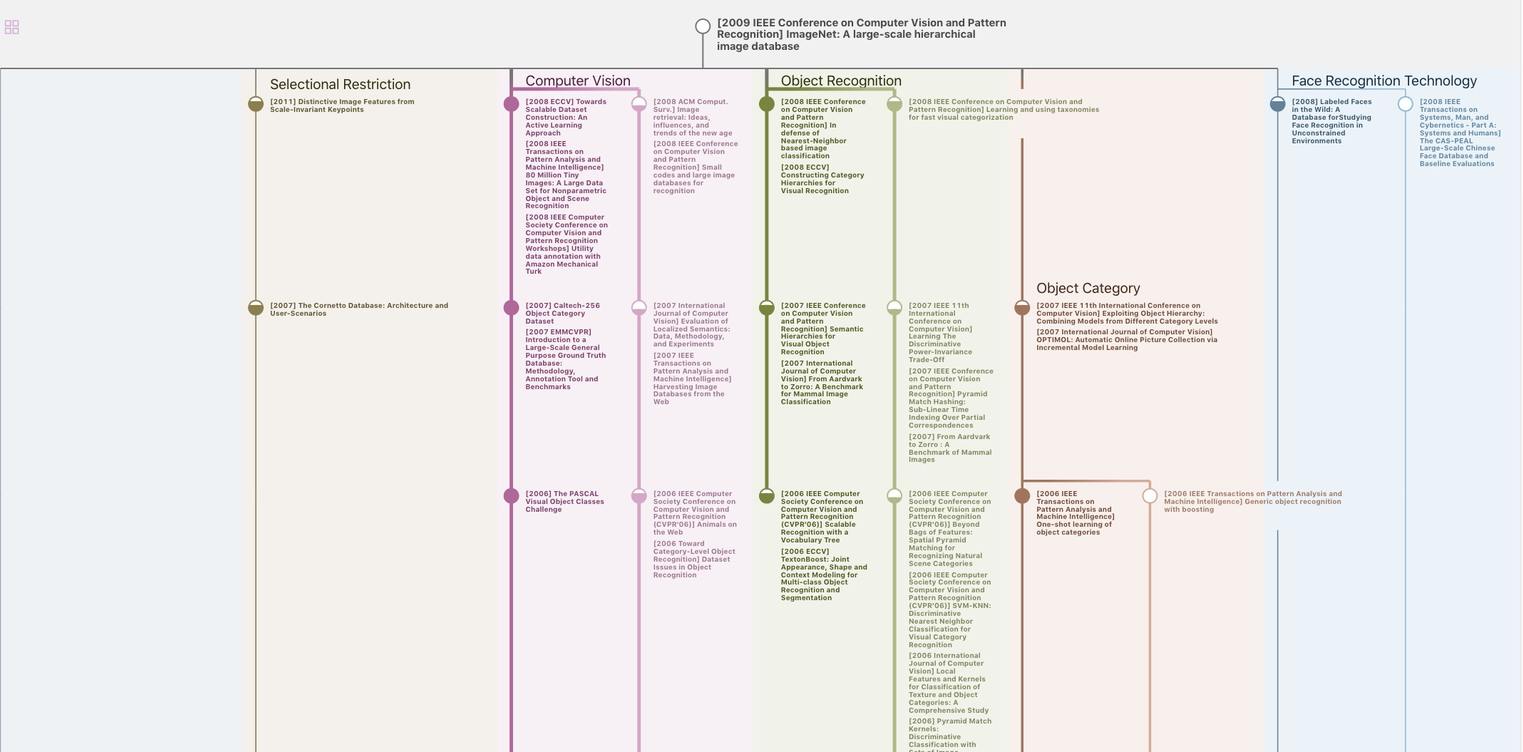
Generate MRT to find the research sequence of this paper
Chat Paper
Summary is being generated by the instructions you defined