Abstract 4951: Integrative multi-omic machine learning model predicts neoadjuvant immunotherapy response using molecular data and deep learning-derived features from digital pathology
Cancer Research(2024)
Abstract
Abstract Background: Over the past decade, there has been an exponential accumulation of digital patient data through molecular profiling and digitization of pathology slides. However, integrating this to advance diagnostics and cancer patient care remains a significant challenge. By integrating information from diverse modalities to capture aspects of the tumor microenvironment (TME) and immune landscape, we aim to improve patient stratification for immunotherapy. Methods: We collected data from baseline biopsies from patients with palpable melanoma stage III (n=195) who received neoadjuvant immunotherapy followed by surgical resection of largest lymph node metastasis. The different modalities included clinical information, whole-genome sequencing (WGS), RNAseq, laboratory tests of blood, haematoxylin and eosin (H&E) images, and PDL1 immunohistochemistry (IHC) images. Cell detection and classification models were trained to identify tumor-infiltrating lymphocytes and tumor cells on H&E whole-slide images (WSI), deriving spatial features to quantitatively describe the tumor-immune interaction. Various biomarkers, including tumor mutational burden, BRAF status, expression of inflammatory gene signatures, systemic neutrophil-to-lymphocyte ratio, and tumor-lymphocyte spatial measures, were collectively integrated using machine learning models (random forest, elastic-net regularized logistic regression, CatBoost, support vector machine (SVM), and an ensemble classifier combined from the other models) within a 5x5-fold nested cross-validation scheme to predict pathologic response. Results: Pathologic response to immunotherapy is associated with multi-omics biomarkers which were integrated using machine learning. The ensemble approach showed that when all modalities were combined a higher AUC (AUC=0.69, 0.61-0.77) could be achieved than for the clinical modality alone. RNA plus clinical emerged as the most predictive standalone modality with an AUC of 0.62 (0.50-0.75). The overall highest AUC was achieved using a SVM (AUC=0.72, 0.66-0.82). Conclusions: Immunotherapy efficacy depends on diverse tumor microenvironment components, which were comprehensively assessed through data integration. A multi-omic predictive model holds promise for refining personalized and effective immunotherapy strategies. Citation Format: Lindsay V. Leek, Vanessa Botha, Vipul A. Baxi, Jonas Teuwen, Hugo M. Horlings, Scott D. Chasalow, Joris van de Haar, Lodewyk F. Wessels, Auditi DebRoy, Emile E. Voest. Integrative multi-omic machine learning model predicts neoadjuvant immunotherapy response using molecular data and deep learning-derived features from digital pathology [abstract]. In: Proceedings of the American Association for Cancer Research Annual Meeting 2024; Part 1 (Regular Abstracts); 2024 Apr 5-10; San Diego, CA. Philadelphia (PA): AACR; Cancer Res 2024;84(6_Suppl):Abstract nr 4951.
MoreTranslated text
AI Read Science
Must-Reading Tree
Example
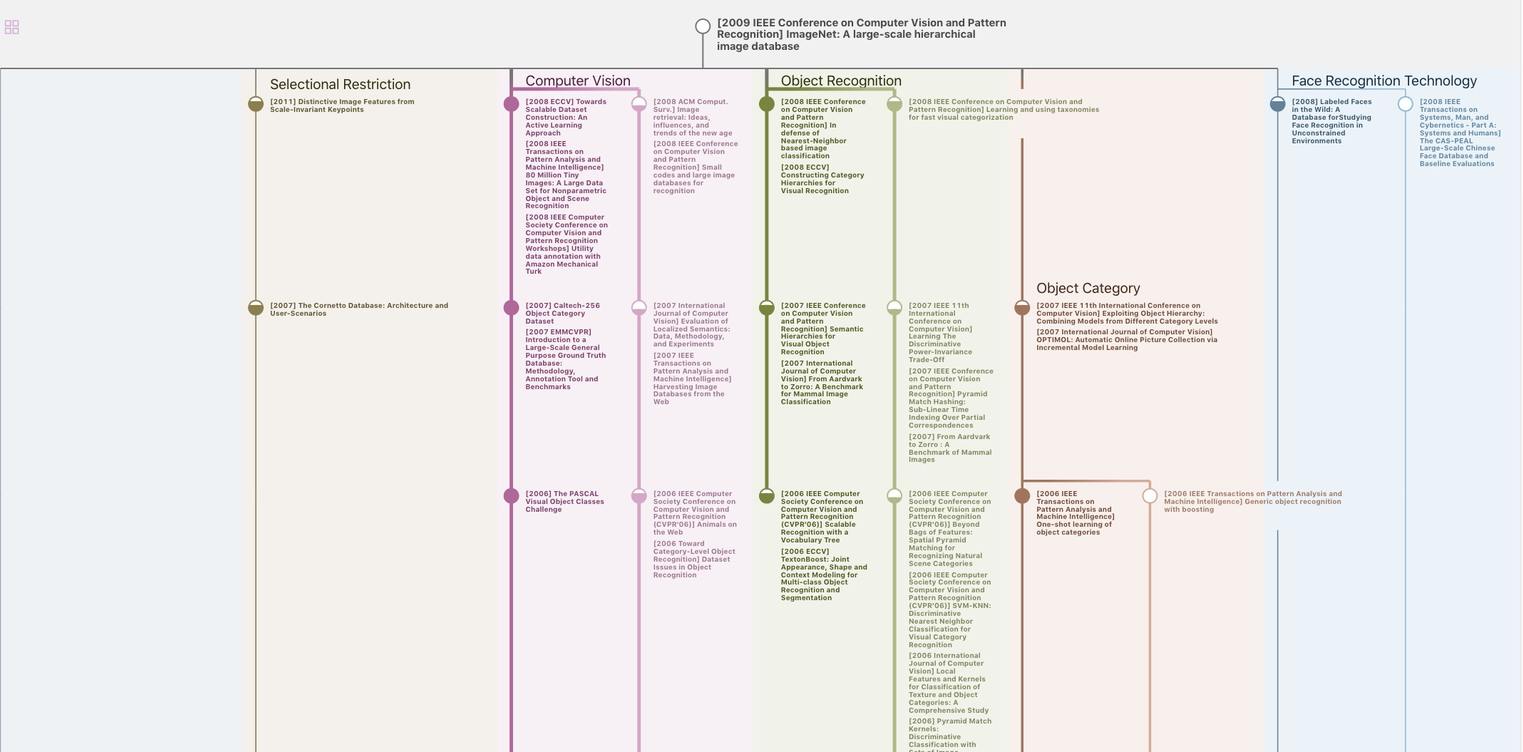
Generate MRT to find the research sequence of this paper
Chat Paper
Summary is being generated by the instructions you defined