Abstract 6619: Dynamic prediction with repeated mammogram images improves 5-year breast cancer risk performance
Cancer Research(2024)
摘要
Abstract Background: Increasing use of digital screening mammography opens potential to add individualized dynamic measures of breast image history to predict future breast cancer risk. Current prediction models use a single mammogram measure, usually breast density, at one point in time, to add to prediction models based on established risk factors. Our previous work has shown that change in each breast over time is related to risk of diagnosis with breast cancer. Methods: We apply a dynamic prediction model that includes full field digital mammogram in each breast to predict future 5-year risk. Data from Joanne Knight Breast Health Cohort (JKBHC) serve as training population with 290 pathology-confirmed breast cancer cases and 658 controls sampled with a total number of 8,712 craniocaudal (CC) view mammograms for analysis. Nurses’ Health Study 2 (NHS2) serves as the external validation with 188 cases and 462 controls sampled with a total number of 3,712 CC-views. The dynamic risk prediction model aims to estimate the probability of future breast cancer risk conditional on the past history of the longitudinal mammograms. We train the model on the JKBH cohort, retaining 20% for testing and 15% for validation. We report a time dependent AUC when evaluating model performance. Results: The mean age of women was 56.6 years at entry to cohort; 81.3% were white. Adding trajectory of mammogram images in each breast to a model improved performance. The mean number of mammograms conditional on 5 years of observation in JKBHC was 3.36 (1.49) and in NHS2 2.36 (1.53). We obtained a 5-year dynamic AUC of 0.81 (0.05) in the JKBHC internal validation and 0.77 (0.06) in the NHS2 external validation. Model performance was comparable in pre- and postmenopausal women. Because breasts with high mammographic density hinder detection of tumors we stratify analyses by dense and non-dense at baseline. Among women with dense breasts at baseline (BIRADS C and D), we observe a dynamic 5-year risk AUC = 0.77. In the subgroup with less dense breasts (BIRADS A and B) we observe AUC = 0.74. Conclusions: With this approach we can accommodate the irregularly observed time-varying images and project only partial information prior to doing the prediction. Adding trajectory of mammogram history in each breast improves 5-year breast cancer risk prediction beyond models used in clinic today. Citation Format: Graham A. Colditz, Rulla M. Tamimi, Bernard Rosner, Shu Jiang. Dynamic prediction with repeated mammogram images improves 5-year breast cancer risk performance [abstract]. In: Proceedings of the American Association for Cancer Research Annual Meeting 2024; Part 1 (Regular Abstracts); 2024 Apr 5-10; San Diego, CA. Philadelphia (PA): AACR; Cancer Res 2024;84(6_Suppl):Abstract nr 6619.
更多查看译文
AI 理解论文
溯源树
样例
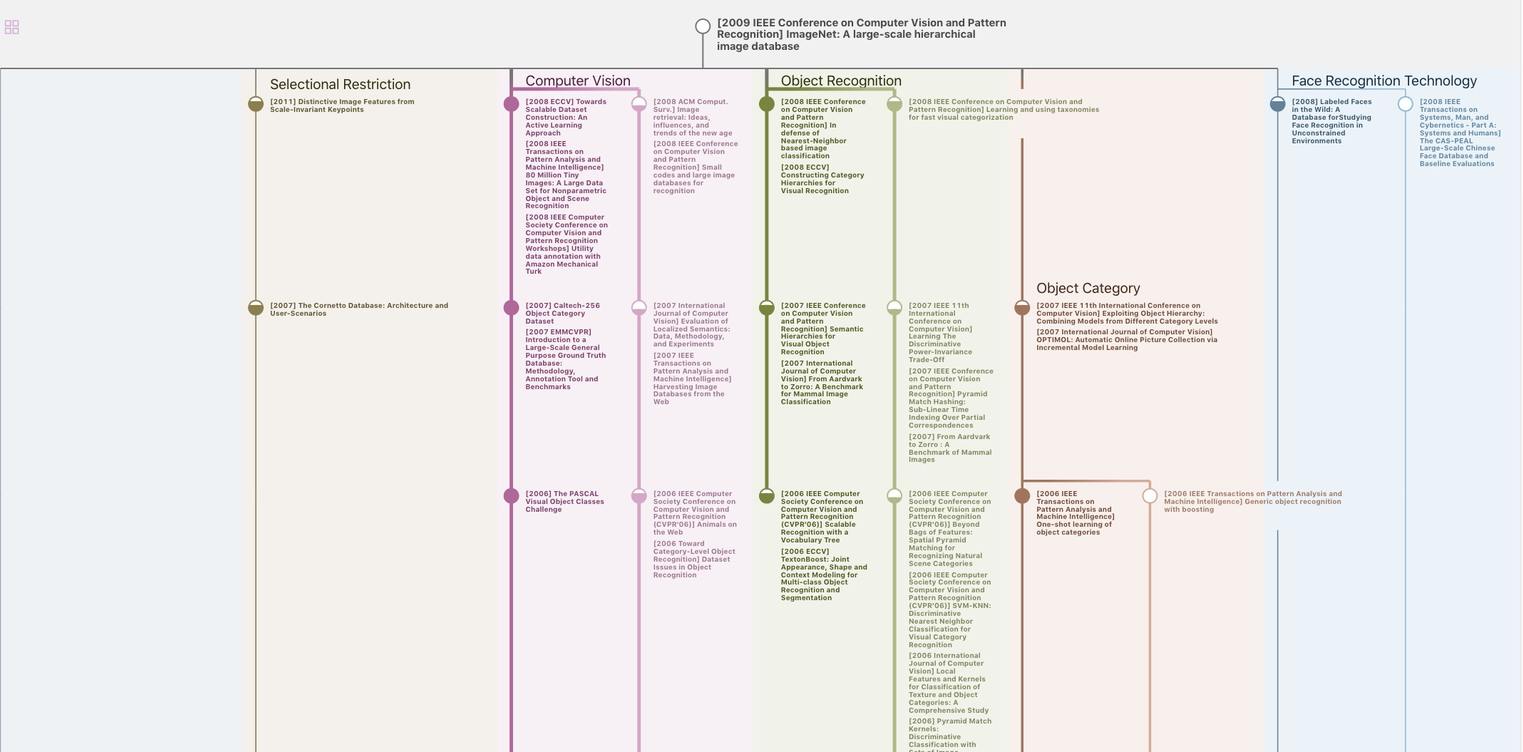
生成溯源树,研究论文发展脉络
Chat Paper
正在生成论文摘要