Abstract 4298: Predicting tumor evolution from digital histology using AI
Cancer Research(2024)
摘要
Abstract Diverse clinical presentations of clear cell renal cell carcinoma (ccRCC) confound clinical decision making, leading to over and undertreatment. Clonal evolution of ccRCC proceeds through distinct trajectories characterised by differing levels of genomic intratumoral heterogeneity (gITH) and chromosomal complexity (weighted genomic instability index, wGII). However, accurate evaluation of these indices requires multiregional profiling of fresh tumour; cost prohibitive and logistically challenging in the clinical setting. Clinical histopathology workflows routinely capture multiple tumour areas enabling the use artificial intelligence (AI) to predict tumour evolutionary features directly from clinical grade H&E whole slide image (WSIs). ccRCC displays profound genetic and histological ITH but the link between these entities remains unclear. We leverage the TRACERx Renal cohort, comprising 1485 WSIs from 81 tumours to predict WGII and gITH and to gain insights into the relationship between genetic and histological ITH. Critically, each WSI is associated with a wGII and gITH label derived from a closely linked fresh tumour sample. For both prediction tasks, we extracted meaningful features for each WSI using self-supervised representation learning “MoCo”. Since high wGII confers poor prognosis we focussed on predicting binary stratification label of high wGII or low wGII (relative to the cohort median). First we predicted wGII as a continuous variable using a supervised multiple instance learning regression model trained on the MoCo features, and then classified the predicted wGII into “high” or “low” achieving 0.80 AUROC. To predict gITH we postulated that the degree of gITH would correlate with histological ITH. Using an unsupervised clustering of refined MoCo features we defined 24 histological clusters. The number of computationally derived histological clusters within a single tumour positively correlated with gITH (pearson’s 0.56). We used the number of clusters to classify WSIs into prognostic binary groups of high or low gITH (relative to the cohort median) achieving an AUROC of 0.80. To understand the biological relationship between histological and genetic ITH we pathologically characterised the histological clusters: a pathologist annotated WSIs with tumour architecture and cytomorphology. Image tiles were associated with the annotations using spatial coordinates, illuminating phenotypic traits of different evolutionary trajectories and providing an interpretability framework for our AI pipelines. Since the tumour evolutionary course dictates disease progression tempo, applying evolutionary classification in clinic can fundamentally improve patient care. Here, for the first time, we provide a framework to translate fundamental evolutionary principles underpinning tumour biology and clinical progression into a prognostic computational pathology biomarker possible to clinically implement. Citation Format: Charlotte E. Spencer, Axel Camara, Auriane Riou, Lewis Au, Jose I. Lopez, Zayd Tippu, Charles Maussion, Kenneth Ho, Amy Strange, Emma Nye, Veronique Birault, Lydwine Van-praet, Kim Edmonds, Eleanor Carlyle, Steve Hazell, Sarah Rudman, James Larkin, Samra Turajlic. Predicting tumor evolution from digital histology using AI [abstract]. In: Proceedings of the American Association for Cancer Research Annual Meeting 2024; Part 1 (Regular Abstracts); 2024 Apr 5-10; San Diego, CA. Philadelphia (PA): AACR; Cancer Res 2024;84(6_Suppl):Abstract nr 4298.
更多查看译文
AI 理解论文
溯源树
样例
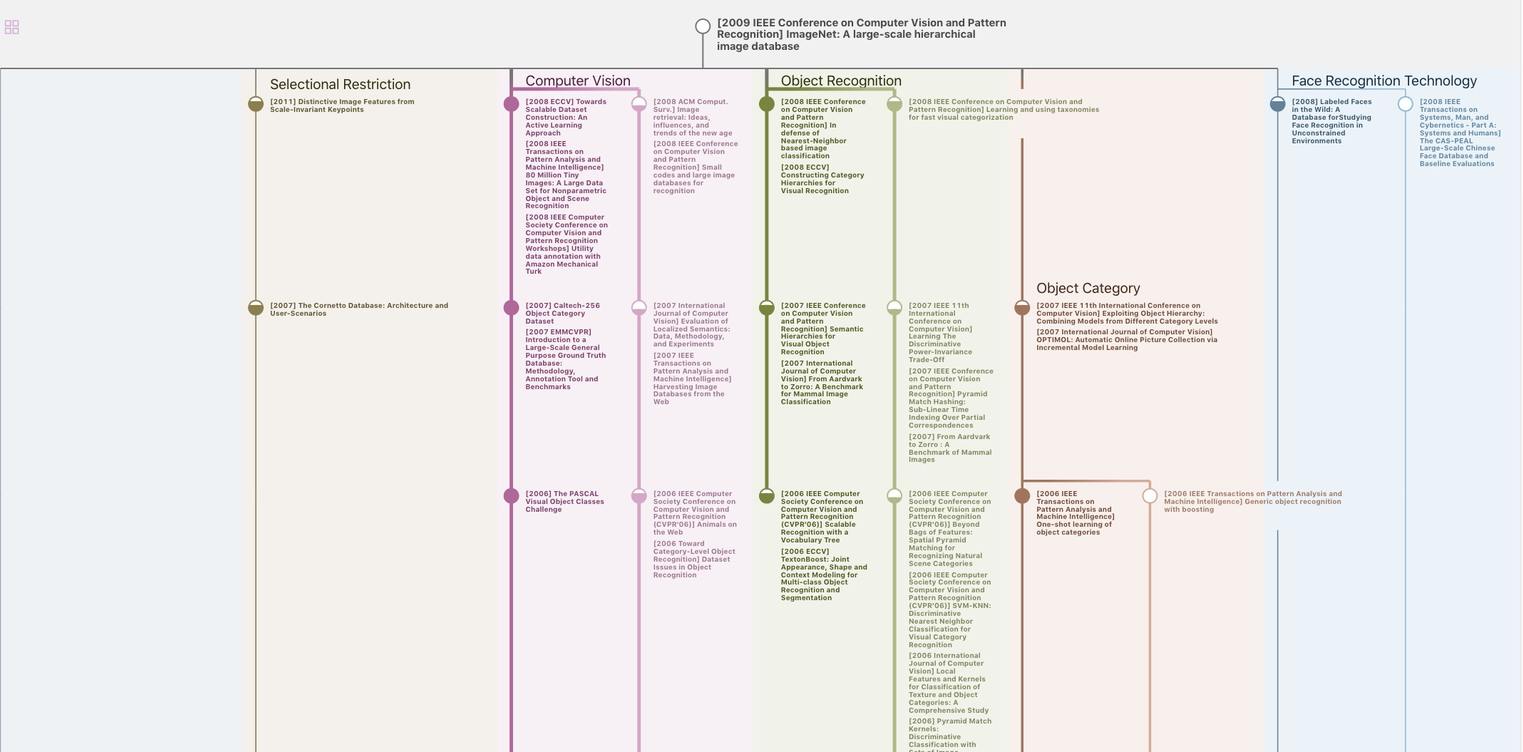
生成溯源树,研究论文发展脉络
Chat Paper
正在生成论文摘要