15 years of Adjoint Algorithmic Differentiation (AAD) in finance
QUANTITATIVE FINANCE(2024)
Abstract
Following the seminal 'Smoking Adjoint' paper by Giles and Glasserman [Smoking adjoints: Fast monte carlo greeks. Risk, 2006, 19, 88-92], the development of Adjoint Algorithmic Differentiation (AAD) has revolutionized the way risk is computed in the financial industry. In this paper, we provide a tutorial of this technique, illustrate how it is immediately applicable for Monte Carlo and Partial Differential Equations applications, the two main numerical techniques used for option pricing, and review the most significant literature in quantitative finance of the past fifteen years.
MoreTranslated text
Key words
Algorithmic differentiation,Monte Carlo simulations,Partial differential equations,Derivatives pricing,Calibration of stochastic models
AI Read Science
Must-Reading Tree
Example
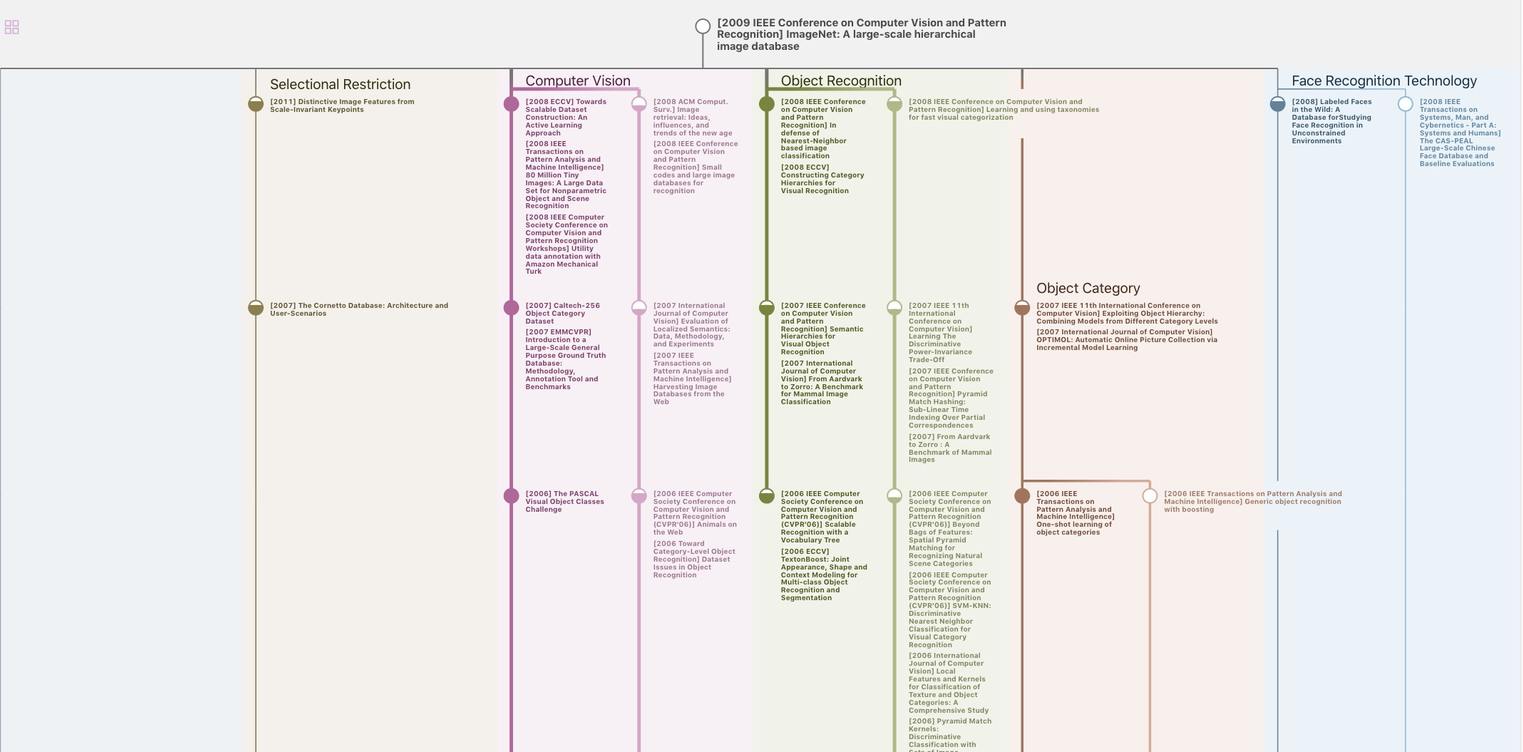
Generate MRT to find the research sequence of this paper
Chat Paper
Summary is being generated by the instructions you defined