Abstract 3516: AI-derived features of 3D glandular networks are associated with likelihood of biochemical recurrence post radical prostatectomy
Cancer Research(2024)
摘要
Abstract Introduction: Prostate cancer is the most common cancer in American men and is mainly diagnosed from two-dimensional histology sections. Recently, three-dimensional (3D) prostate histopathology has emerged as a valuable strategy to enhance the understanding of the disease by increasing microscopic sampling of specimens. This strategy enhances the microscopic examination of specimens by facilitating the analysis of the volumetric shape of cells and glands, as well as the pathway structures through entire biopsies and other morphological features. These aspects hold the potential to be linked with biochemical recurrence (BCR) outcomes. This study highlights the potential of features derived from the 3D structure of glands in samples of patients with prostate cancer for identifying patients who are at higher risk of BCR. Methods: Radical prostatectomy specimens were collected from 50 patients along with 5-year postoperative BCR follow-up from the University of Washington. A total of 118 ex vivo whole-biopsy 3D pathology images were obtained from RP by an open-top light-sheet microscopy platform. Images were converted into a synthetic CK8 immunofluorescence dataset using an image-sequence translation model. From these 3D images, a glandular structure mask was generated by a computer-vision algorithm. The 3D structure of prostate glands was modeled through a Tree-structure Extraction Algorithm for Accurate and Robust Skeletons (TEASER), which provides a simplification of the length of the gland, its shape, and its pathway. 91 features were then extracted from the glandular skeleton architecture. A 3-fold cross-validation approach was used in which patients were divided into training (D1) and test (D2) sets of 33 and 17 patients, respectively. Fisher’s score, used to select features based on their correlation to the outcome, was applied to D1 to identify the top 5 features most correlated with BCR. We then utilized these features to train a K-Nearest Neighbors classifier on D1, aimed at predicting whether a patient would experience BCR. The model’s performance was evaluated on D2 and averaged across all folds. Results: The classifier was able to accurately differentiate between BCR+ and BCR- patients, achieving an average ROCAUC of 0.73 (±0.04) in D2. Significant differences in 3D skeletal features were observed between BCR+ and BCR- groups (p<0.05). Among the five selected features, three were related to the length and diameter of the gland, with the longest glands observed in BCR- patients. The remaining two features pertained to gland curvature and tortuosity, revealing more twisted glands in BCR+ patients. Conclusion: Features derived from a skeleton model of the 3D glandular structure of biopsies showed promise in identifying patients with prostate cancer who are at a higher risk of BCR. Additional, multi-site independent validation of these findings is warranted. Citation Format: Jennifer Salguero, Hisham Abdeltawab, Kamal Hammouda, German Corredor, Sarah Hawley, Pushkar Mutha, Rohan Dhamdhere, Sebastian Medina, Tilak Pathak, Kevin Bishop, Rob Serafin, Tuomas Mirtti, Priti Lal, Lawrence True, Jonathan Liu, Anant Madabhushi. AI-derived features of 3D glandular networks are associated with likelihood of biochemical recurrence post radical prostatectomy [abstract]. In: Proceedings of the American Association for Cancer Research Annual Meeting 2024; Part 1 (Regular Abstracts); 2024 Apr 5-10; San Diego, CA. Philadelphia (PA): AACR; Cancer Res 2024;84(6_Suppl):Abstract nr 3516.
更多查看译文
AI 理解论文
溯源树
样例
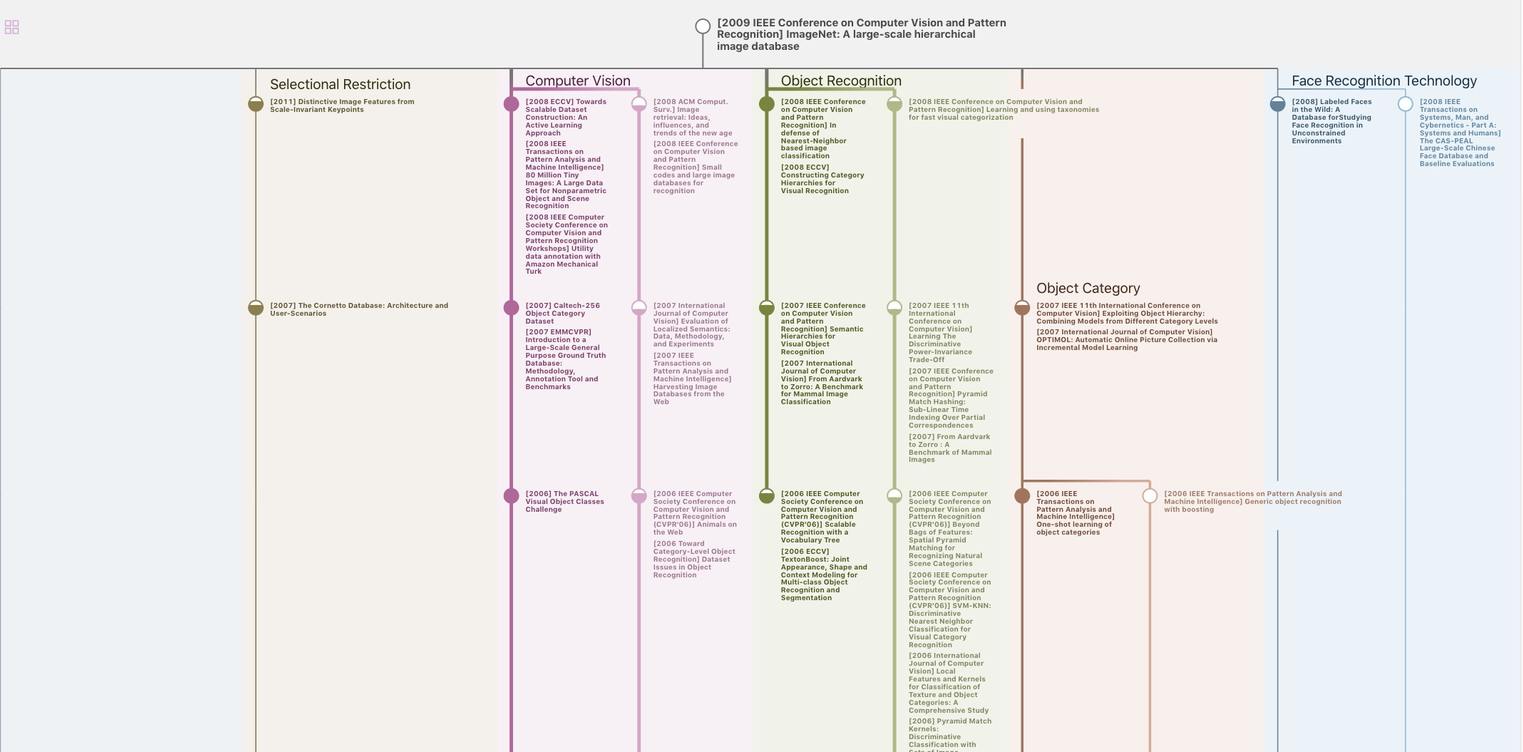
生成溯源树,研究论文发展脉络
Chat Paper
正在生成论文摘要