Abstract 7566: Identification of cancer subtypes with a ctDNA-based targeted methylation assay
Cancer Research(2024)
摘要
Abstract Introduction: Identifying cancer subtypes is necessary for cancer diagnosis, prognosis determination, and treatment selection. Furthermore, as transformation between subtypes is increasingly recognized as a key resistance mechanism to targeted therapies, serial subtype reassessment is likely to gain adoption. Cancer subtypes have traditionally been determined by pathologists based on histology; more recently, molecular subtyping has been performed using IHC, RNAseq, or assays that detect genetic alterations. However, these methods have significant limitations, including tissue biopsy requirement (IHC and RNAseq), low discriminative resolution for distinct transcriptional programs (IHC and mutation detection), and poor reproducibility and feasibility (RNAseq). The GRAIL plasma-only, ctDNA-based targeted methylation platform is a robust, biopsy-free, scalable assay that can distinguish cancer methylation patterns between different cancer signal origins through the use of a proprietary classifier. Here, we explore the GRAIL platform’s potential to detect finer-scale differences in cancer biology by developing classification algorithms for subtyping of three common cancer types. Methods: As part of the Circulating Cell-free Genome Atlas (NCT02889978) and STRIVE (NCT03085888) studies, clinical data were recorded and plasma samples were collected, accessioned, stored, and processed through GRAIL’s targeted methylation assay from 3,989 cancer and 6,013 non-cancer participants. We developed algorithms to predict cancer status and subtype for breast (triple negative breast cancer [TNBC] model; 531 cancer training participants), lung (lung histology model; 334 cancer training participants), and head and neck (HPV model; 3090 cancer training participants) cancers. Dimensionality reduction of methylation data was performed to illustrate subtype separation within these cancer types. Performance of these three algorithms was assessed on a held-out cohort of cancer samples (184 breast, 241 lung, 67 head and neck) with detectable ctDNA. Results: The lung histology model correctly classified 95% (105/111) of adenocarcinomas, 88% (68/77) of squamous cell carcinomas, and 94% (59/63) of lung neuroendocrine carcinomas and tumors. The TNBC model correctly classified 84% (58/69) of TNBCs and 82% (94/115) of non-TNBCs. The HPV model correctly identified 98% (48/49) of HPV-positive head and neck cancers, and 89% (16/18) of HPV-negative head and neck cancers. Conclusions: GRAIL’s proprietary ctDNA-based targeted methylation platform demonstrates the ability to accurately predict cancer subtypes in multiple cancers using only a plasma sample without the need for tumor biopsy. Future efforts will be directed towards generalizing the GRAIL subtyping method to additional cohorts and cancer types. Citation Format: Tracy Nance, Timothy Shaver, Yifan Zhou, Margaret Antonio, Yinjie Gao, Jonathan Heiss, Robert Calef, Joseph Hiatt, Oliver Venn, Joerg Bredno, John Beausang, Lisa Newman, Charles Swanton, Chun Zhang. Identification of cancer subtypes with a ctDNA-based targeted methylation assay [abstract]. In: Proceedings of the American Association for Cancer Research Annual Meeting 2024; Part 1 (Regular Abstracts); 2024 Apr 5-10; San Diego, CA. Philadelphia (PA): AACR; Cancer Res 2024;84(6_Suppl):Abstract nr 7566.
更多查看译文
AI 理解论文
溯源树
样例
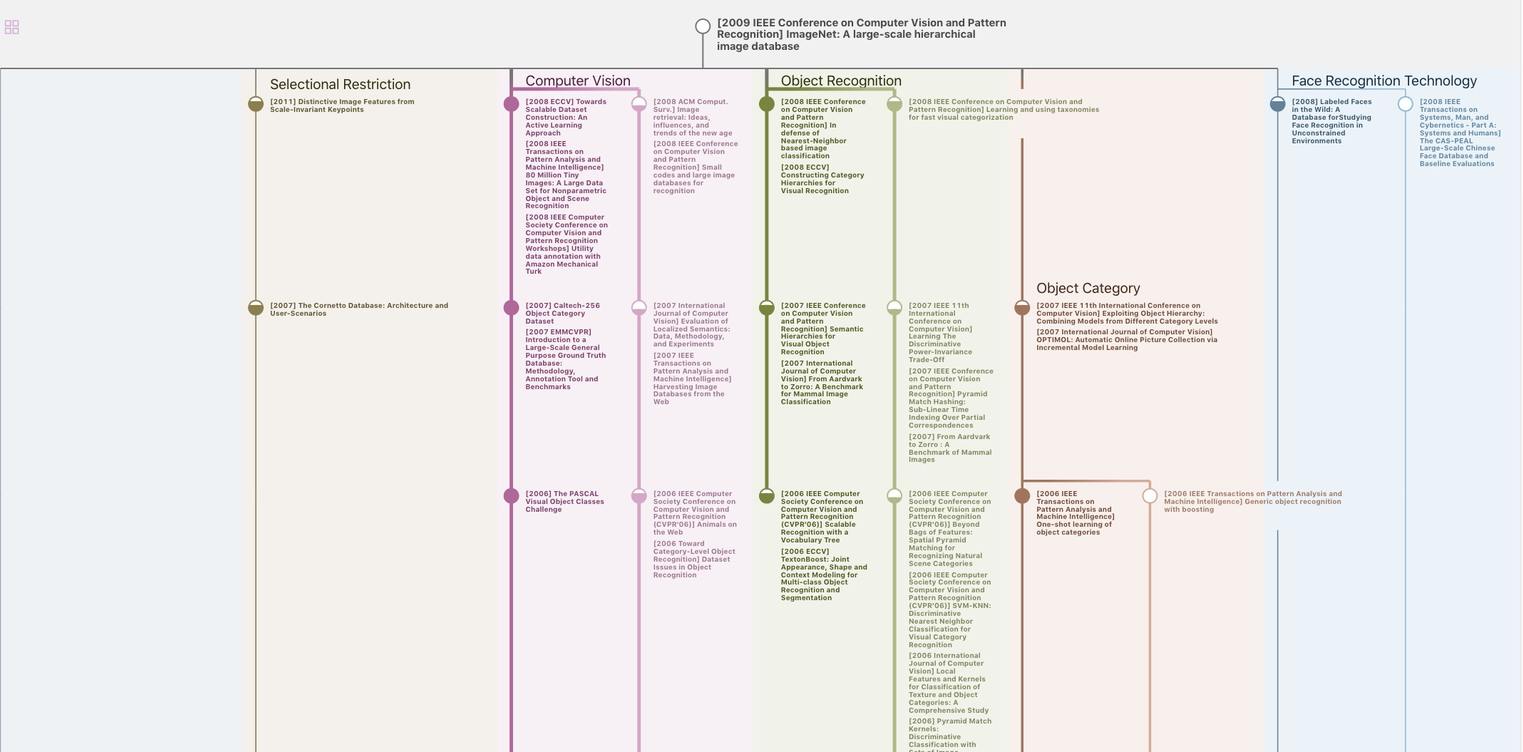
生成溯源树,研究论文发展脉络
Chat Paper
正在生成论文摘要