Effective Clustering Based Privacy Preserving Model Against Feature Attacks
Mesopotamian Journal of Computer Science(2024)
Abstract
The rise in healthcare-related illnesses has generated a substantial amount of patient data, making the safeguarding of patient data privacy imperative. Existing privacy protection methods face challenges, including longer execution times, compromised data quality, and increased information loss as data dimensions expand. Effective attribute selection is vital to enhance preservation methods. Our research introduces a privacy-preserving clustering approach that addresses these concerns through two stages: feature selection and anonymization. The first stage selects relevant features using symmetrical uncertainty (SU) and eliminates duplicates with Kendall’s Tau Correlation Coefficient. The Utility Preserved Anonymization (UPA) algorithm is employed in the second phase to achieve privacy preservation. Additionally, our approach reduces data dimensionality to simplify cluster creation for anonymization. Experimental analysis on real-time data demonstrates the strategy’s effectiveness, with outstanding sensitivity (97.85%) and accuracy (95%), efficiently eliminating unnecessary features and simplifying clustering complexity.
MoreTranslated text
AI Read Science
Must-Reading Tree
Example
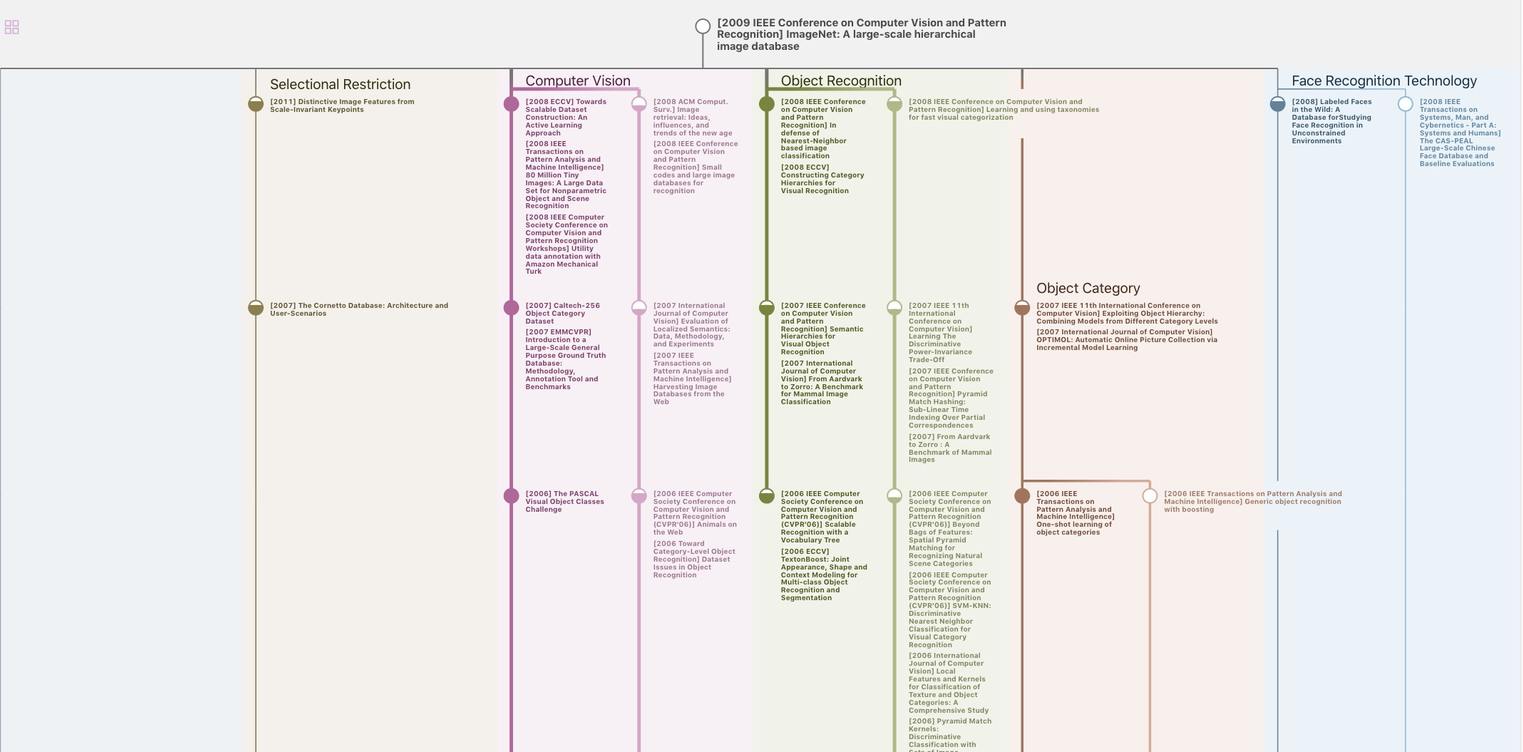
Generate MRT to find the research sequence of this paper
Chat Paper
Summary is being generated by the instructions you defined