Data-Driven Quasi-Static Surrogate Models for Nuclear-Powered Integrated Energy Systems
2024 IEEE Texas Power and Energy Conference (TPEC)(2024)
摘要
The integration of nuclear power into energy systems presents a promising avenue to address the growing global energy demands while minimizing greenhouse gas emissions. In this paper, we introduce a data-driven quasi-static surrogate model for nuclear-powered Integrated Energy Systems (IES) that comprises various components, including a small modular reactor (SMR), steam manifold, balance of plant (BOP), high-temperature steam electrolysis (HTSE), and district heating (DH) system. Traditional physics-based models for these components often entail significant computational resource and time con-sumption, necessitating the development of efficient surrogate models. The development of a complete surrogate model for the IES involves the creation of individual surrogate models for each component, leveraging machine learning techniques and simulated data. These isolated surrogate models are subsequently integrated, enabling a holistic view of the IES and reducing the computational burden associated with detailed physics-based simulations. This paper outlines the development process, validation, and the performance evaluation of the surrogate models. The exceptional performance, with low root-mean-squared errors and R-squared scores of at least 99.8% across all individual surrogate models, underscores their accuracy and practical applicability. These results demonstrate the potential of these models to expedite the analysis of nuclear-powered IES, offering insights that can shape future research and development efforts.
更多查看译文
关键词
District heating,integrated energy systems,machine learning,nuclear energy,surrogate model
AI 理解论文
溯源树
样例
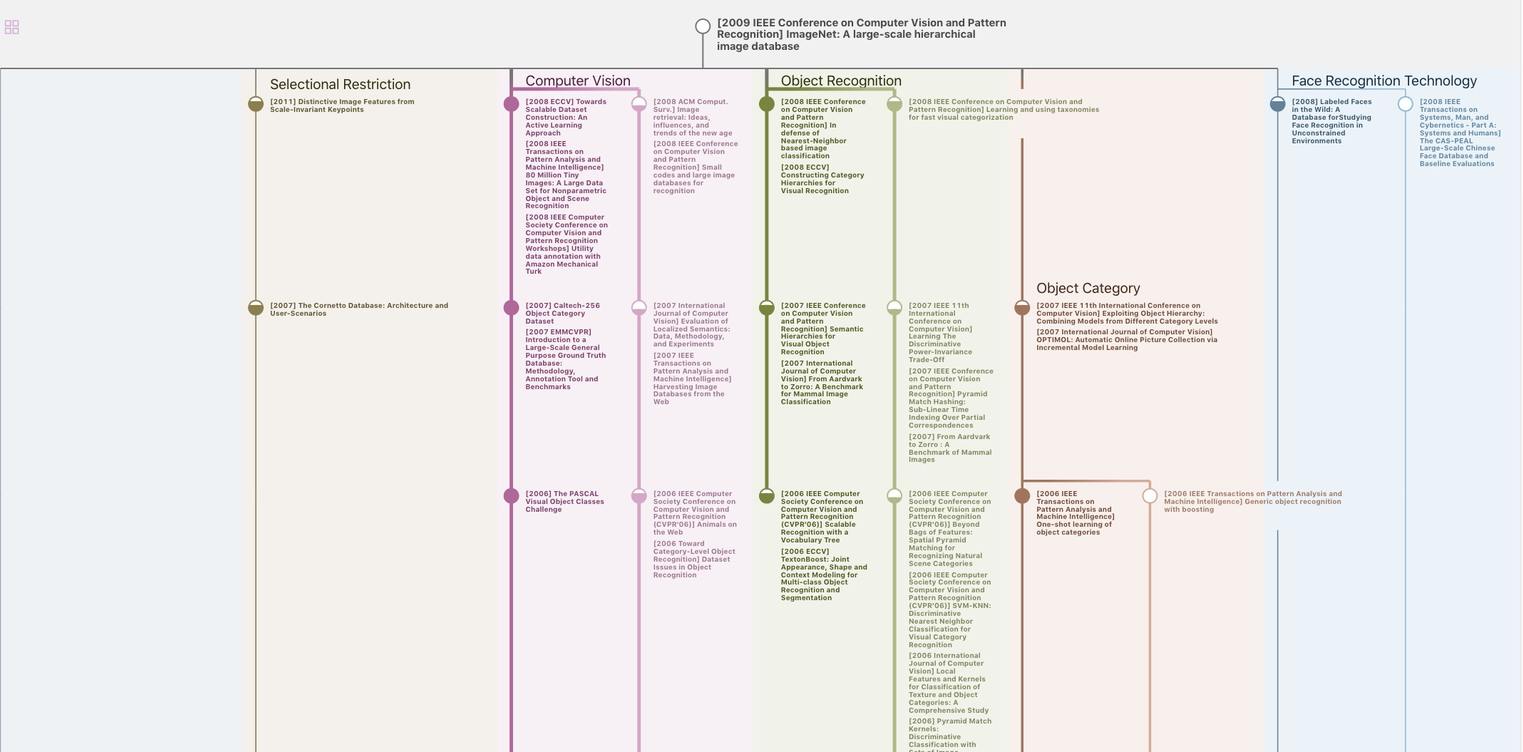
生成溯源树,研究论文发展脉络
Chat Paper
正在生成论文摘要