Abstract 4922: Unraveling sarcomatoid features in clear cell renal cell carcinoma with RNA-seq
Cancer Research(2024)
摘要
Abstract Introduction: Sarcomatoid сlear cell renal cell carcinoma (ccRCC) represents a challenging and aggressive subset of kidney cancers with poor prognosis and resistance to conventional treatments. Recent studies have shown the benefit of checkpoint inhibitors (CPIs) for patients with sarcomatoid components; therefore, precise and objective evaluation of sarcomatoid differentiation is essential for CPI selection. Here, we developed a predictive model to identify sarcomatoid features in ccRCC. Methods: The machine learning-based transcriptomic sccRCC model was developed on 512 samples from publicly available datasets: TCGA-KIRC and CPTAC-ccRCC. Samples were excluded with the following criteria: inconsistent with ccRCC, less than 10% sarcomatoid component, or <20% tumor neoplastic cellularity. Sarcomatoid features for each case were assessed by 3 pathologists. The meta-cohort was divided into training (n = 348) and validation (n = 164) cohorts.The model was tested on a publicly available cohort of ccRCC patients from Sato et al. (n = 100). Clinical validation was performed using an additional prospective cohort that received BostonGene’s Tumor PortraitTM test (n = 32) with RNA-seq and whole exome sequencing (WES). The JAVELIN Renal 101 cohort was used to uncover differences in progression-free survival (PFS) associated with a predicted sarcomatoid component in advanced or metastatic ccRCCs treated with a combination of CPIs and tyrosine kinase inhibitors (TKIs). Results: H&E analysis of the TCGA, CPTAC, and BostonGene cohorts identified zones with sarcomatoid features in 129 out of 556 (23.2%) samples. The sarcomatoid predictive model demonstrated robust performance, with weighted F1-scores of 0.85 when applied to the test cohort (Sato et al.) and 0.70 for the prospective clinical cohort. The model accurately classified 9 out of 10 total sarcomatoid samples in the prospective clinical cohort, highlighting its potential for real-world clinical applications. When applied to TCGA validation samples, sarcomatoid patients identified by the transcriptomic model exhibited median overall survival (mOS) of 55 months, whereas sarcomatoid patients based on H&E assessment had a mOS of 67 months, indicative of the model’s superior performance when detecting sarcomatoid features for OS prediction. Moreover, the sarcomatoid ccRCCs predicted by transcriptomic model that were treated with a combination of CPIs and TKIs had statistically (p=0.0001) improved PFS compared to the TKI-treated group. Conclusion: The developed transcriptomic model effectively differentiated sarcomatoid from non-sarcomatoid ccRCC samples with robust performance metrics. With additional investigation, the predictive value of the sarcomatoid ccRCC transcriptomic model can be leveraged to guide CPI and TKI selection. Citation Format: Kirill Kryukov, Suren Davitavyan, Danil Stupichev, Anna Sharun, Anna Love, Mikhail Kleimenov, Stepan Kuznetsov, Arina Tkachuk, Vladimir Kushnarev. Unraveling sarcomatoid features in clear cell renal cell carcinoma with RNA-seq [abstract]. In: Proceedings of the American Association for Cancer Research Annual Meeting 2024; Part 1 (Regular Abstracts); 2024 Apr 5-10; San Diego, CA. Philadelphia (PA): AACR; Cancer Res 2024;84(6_Suppl):Abstract nr 4922.
更多查看译文
AI 理解论文
溯源树
样例
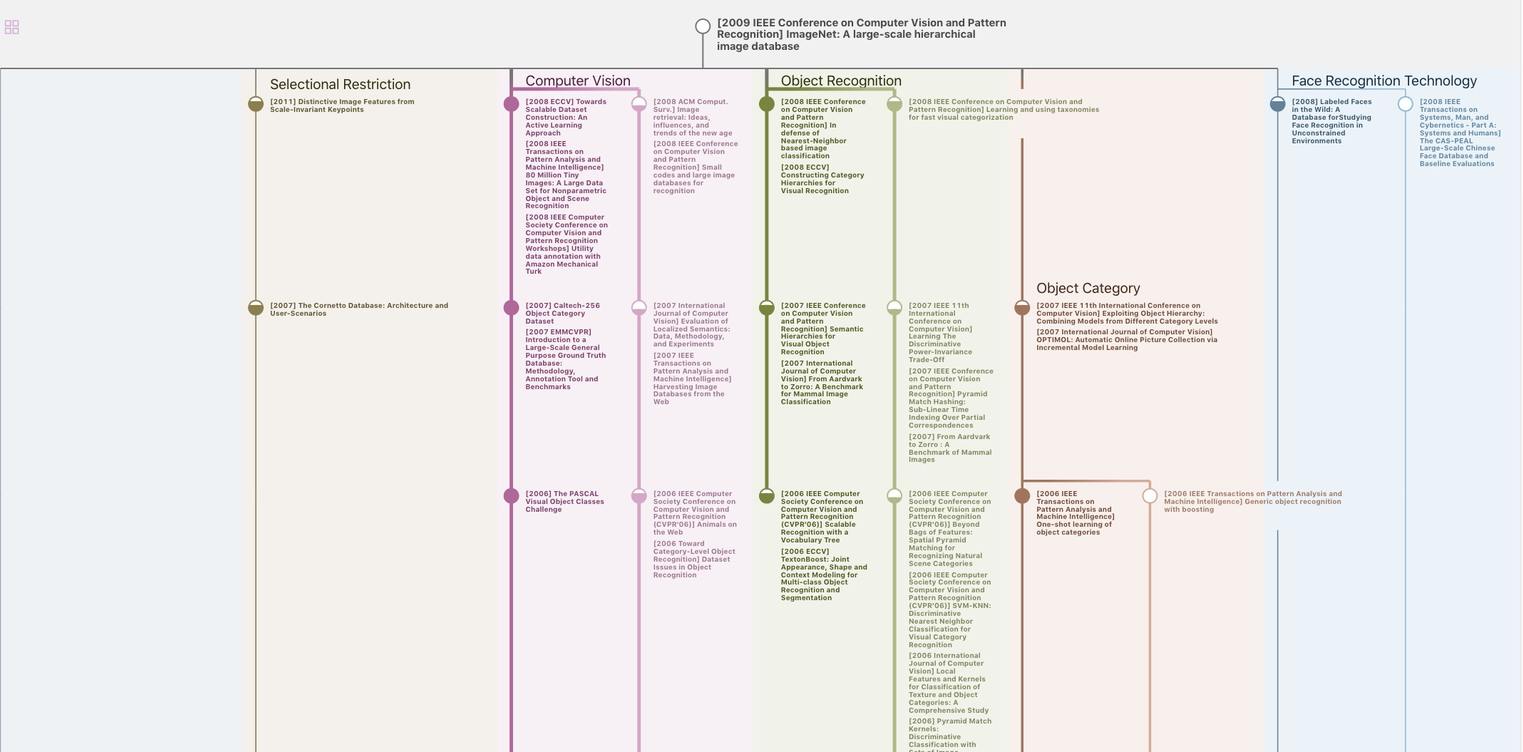
生成溯源树,研究论文发展脉络
Chat Paper
正在生成论文摘要