Deep Attention GRU-GRBM with Dropout for Fault Location in Power Distribution Networks
2024 IEEE Texas Power and Energy Conference (TPEC)(2024)
摘要
Effective fault location algorithms contribute to reducing the recovery and restoration time and improve the resilience of the power distribution networks. The existing machine learning-based approaches for fault location exhibit limitations, notably the absence of unsupervised feature learning, disregarding the capture of semantic features, and overlooking task-relevant features. This paper introduces the deep-attention Gated Recurrent Unit Gaussian Restricted Boltzmann Machine (GRU-GRBM) framework for fault location and classification. It combines an attention-enhanced GRU for accurate task-relevant temporal feature extraction, a GRBM-based autoencoder for unsupervised generative feature learning, and a sparse deep Rectified Linear Unit (ReLU) network with a mutual information (MI)-based dropout technique for supervised estimation of fault location and class. The proposed structure is shown to outperform the state-of-the-art methods on the IEEE 123-bus system through generative feature extraction, attention mechanisms, and feature sparseness.
更多查看译文
关键词
Fault Classification,Fault Location,Power Distribution Networks
AI 理解论文
溯源树
样例
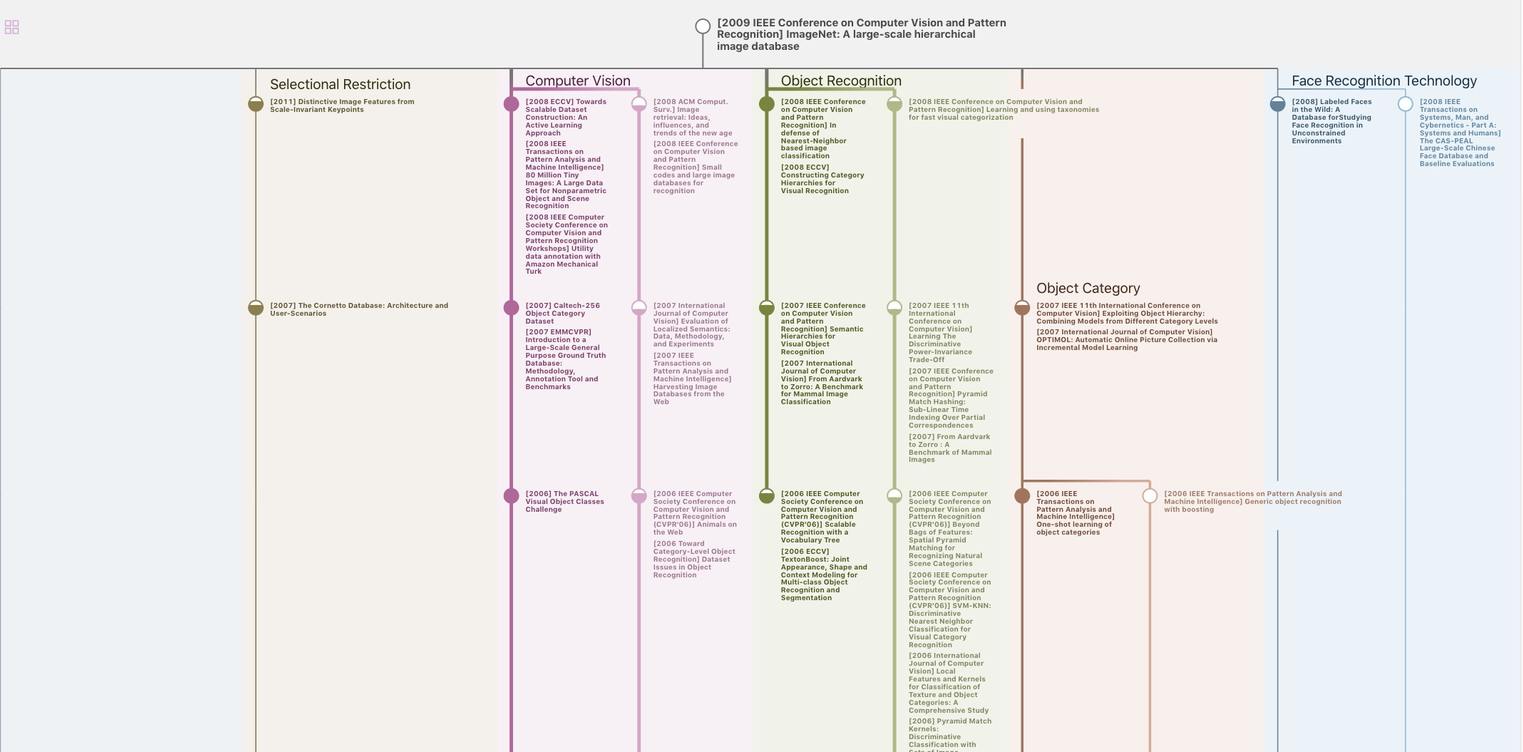
生成溯源树,研究论文发展脉络
Chat Paper
正在生成论文摘要