Abstract 899: Development of a deep learning model for cell type mapping in colorectal cancer using H&E images leveraging image-based spatial transcriptomics data
Cancer Research(2024)
摘要
Abstract Purpose The tumor microenvironment (TME) is crucial in colorectal cancer as it influences disease progression, treatment response, and patient outcomes, providing valuable insights for personalized therapies and prognostic assessments. Here, we have developed a deep learning model by integrating hematoxylin and eosin (H&E) stained images of colorectal cancer and image-based spatial transcriptomics (Xenium) to infer spatial mapping of cell types in TME only using H&E images. Methods A total of 30 H&E images of colorectal cancer obtained by tissue microarray were registered with image-based spatial transcriptomics data (Xenium). Utilizing a Variational Autoencoder (VAE) based model and leveraging reference single-cell data enables the acquisition of cell types for individual cells in image-based spatial transcriptomics. A convolutional neural network (CNN) model was developed using H&E image as inputs to predict cell types in H&E-stained tissue image patches of colorectal cancer collected from various patients. The model also estimated the cell types from H&E-stained whole slide tissue image of colorectal cancer of The Cancer Genome Atlas (TCGA-COAD). Results The accuracy of the model's predictions for cell types using H&E image patches was notably high and exhibited a significant concordance with the results obtained through the validation. The Intersection over Union (IoU) metric for image segmentation indicated a value of 0.66 for epithelial cells and 0.44 for TNK cells. The output of deep learning model for epithelial cells and T/NK cells from TCGA-COAD tissue images showed a correlation with human-labeled regions of cancer epithelium and immune cells. Conclusions Leveraging image-based spatial transcriptomics, we developed a deep learning model capable of discerning various cell types within the tumor microenvironment solely from H&E images. This clinically translatable approach is valuable for investigating tumor microenvironment to develop biomarkers associated with various cancer therapeutics particularly immuno-oncology drugs. This approach can yield objective deep learning-based models without human labels for characterizing the tumor microenvironment in single-cell resolution, particularly regarding spatial immune distribution. Citation Format: Seungho Cook, Dongjoo Lee, Myunghyun Lim, Jae Eun Lee, Daeseung Lee, Hyung-Jun Im, Jung-Soo Pyo, Kwon Joong Na, Hongyoon Choi. Development of a deep learning model for cell type mapping in colorectal cancer using H&E images leveraging image-based spatial transcriptomics data [abstract]. In: Proceedings of the American Association for Cancer Research Annual Meeting 2024; Part 1 (Regular Abstracts); 2024 Apr 5-10; San Diego, CA. Philadelphia (PA): AACR; Cancer Res 2024;84(6_Suppl):Abstract nr 899.
更多查看译文
AI 理解论文
溯源树
样例
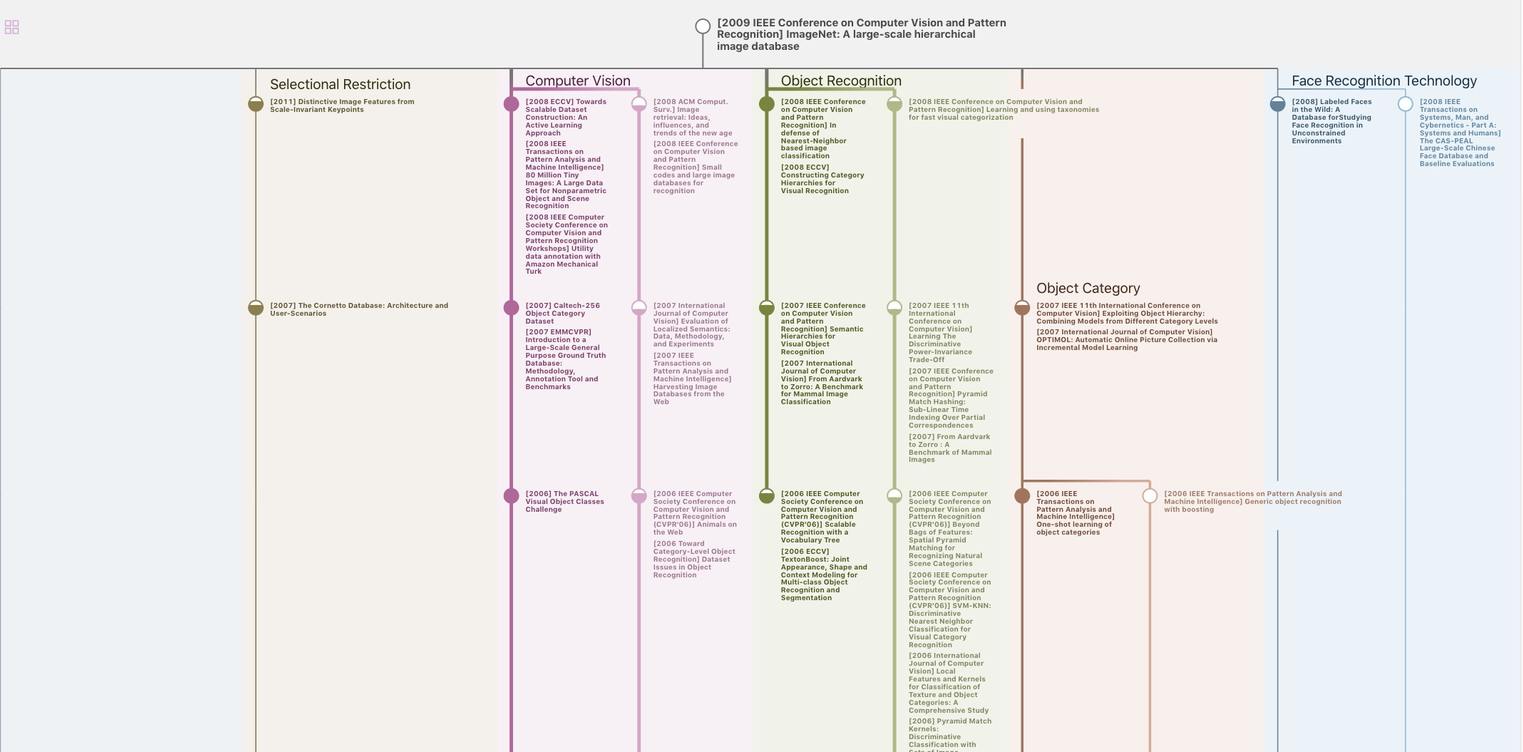
生成溯源树,研究论文发展脉络
Chat Paper
正在生成论文摘要