SALSA: Salience-Based Switching Attack for Adversarial Perturbations in Fake News Detection Models.
ECIR (5)(2024)
摘要
Despite advances in fake news detection algorithms, recent research reveals that machine learning-based fake news detection models are still vulnerable to carefully crafted adversarial attacks. In this landscape, traditional methods, often relying on text perturbations or heuristic-based approaches, have proven insufficient, revealing a critical need for more nuanced and context-aware strategies to enhance the robustness of fake news detection. Our research identifies and addresses three critical areas: creating subtle perturbations, preserving core information while modifying sentence structure, and incorporating inherent interpretability. We propose SALSA, an adversarial Sal ience-based S witching A ttack strategy that harnesses salient words, using similarity-based switching to address the shortcomings of traditional adversarial attack methods. Using SALSA, we perform a two-way attack: misclassifying real news as fake and fake news as real. Due to the absence of standardized metrics to evaluate adversarial attacks in fake news detection, we further propose three new evaluation metrics to gauge the attack’s success. Finally, we validate the transferability of our proposed attack strategy across attacker and victim models, demonstrating our approach’s broad applicability and potency. Code and data are available here at https://github.com/iamshnoo/salsa .
更多查看译文
AI 理解论文
溯源树
样例
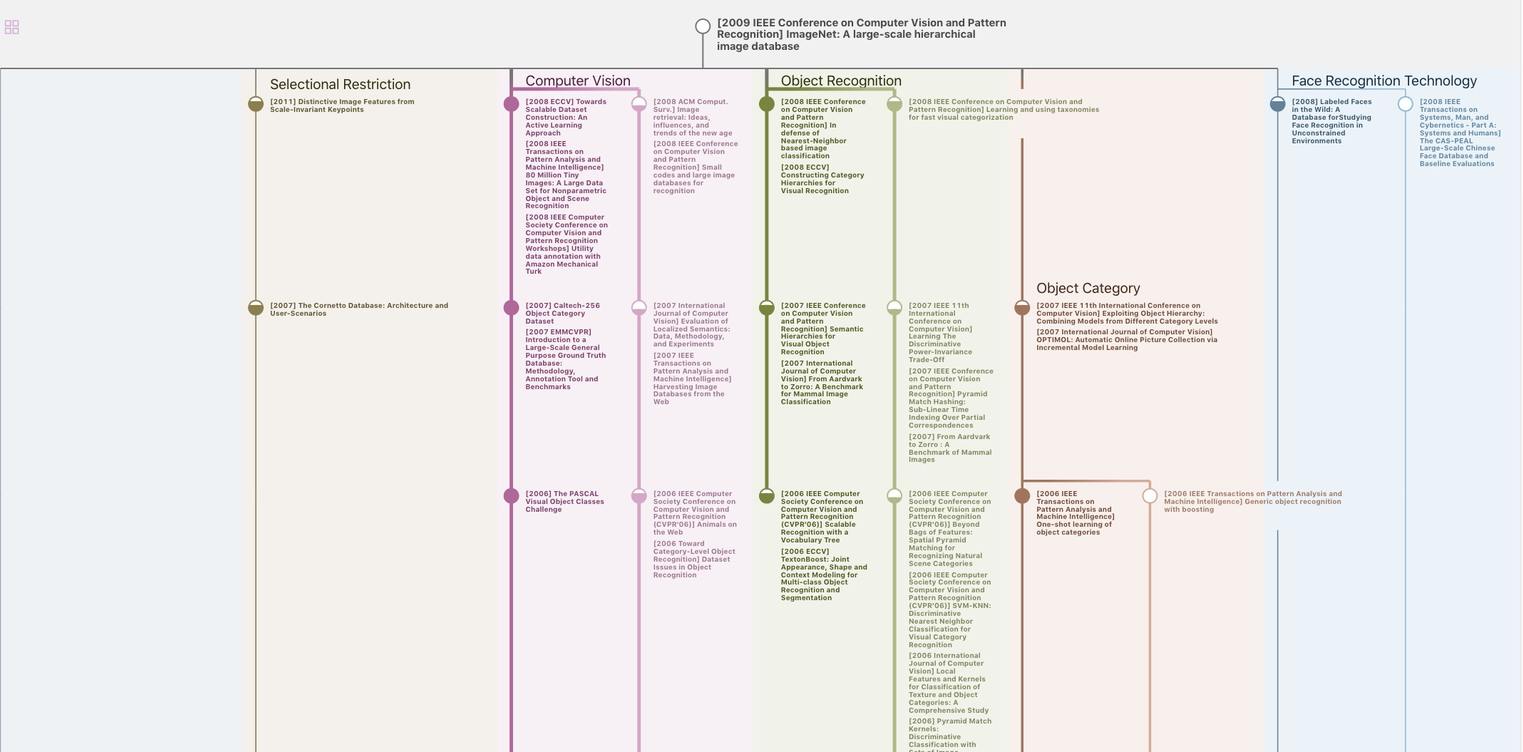
生成溯源树,研究论文发展脉络
Chat Paper
正在生成论文摘要