Semanformer: Semantics-aware Embedding Dimensionality Reduction Using Transformer-Based Models.
IEEE International Conference on Semantic Computing(2024)
摘要
In recent years, transformer-based models, particularly BERT (Bidirectional encoder Representations from Transformers), have revolutionized natural language processing tasks, achieving state-of-the-art performance in various domains. In the context of natural language processing (NLP) and linguistics, understanding the semantic aspects of text is crucial for tasks like information retrieval, sentiment analysis, machine translation, and many others. However, the high dimensionality of BERT embeddings presents challenges in real-world applications due to increased memory and computational requirements. Reducing the dimensionality of BERT embeddings would benefit many application downstream tasks by reducing the computational requirements. Although there are prevalently used dimensionality reduction methods which focus on feature representation with lower dimensions, their application on NLP tasks may not yield semantically correct results. We propose a novel framework named as semanformer (semantics-aware encoder-decoder dimensionality reduction method) that leverages transformer-based encoder-decoder model architecture to perform dimensionality reduction on BERT embeddings for a corpus while preserving crucial semantic information. To evaluate the effectiveness of our approach, we conduct a comprehensive use case evaluation on diverse text datasets by sentence reconstruction. Our experiments show that our proposed method achieves high sentence reconstruction accuracy (SRA) more than 83% compared to the traditional dimensionality reduction methods such as PCA (SRA < 66%) and t-SNE (SRA < 9%).
更多查看译文
关键词
BERT,Transformer,Dimensionality reduction,Sentence reconstruction,Embedding reconstruction
AI 理解论文
溯源树
样例
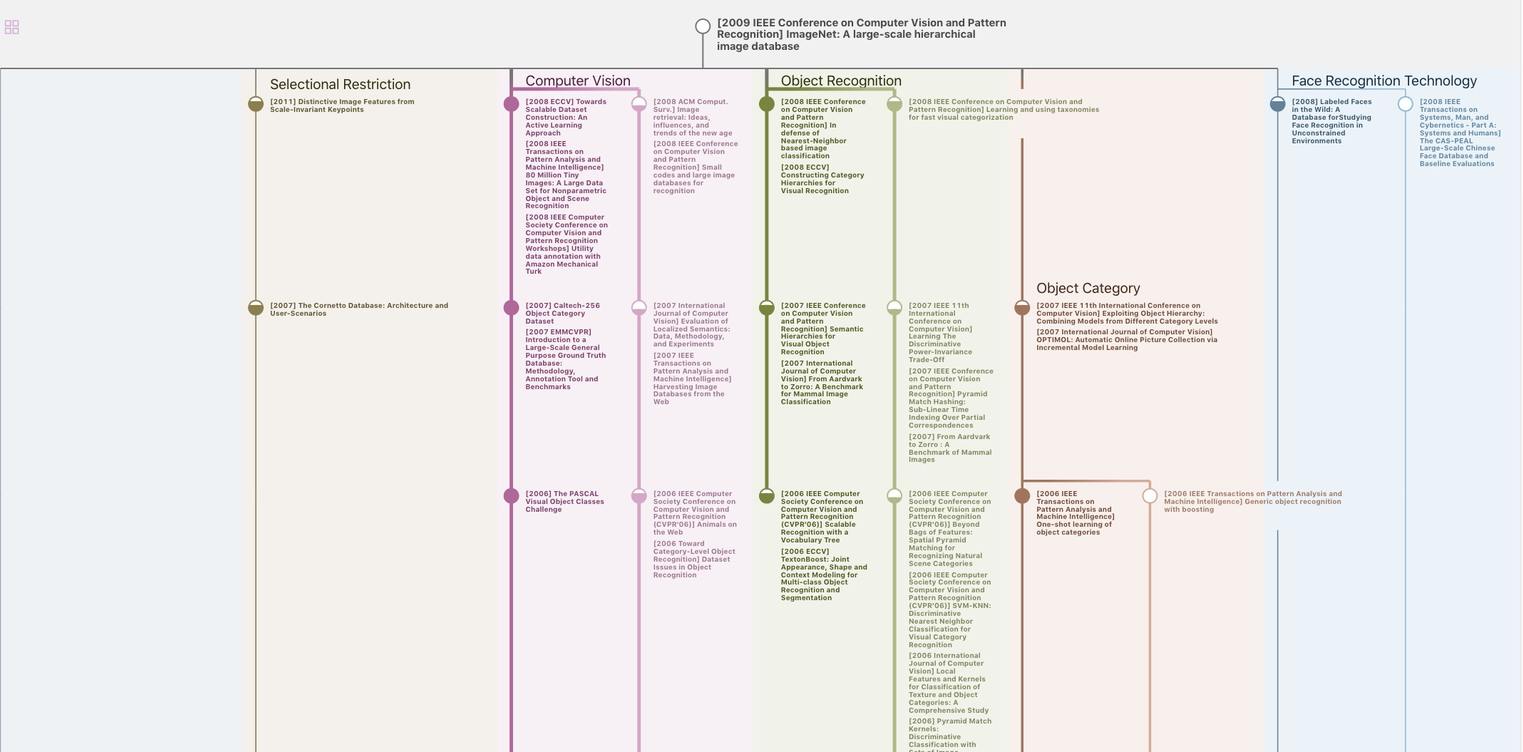
生成溯源树,研究论文发展脉络
Chat Paper
正在生成论文摘要