A Zero-Shot Deep Learning Approach for Unknown IoT Botnet Attack Detection.
RIVF International Conference on Computing and Communication Technologies(2023)
摘要
In recent years, as digitalization greatly advances, many homes and organizations have adopted Internet of Things (IoTs) networks to manage and regulate different devices in their infrastructure. This presents a vulnerability when a device is attacked by a Botnet as the whole network can be compromised. In such cases, one Botnet can have different behaviors when deployed in another device's environment or multiple Botnet families altogether can target different devices on the network. This poses a challenge in IoT Botnet detection for unseen Botnet families on unknown devices, as the data is unfamiliar. Machine learning (ML) algorithms and more specifically deep learning (DL) methods have proven to be effective in addressing the traditional detection approach where training data includes all samples of the attacking Botnet. However, with new attack data, it is still a remaining problem to accurately discern malicious flows from benign traffic. In this paper, we propose a zero-shot DL approach to detect Botnet samples from both seen and unseen families on unknown devices. Specifically, we propose a Deep Sparse Contrastive Auto-Encoder (DSCAE) model for Botnet detection by boosting performance with the meaningful latent representation of features. We also tested our approach against classical ML methods as well as simple Auto-Encoder. The results demonstrate that our proposed model is able to improve results in most cases and often achieves the highest metric values.
更多查看译文
关键词
IoT Botnet detection,Deep Sparse Contrastive Auto- Encoder,Zero-shot learning,Deep learning
AI 理解论文
溯源树
样例
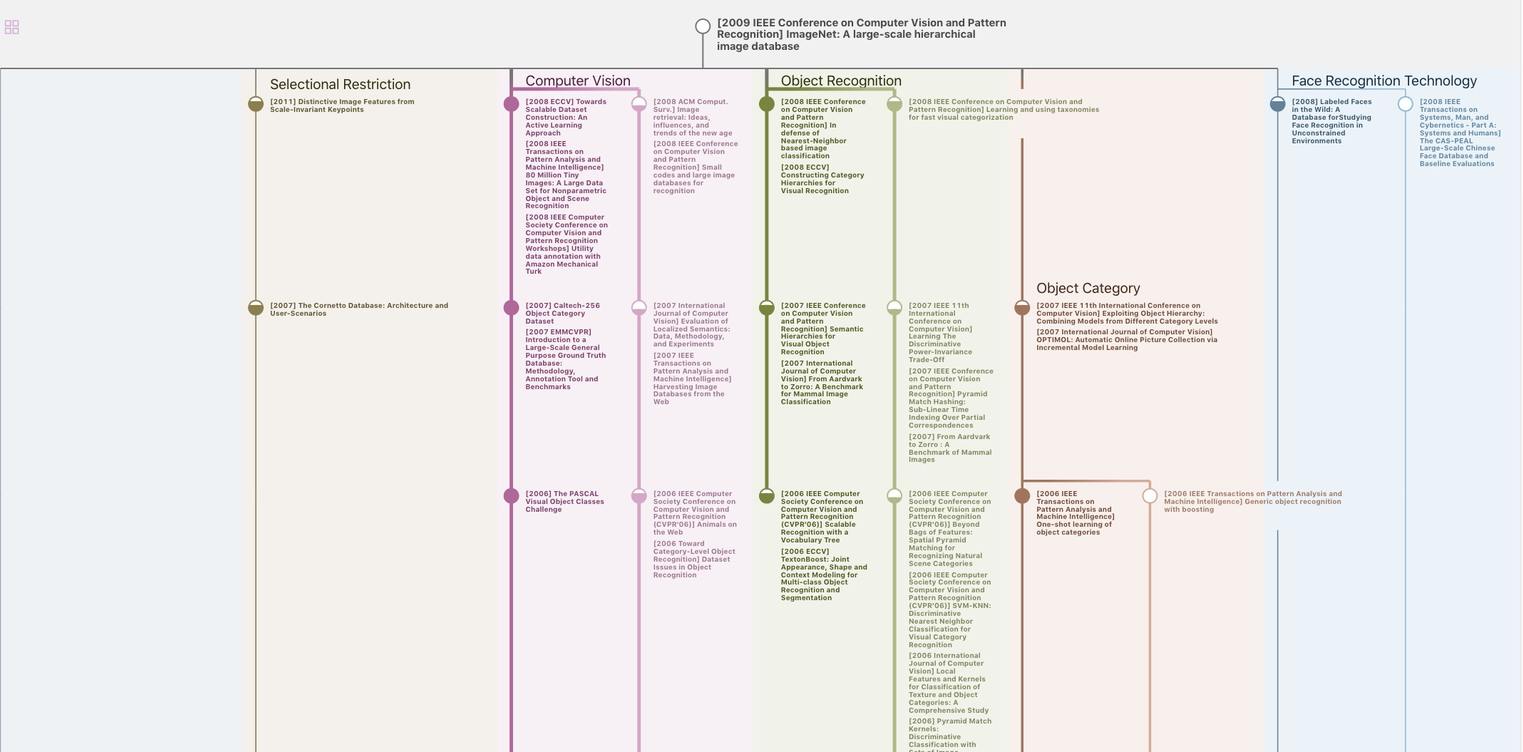
生成溯源树,研究论文发展脉络
Chat Paper
正在生成论文摘要