AMP-BiLSTM: An Enhanced Highlight Extraction Method Using Multi-Channel Bi-LSTM and Self-Attention in Streaming Videos.
IEEE International Conference on Semantic Computing(2024)
摘要
With the rise of conversation-oriented streaming videos, the platforms that host them like Twitch have rapidly become prominent information hubs. However, the lengthy nature of such streams often deters viewers from consuming the full content. To mitigate this, we propose AMP-BiLSTM, a novel highlight extraction method which focuses on the textual information in streamer discourses and viewer responses rather than visual features. This approach addresses the limitations of previous methods, which primarily centered on analyzing visual features, and were thus insufficient for conversation-oriented streams where highlights emerge from dialogues and viewer interactions. AMP-BiLSTM is built on techniques of Attention, Multi-channel, and Position enrichment integrated into a Bidirectional Long Short-Term Memory (BiLSTM) network. Through experiments on a real-world dataset, we found that streamer discourses and viewer messages provide significant utility for highlight extraction in conversation-oriented streaming videos. Furthermore, our proposed Multi-channel and self-attention techniques effectively distill text streams into semantically-rich embeddings. The experiment results demonstrate that AMP-BiLSTM outperforms several state-of-the-art methods for deep learning-based highlight extraction, thus showing promise for improved conversation-oriented streaming video content digestion.
更多查看译文
关键词
Highlight Extraction,Conversation-Oriented Streaming Video,Deep Learning,BiLSTM,Attention Mechanism,Multi-channel Analysis,Position Enrichment
AI 理解论文
溯源树
样例
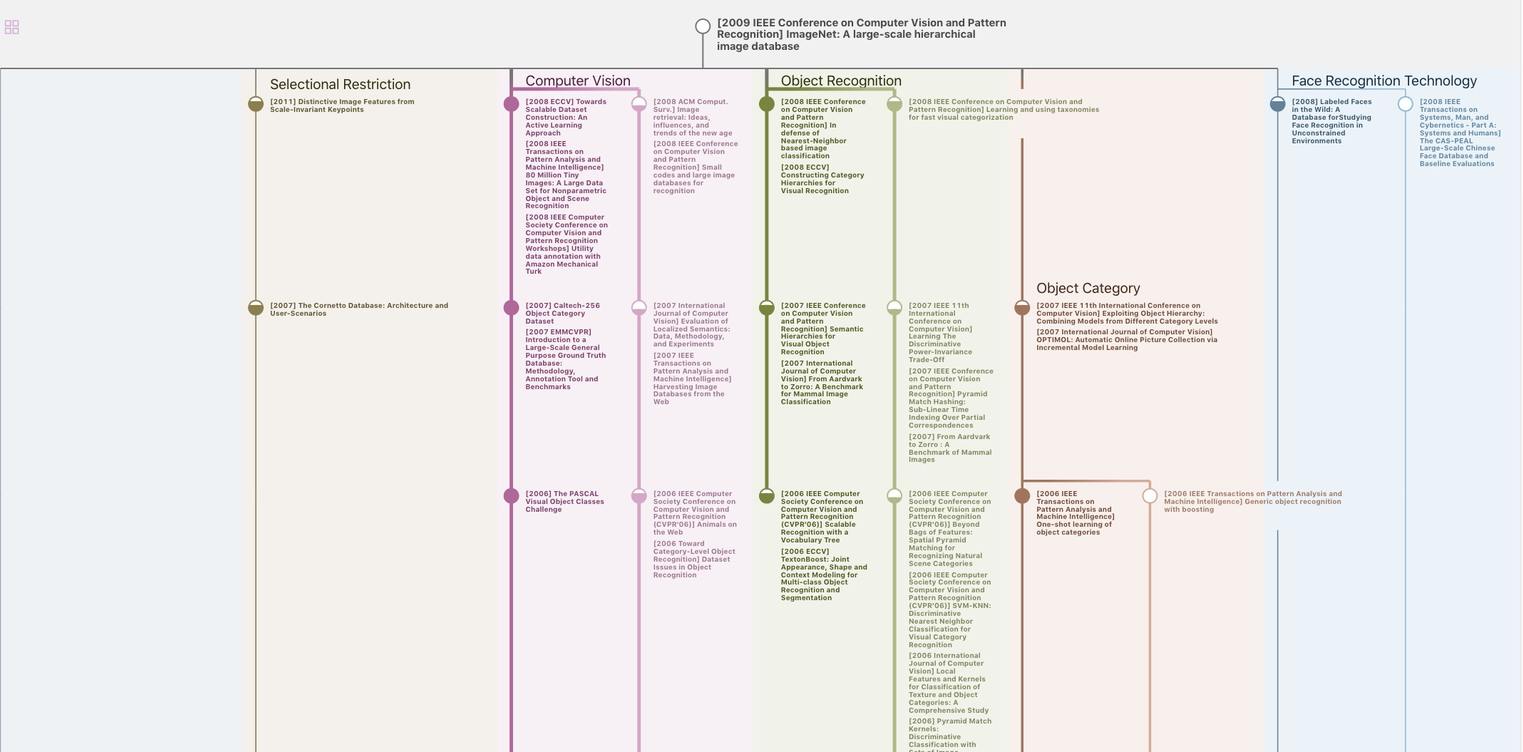
生成溯源树,研究论文发展脉络
Chat Paper
正在生成论文摘要