Abstract 7400: AI-driven multimodal 3D tumor modeling with spatial molecular insights for predicting ICI response in gastric cancer
Cancer Research(2024)
Abstract
Abstract Traditional cancer research methodologies predominantly focus on 2D tumor modeling, including spatial profiling and AI-driven analysis at the 2D level. To advance beyond these limitations, we implemented Holotomography (HT), a 3D, label-free optical imaging method. This technique, leveraging refractive index contrasts, allows for enhanced visualization of tumor tissues, organoids, and cells in three dimensions. Using serial H&E sections and unstained slides, we created detailed 3D tumor models in gastric cancer, providing insights into tumor, immune, and stromal composition, as well as cell-cell interactions and extracellular matrix characteristics. AI algorithms further enhanced our 3D tumor modeling, offering novel perspectives on the initiation and progression of tumor, immune, and stromal components at the subcellular level. Analyzing gastric tumors treated with immune checkpoint inhibitors before, during, and after treatment revealed dynamic changes in spatial composition over time, offering unique insights into the evolution of the 3D tumor ecosystem. Additionally, by integrating spatial transcriptomic and in situ molecular imaging data, we developed a novel Multimodal 3D Tumor Model. This model not only uncovers subcellular-level molecular characteristics but also elucidates cellular interactions during treatment, suggesting potential biomarkers and therapeutic approaches. Lastly, by constructing 3D cellular interaction networks incorporating true 3D interactions, we provided an in-depth view of cellular communication within the tumor microenvironment. This pioneering approach, representing the first Multimodal 3D Tumor Model at the subcellular level, offers novel insights into gastric cancer's spatial evolution, potentially revolutionizing our understanding and treatment of gastric cancer. Citation Format: Yoon Ho Choi, Minji Kim, Katya Redekop, Inyeop Jang, Jean R. Clemenceau, Isabel Barnfather, Sumanth Reddy Nakkireddy, Changjin Hong, Sunho Park, Ji-Youn Sung, Jeong Hwan Park, Shitara Kohei, Aoki Yu, Sung Hak Lee, Sam Wang, Jaeho Cheong, Tae Hyun Hwang. AI-driven multimodal 3D tumor modeling with spatial molecular insights for predicting ICI response in gastric cancer [abstract]. In: Proceedings of the American Association for Cancer Research Annual Meeting 2024; Part 1 (Regular Abstracts); 2024 Apr 5-10; San Diego, CA. Philadelphia (PA): AACR; Cancer Res 2024;84(6_Suppl):Abstract nr 7400.
MoreTranslated text
AI Read Science
Must-Reading Tree
Example
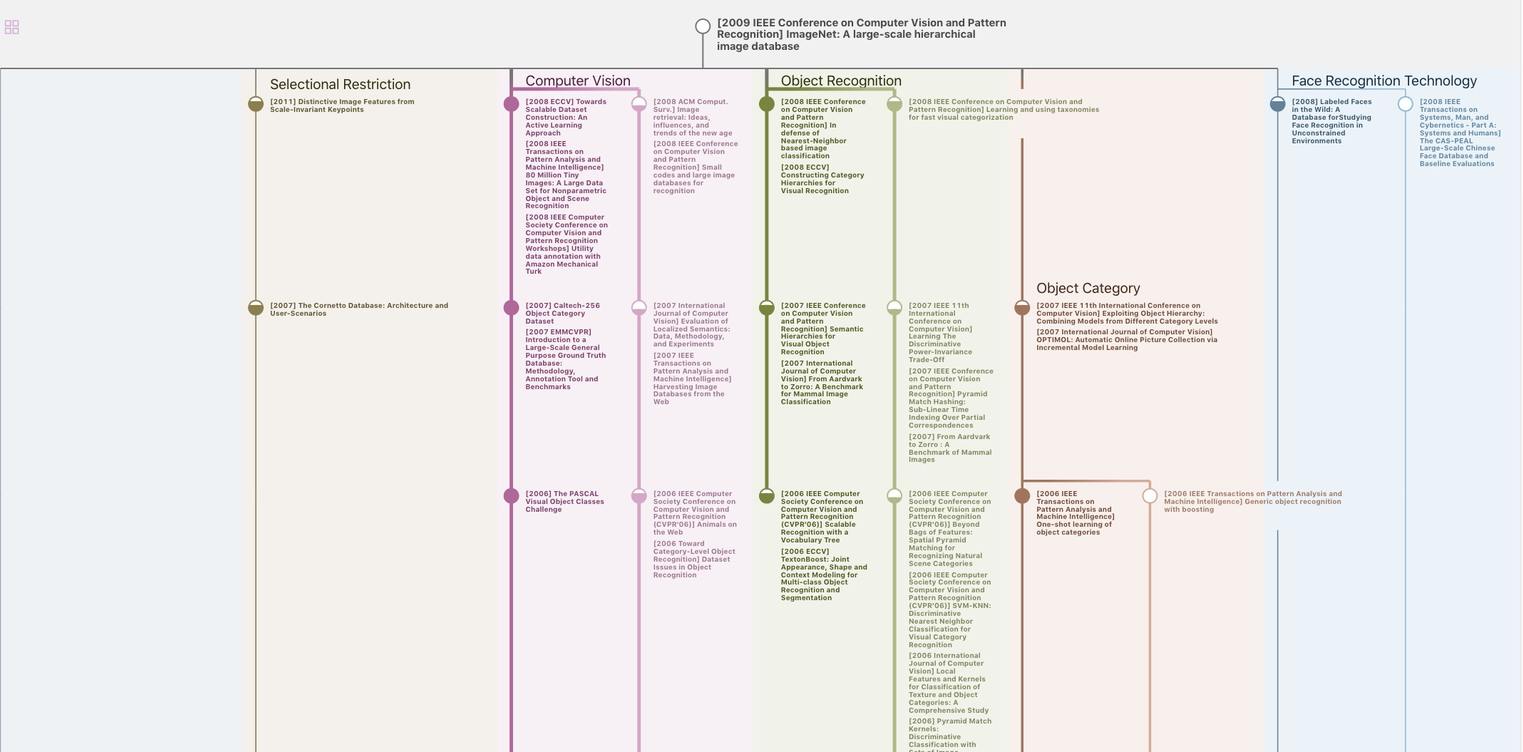
Generate MRT to find the research sequence of this paper
Chat Paper
Summary is being generated by the instructions you defined