Classification Model of the Impact of Psychological Factors on Children's Academic Performance Based on Machine Learning
2023 IEEE 3rd International Conference on Social Sciences and Intelligence Management (SSIM)(2023)
摘要
The academic performance of primary school students varies, influenced by factors such as age, gender, and parental education, as well as psychological factors such as anxiety, depression, and sense of agency (SoA). We analyzed the academic performance of primary school students in a binary mathematical problem approach. Firstly, the academic performance was quantified into a binary form through histogram analysis, creating a binary learning scenario. Then, correlation analysis was conducted to identify features with a strong correlation and academic performance. Finally, the CHAID decision tree algorithm, a machine learning technique, was applied to construct a binary classification model for academic performance. The experimental results demonstrated that academic performance was positively correlated with age, father's education, mother's education, and students' SoA scores. Conversely, it was negatively correlated with depression scores. However, the correlation between gender and anxiety was not significant. The decision tree model validated the results of the correlation analysis and provided a profile of students with better academic performance, including older age, higher parental education, and higher SoA scores. The accuracy of the decision tree model was 74.1% showing practical implications for teaching. This research results highlighted the importance of considering various factors, such as age, parental education, and psychological factors, in understanding and predicting primary school students' academic performance.
更多查看译文
关键词
machine learning,decision tree,psychological factors,sense of agency,academic performance
AI 理解论文
溯源树
样例
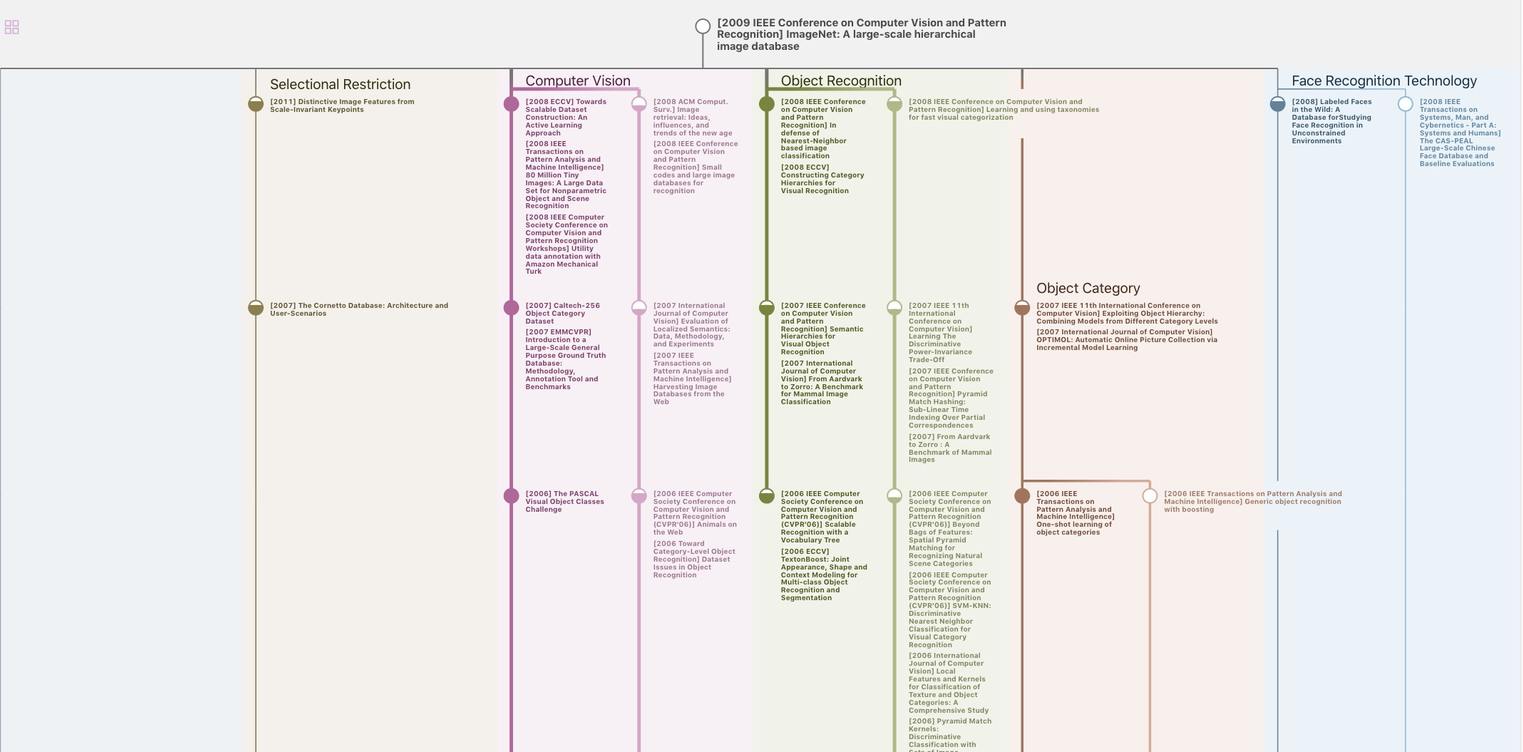
生成溯源树,研究论文发展脉络
Chat Paper
正在生成论文摘要