A Self-Attention-Based Deep Convolutional Neural Networks for IIoT Networks Intrusion Detection
IEEE ACCESS(2024)
摘要
The Industrial Internet of Things (IIoT) comprises a variety of systems, smart devices, and an extensive range of communication protocols. Hence, these systems face susceptibility to privacy and security challenges, making them prime targets for malicious attacks that can result in harm to the overall system. Privacy breach issues are a notable concern within the realm of IIoT. Various intrusion detection systems based on machine learning (ML) and deep learning (DL) have been introduced to detect malicious activities within these networks and identify attacks. However, traditional ML and DL models encounter significant hurdles when faced with highly imbalanced training data and repetitive patterns within network datasets, hampering their performance in distinguishing between various classes of attacks. To overcome the challenges inherent in existing systems, this paper presents a self-attention-based deep convolutional neural network (SA-DCNN) model designed for monitoring the IIoT networks and detecting malicious activities. The SA mechanism computes the significance value for each input feature, and the DCNN processes these parameters to detect IIoT network behavior. Additionally, a two-step cleaning method has been implemented to eliminate redundancy within the training data, considering both intra-class and cross-class samples. Furthermore, to tackle the issue of underfitting, we have employed a mutual information-based feature filtering method. This method ranks all the features in descending order based on their mutual information and subsequently removes the features with negative impact from the dataset. The performance of the SA-DCNN model is assessed using IoTID20 and Edge-IIoTset datasets. Moreover, the proposed study is demonstrated through a comprehensive comparison with other ML and DL models, as well as against relevant studies, showcasing the superior performance and efficacy of the proposed model.
更多查看译文
关键词
Industrial Internet of Things,Data models,Filtering,Cleaning,Convolutional neural networks,Computational modeling,Training,Intrusion detection,Smart devices,Deep learning,Attention mechanism,CNN,deep learning,IIoT,intrusion detection
AI 理解论文
溯源树
样例
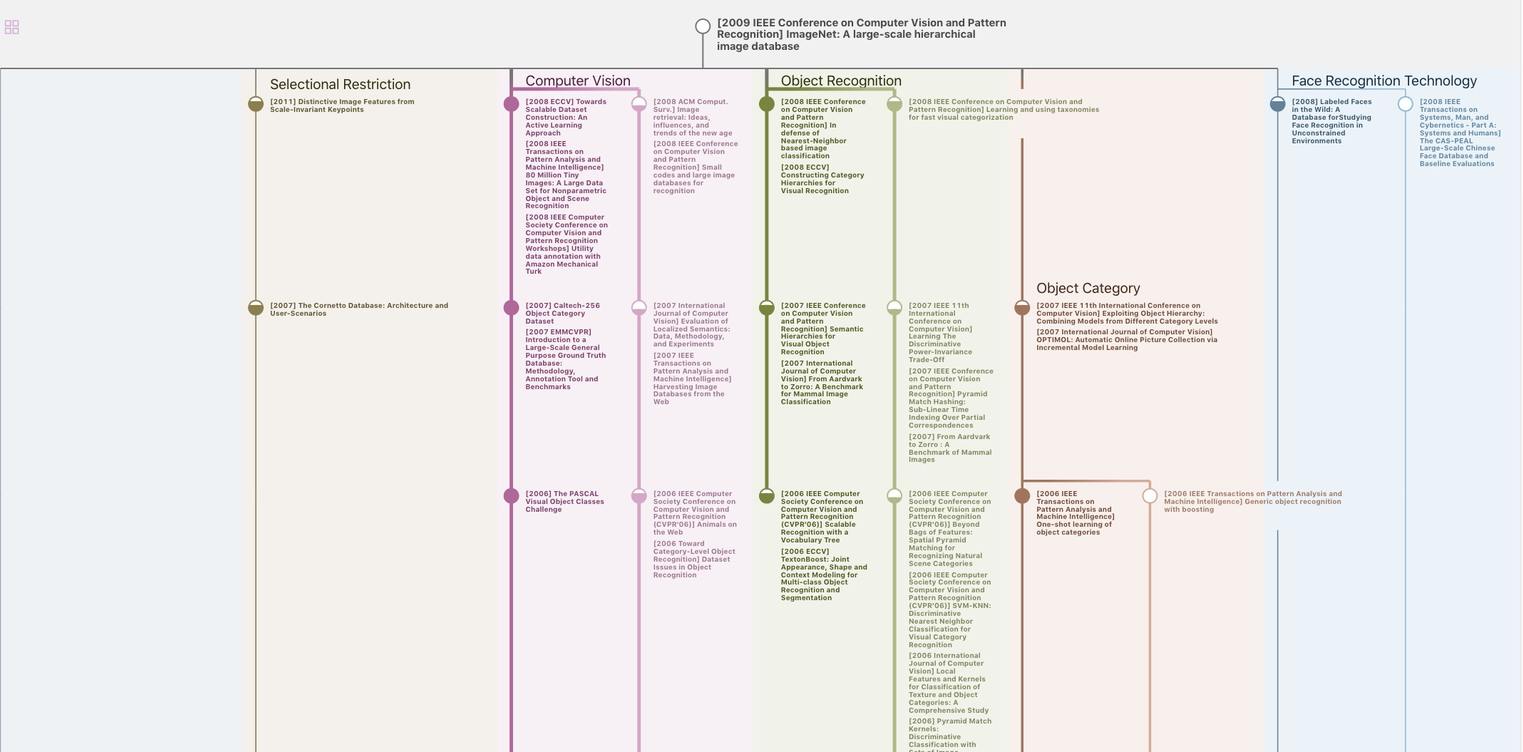
生成溯源树,研究论文发展脉络
Chat Paper
正在生成论文摘要