Abstract 6245: Missed opportunity at the subcellular level: Enhancing the utility of cellular imaging modality in spatial transcriptomic profiling of tumor tissues
Cancer Research(2024)
摘要
Abstract Mini-bulk or single-spot spatial transcriptomic technologies, such as GeoMX Digital Spatial Profiler (DSP), have revolutionized our ability to probe spatial heterogeneity and examine transcripts at a subcellular level. In cancer research, this level of granularity is crucial for uncovering the distinct gene expression signatures within specific compartments or structures of interest such as tumor, immune, stroma, and tertiary lymphoid structures. However, in most GeoMX DSP studies, the spatial information obtained from multiplex immunofluorescence imaging has been primarily used for identifying the regions of interest (ROI), rather than as an integral part of the downstream transcriptomic data interpretation. To fix this missed opportunity, we developed a machine learning based analytical framework to fully leverage the rich spatial context provided by the in situ imaging modality. The framework has two main functionalities. (1) ROI cropping and cell segmentation: our framework enables the automated cropping of ROI images, followed by a cell segmentation process utilizing a multi-color-optimized deep learning model pre-trained based on the TissueNet. (2) Cell typing and integrative spatial analysis: the pipeline extracts cell-level morphological features (e.g. cell size and circularity), and AI-driven characteristics. These features are subsequently employed for cell typing and the quantification of cell mixture. The framework is equipped to perform a series of advanced spatial analyses, such as spatial metrics calculation and consensus clustering analysis with the matched gene expression data. To validate the framework, we conducted GeoMX DSP analyses on samples obtained from bladder cancer and upper tract urothelial carcinoma, encompassing 56 ROIs annotated by pathologists. Our results demonstrated high accuracy, with the calculated tumor-immune proportions aligning precisely with the original annotations. We further compared the cell deconvolution results of our framework with those obtained using SpatialDecon. Consistency was noted across both methods, with a high correlation reaching 0.90. However, we observed that SpatialDecon tends to underestimate tumor purity in tumor core regions, with discrepancies as large as 0.30, and overestimating tumor purity in stromal regions. Additionally, analysis of ROIs in tumor margins revealed that SpatialDecon consistently overestimates the overall proportions of immune cells. In conclusion, our results underscore the importance of integrating in situ imaging with subcellular-level spatial transcriptomics for a more accurate and reliable analysis of tumor tissues. Our approach provides critical insights into the tumor microenvironment and cellular interactions, with significant implications for both research and clinical applications in oncology. Citation Format: Xiaofei Song, Xiaoqing Yu, Carlos M. Moran-Segura, G Daniel Grass, Roger Li, Xuefeng Wang. Missed opportunity at the subcellular level: Enhancing the utility of cellular imaging modality in spatial transcriptomic profiling of tumor tissues [abstract]. In: Proceedings of the American Association for Cancer Research Annual Meeting 2024; Part 1 (Regular Abstracts); 2024 Apr 5-10; San Diego, CA. Philadelphia (PA): AACR; Cancer Res 2024;84(6_Suppl):Abstract nr 6245.
更多查看译文
AI 理解论文
溯源树
样例
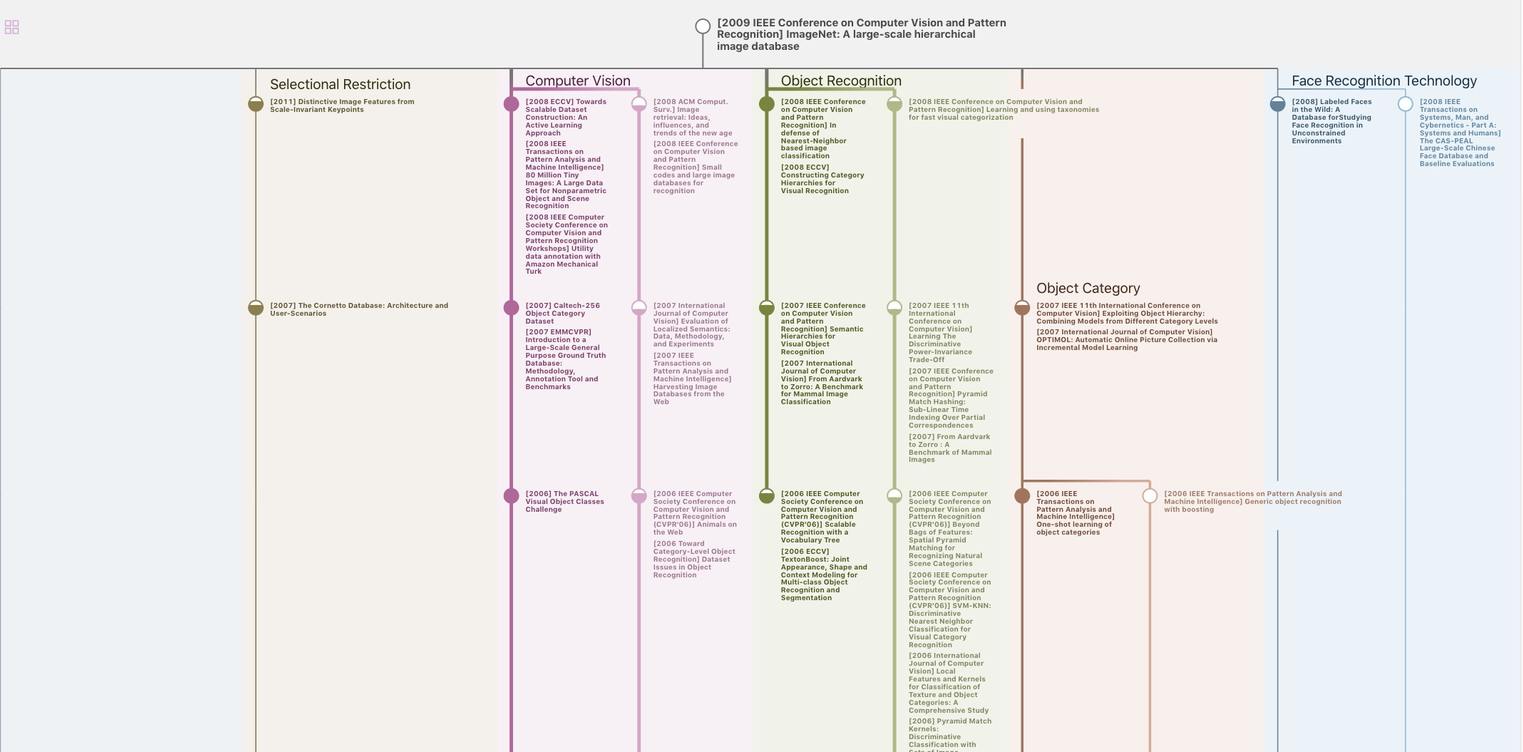
生成溯源树,研究论文发展脉络
Chat Paper
正在生成论文摘要