Adaptive Merging Migration
GEOPHYSICS(2024)
Abstract
Velocity errors and data noise are inevitable for seismic imaging of field datasets in current production; therefore, it is desirable to improve the seismic images as part of the migration process to mitigate the influence of such errors and noise. To address this, we have developed a new method of adaptive merging migration (AMM). This method can produce migrated sections of equal quality to conventional migration methods given a correct velocity model and noise-free data. Additionally, it can ameliorate the seismic image quality when applied with erroneous migration velocity models or noisy seismic data. AMM employs an efficient recursive Radon transform to generate multiple p-component images, representing migrated sections associated with different local plane slopes. It then adaptively merges the subsections from those p-component images that are less distorted by velocity errors or noise into the whole image. Such merging is implemented by computing adaptive weights followed by a selective stacking. We use three synthetic velocity models and one field dataset to evaluate the AMM performance on isolated Gaussian velocity errors, inaccurate smoothed velocities, velocity errors around high-contrast and short-wavelength interfaces, and noisy seismic data. Numerical tests conducted on both synthetic and field datasets validate that AMM can effectively improve the seismic image quality in the presence of different types of velocity errors and data noise.
MoreTranslated text
AI Read Science
Must-Reading Tree
Example
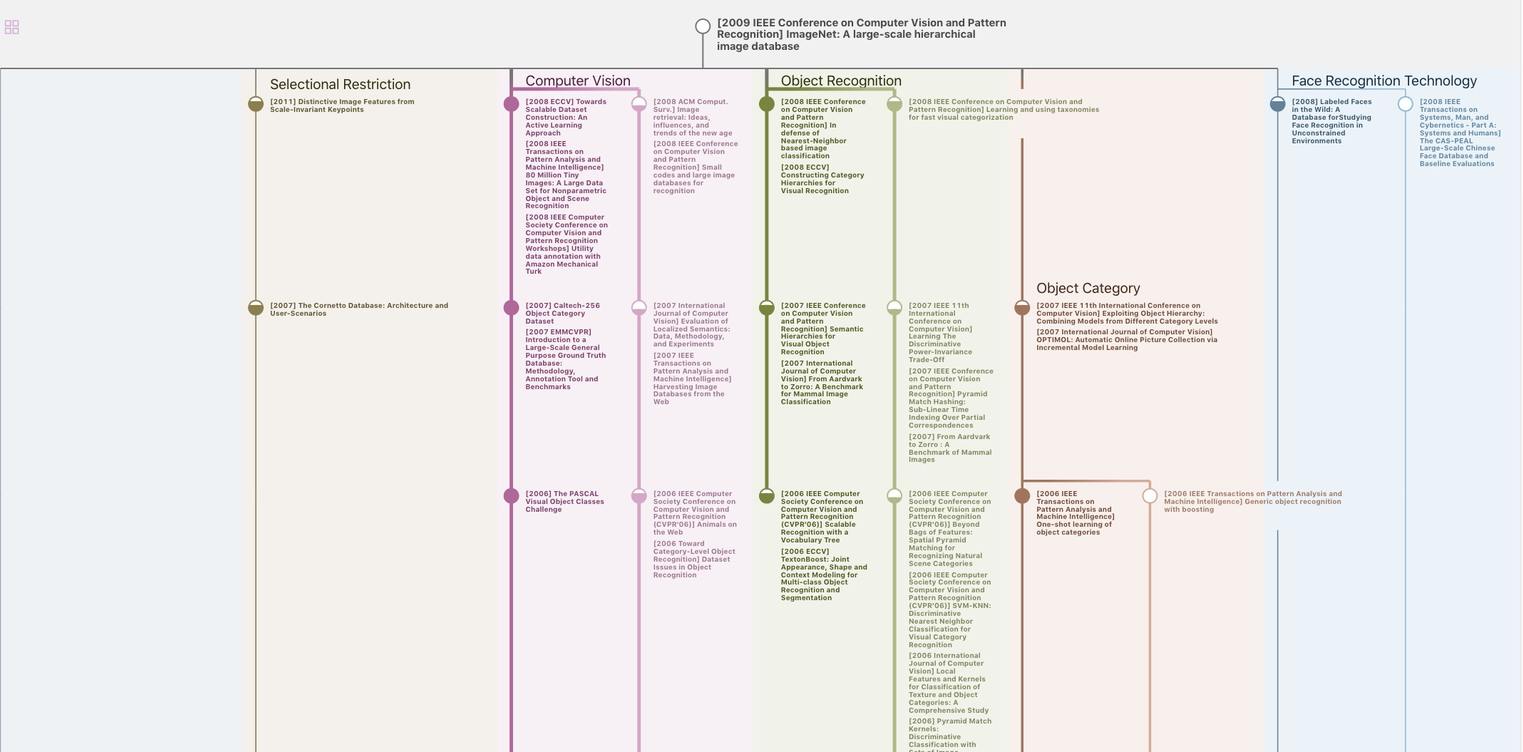
Generate MRT to find the research sequence of this paper
Chat Paper
Summary is being generated by the instructions you defined