Complex Semantic Tabular Interpretation using SDD-Gen.
IEEE International Conference on Semantic Computing(2024)
摘要
Knowledge graphs have become an essential technology for both businesses and governments. They enable a wide variety of critical tasks, such as aligning diverse datasets, improving the capabilities of search engines, supporting error checking, and generating explanations using inference engines. However, populating, augmenting, and/or validating a knowledge graph can be challenging because developers need domain knowledge to understand their data and experience in ontology modeling to align concepts properly as well as experience with conflict detection and truth maintenance tools. Previous efforts have explored automatically integrating simple tabular data into knowledge graphs to lower the barrier to entry. These methods heavily rely on named entity overlap and require that tables are similar to relational tables in third normal form. While these methods have been successful under competition, these limitations make them impractical for general usage. In this paper, we introduce the semantic data dictionary generator (SDD-Gen), an algorithm that aligns complex tabular data to ontological terms for knowledge graph generation. Our methodology leverages context information from data dictionaries to make alignments, enabling us to align complex tables with few named entities and multiple subject columns.
更多查看译文
关键词
Tabular Data,Knowledge Graph,Semantic Annotation
AI 理解论文
溯源树
样例
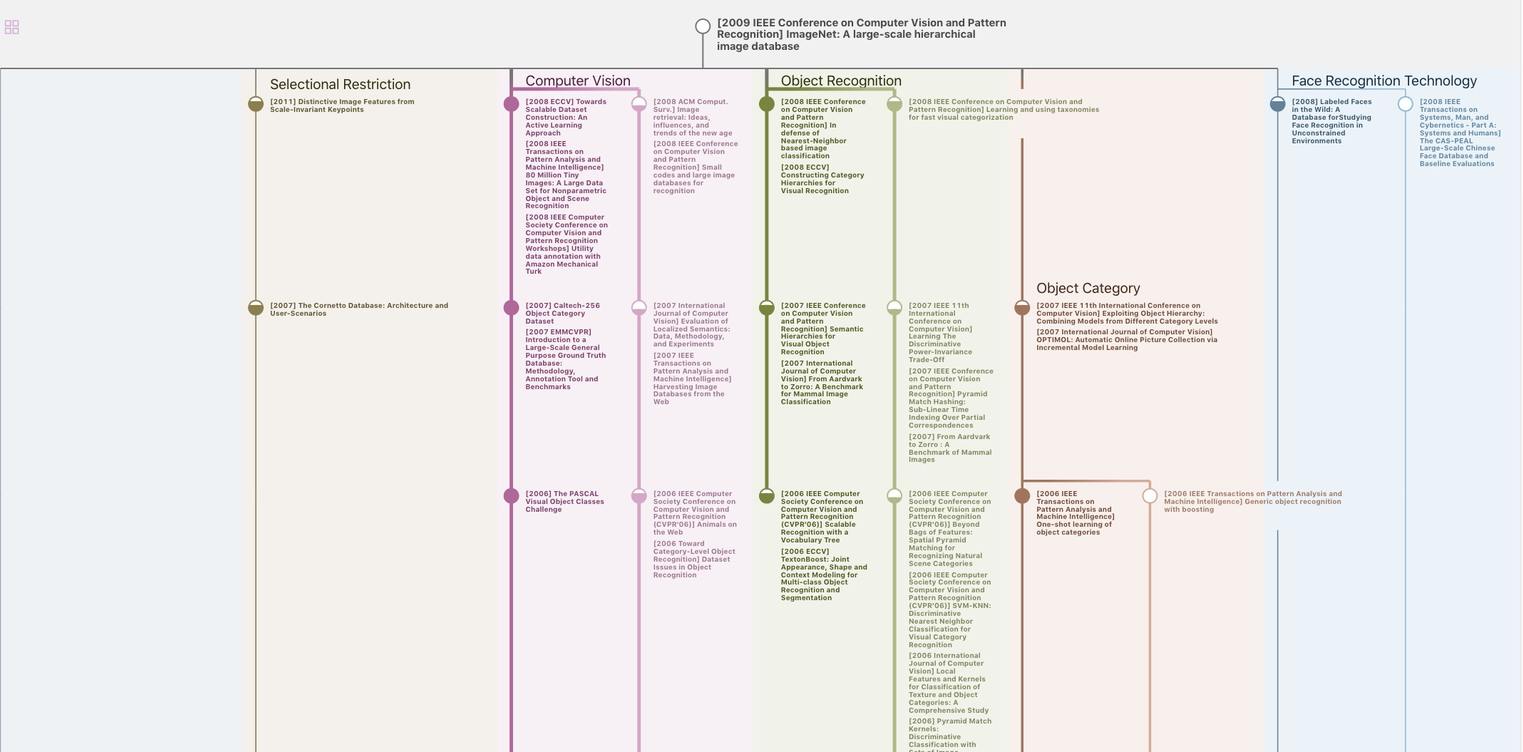
生成溯源树,研究论文发展脉络
Chat Paper
正在生成论文摘要