Semi-physical simulation-driven contrastive decoupling net for intelligent fault diagnosis of unseen machines under varying speed
MEASUREMENT SCIENCE AND TECHNOLOGY(2024)
摘要
Multi-source domain generalization provides a good idea for intelligent fault diagnosis of unseen machines. However, the acquisition of multi-source training sets would not only affect the generalization effect, but also cause huge economic loss. Too much difference between source training sets would lead to negative transfer, while too much similarity between the sets would cause zero transfer. Simultaneously, the current methods based on the assumption of stable speed cannot realize intelligent diagnosis under varying speed. Therefore, a semi-physical simulation-driven contrastive decoupling network is presented. First, a phenomenological model is used to generate large amount of simulation data with different modulation frequencies and signal-to-noise ratios to provide support for the training of the net. Next, with the joint help of simulation data and physical data, a contrastive framework is introduced to extract domain-independent features. Finally, a decoupling framework is proposed to minimize speed-related information while maximizing health-related features, thereby enabling intelligent diagnosis of unseen devices at varying speed. The proposed method is tested on two cases operating under sharp speed variation and compared with some state-of-the-art methods. The results show the superiority of the proposed net in intelligent diagnosis of unseen equipment under drastically varying speed.
更多查看译文
关键词
domain generalization,feature decoupling,intelligent fault diagnosis,phenomenological modeling,varying speed
AI 理解论文
溯源树
样例
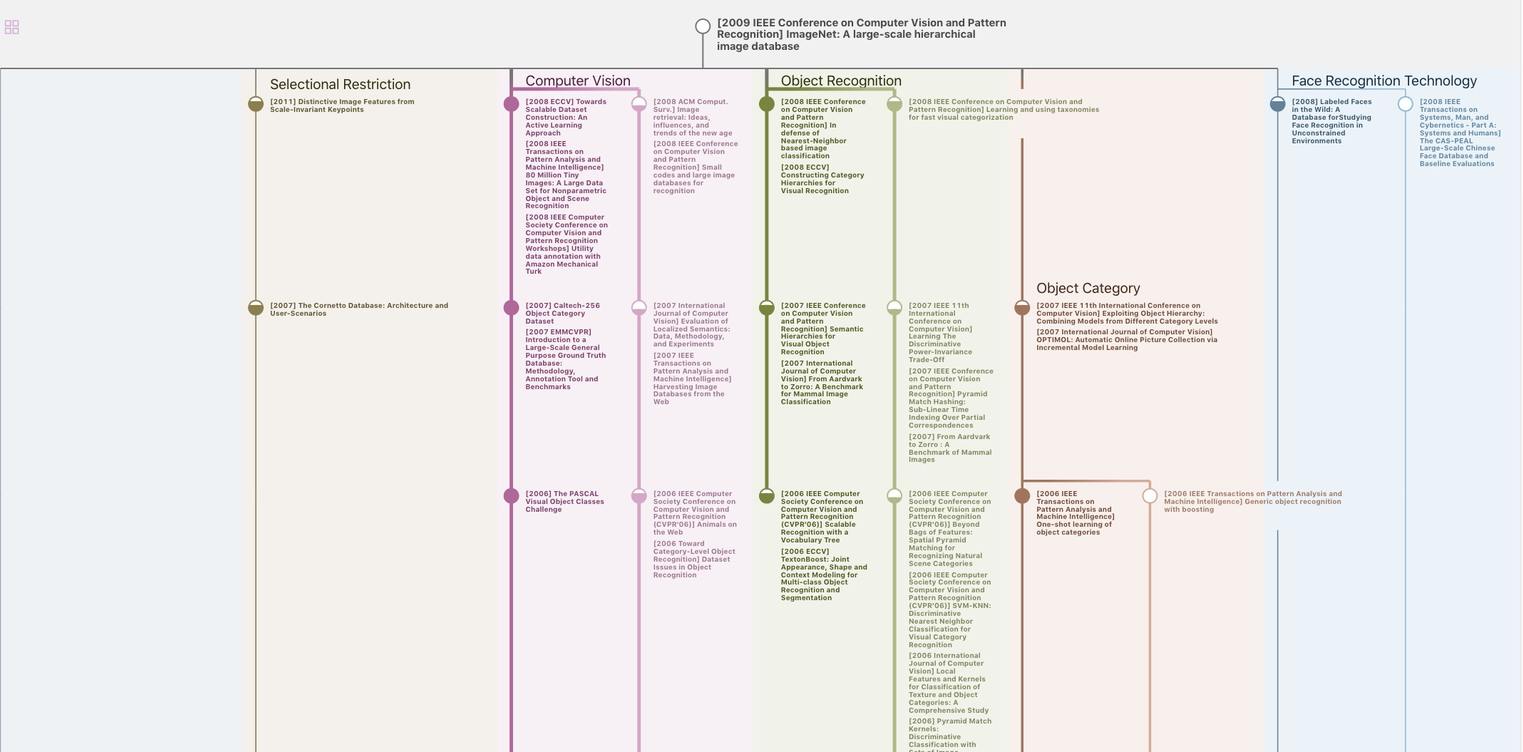
生成溯源树,研究论文发展脉络
Chat Paper
正在生成论文摘要