Abstract 4924: Assessing microsatellite instability dominance in colorectal cancer phenotype: A multi-study initiative using multi-target transformers for genomic biomarker prediction
Cancer Research(2024)
摘要
Abstract We used deep learning (DL) to predict multiple genetic biomarkers of colorectal cancer (CRC) from routine histopathology images, aiming to identify morphological changes associated with the mutational profile of tumors. A new Transformer-based prediction model was developed which can simultaneously predict multiple biomarkers from a single histopathology image. We compared the performance of the multi-target approach to conventional single-target models. We analyzed five patient cohorts from the Genetics and Epidemiology of CRC Consortium (GECCO) with colorectal cancer (N=1,385 patients). Our model was trained on an internal data set (N=739) to predict the presence of >200 genetic alterations, focusing on genes with non-silent mutations and mutational signatures, which were assessed using targeted sequencing. We used a 7-fold cross validation and validated the model on an independent, external data set (N=646). Morphological features of microsatellite instability (MSI) are detectable by DL models in histopathology images, making MSI a potential confounding factor. Thus, we also assessed whether our model can predict genetic alterations regardless of MSI status, or whether the model identifies the MSI phenotype. For the majority of biomarkers, the multi-target transformer reached a higher prediction performance as measured by the mean Area Under the Receiver Operating Characteristic (AUROC) Curve and lower standard deviation than single-target DL models. For the external validation set, the mean AUROC of the multi-target versus the single-target model was 0.78 (+/- 0.01) versus 0.72 (+/- 0.06) for BRAF mutations, 0.88 (+/- 0.01) versus 0.86 (+/- 0.03) for hypermutation status, 0.94 (+/- 0.01) versus 0.91 (+/- 0.02) for MSI, 0.86 (+/- 0.01) versus 0.80 (+/- 0.05) for RNF43 mutations and 0.72 (+/- 0.02) versus 0.69 (+/- 0.05) for TP53 mutations, respectively. However, our analysis of the individual prediction scores suggests that the model does not identify the phenotype of the alterations themselves. Instead, the model seems to determine the status of specific genetic alterations based on their correlation with MSI. Consequently, biomarkers which are associated with microsatellite stability have prediction scores negatively correlated with predicted MSI scores. Conversely, biomarkers which are associated with MSI have prediction scores positively correlated with predicted MSI scores. Our results demonstrate potential benefits of multi-target DL models in improving the predictive power and efficacy of histology-based biomarker extraction compared to single-target DL models. However, our results crucially highlight the importance of considering potential confounders, such as MSI status in CRC, and emphasize the need for extensive analysis beyond AUROC in studies focusing on DL-based biomarker detection. Citation Format: Marco Gustav, Marko van Treeck, Zunamys I. Carrero, Chiara M. Loeffler, Nic G. Reitsam, Bruno Märkl, Asier Rabasco Meneghetti, Lisa A. Boardman, Amy J. French, Ellen L. Goode, Andrea Gsur, Stefanie Brezina, Marc J. Gunter, Neil Murphy, Paul Limburg, Stephen Thibodeau, Sebastian Foersch, Robert Steinfelder, Tabitha Harrison, Ulrike Peters, Amanda Phipps, Jakob N. Kather. Assessing microsatellite instability dominance in colorectal cancer phenotype: A multi-study initiative using multi-target transformers for genomic biomarker prediction [abstract]. In: Proceedings of the American Association for Cancer Research Annual Meeting 2024; Part 1 (Regular Abstracts); 2024 Apr 5-10; San Diego, CA. Philadelphia (PA): AACR; Cancer Res 2024;84(6_Suppl):Abstract nr 4924.
更多查看译文
AI 理解论文
溯源树
样例
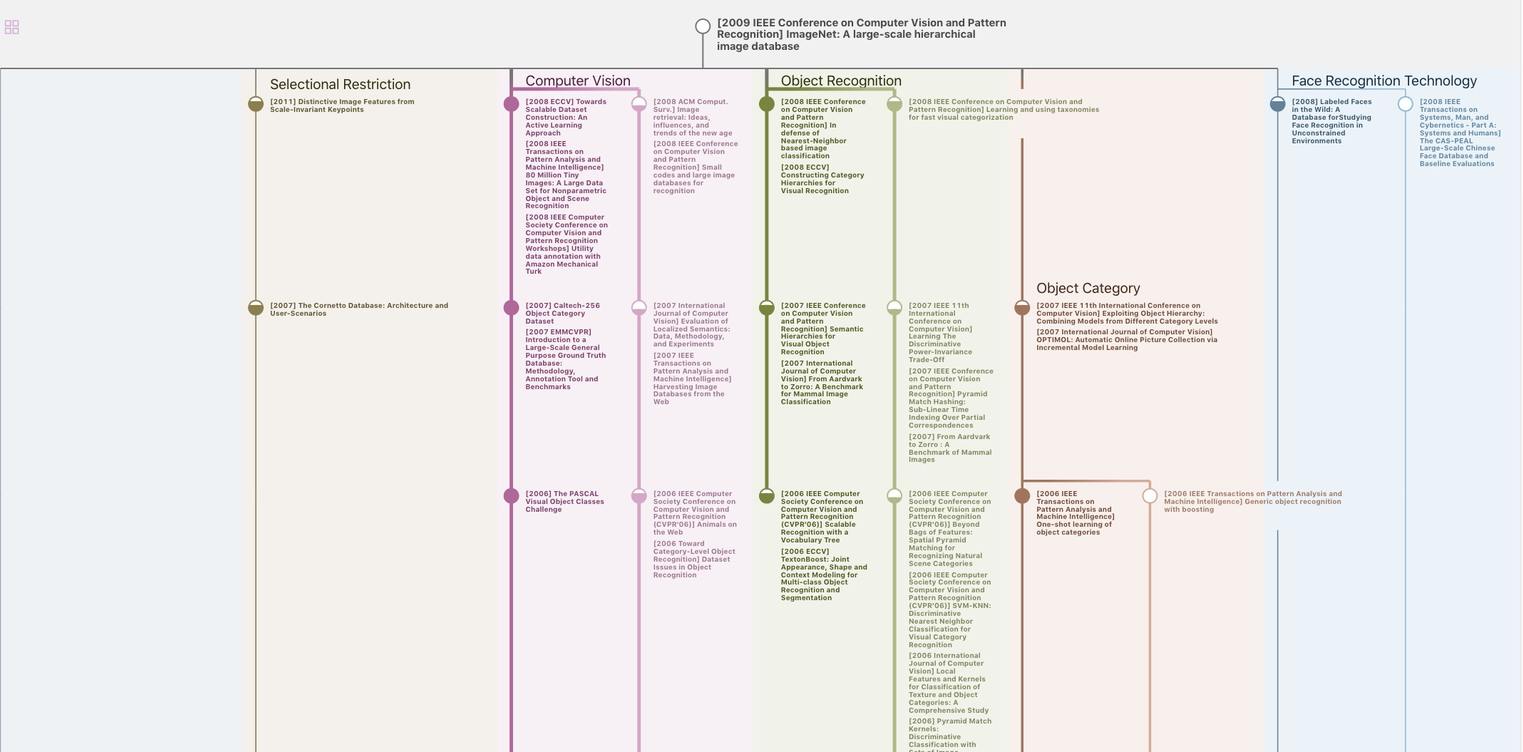
生成溯源树,研究论文发展脉络
Chat Paper
正在生成论文摘要