A Transfer Learning-Based Fine Tuned VGG16 Model for PCOS Classification
2024 2nd International Conference on Intelligent Data Communication Technologies and Internet of Things (IDCIoT)(2024)
摘要
The most prevalent hormonal condition is polycystic ovarian syndrome (PCOS), which occurs when the ovaries produce an excessive amount of small fluid-filled sacs known as follicles. Ovulation, the release of eggs, may not always occur as expected. An effective method for early PCOS diagnosis and treatment planning involves identifying multiple ovaries through ultrasonography (USG) scans. In this research, a dataset consisting of 1924 USG images of ovaries was utilized to develop and assess a deep-learning approach for predicting PCOS cycles. The images were sourced from the Kaggle repository. The proposed work utilizes a Convolutional Neural Network (CNN) based pre-trained VGG16 model to extract crucial features and differentiate between ovaries with and without PCOS. The experimental findings demonstrate that this VGG16 model achieved an impressive classification accuracy of 99.4% for distinguishing between PCOS and non-PCOS ovaries. Furthermore, this model can be extended by performing feature extraction with the pre-trained models and classification using neural networks for optimal and accurate results.
更多查看译文
关键词
Polycystic ovarian syndrome,deep learning,convolution neural network,visual geometry group,disease detection
AI 理解论文
溯源树
样例
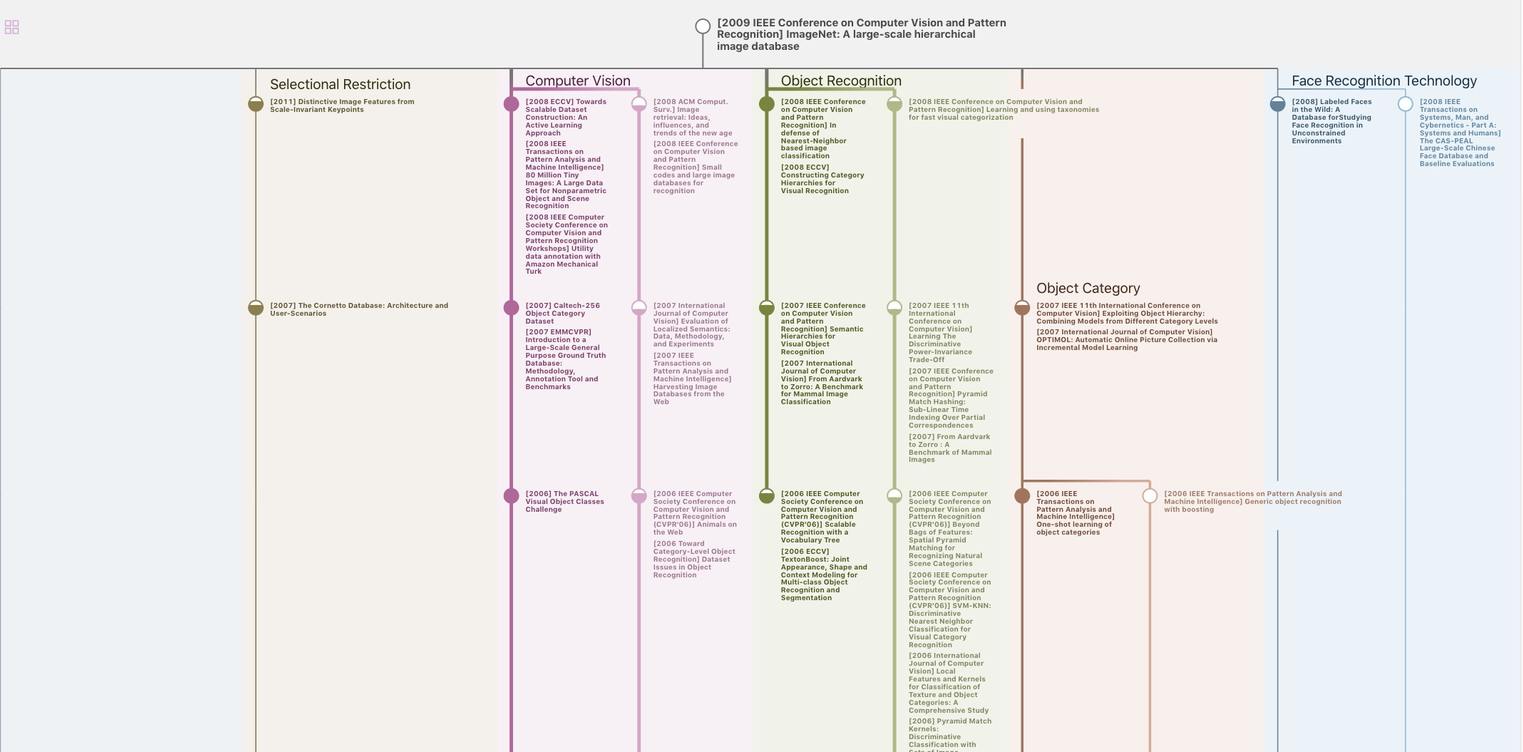
生成溯源树,研究论文发展脉络
Chat Paper
正在生成论文摘要