Evaluating Tabular and Textual Entity Linking in Financial Documents.
IEEE International Conference on Semantic Computing(2024)
摘要
Entity linking poses a longstanding challenge within natural language processing, a challenge that many studies have sought to address from diverse perspectives. However, these solutions have focused on open-domain knowledge bases. In contrast, our study shifts focus towards the financial domain, characterized by complexities, including intricate concept definitions and tables with complicated hierarchical structures. We introduce a novel dataset comprised of a hybrid mixture of tabular and textual inputs for entity linking, providing a total of 10,000 samples. The dataset requires predicting a concept that describes a specific table cell from the given table and surrounding texts. Our experiment includes three approaches. The first one is an approximate string-matching algorithm. The second is a fine-tuned language-model retriever. The last one involves prompting on a large language model. The results highlight the challenges inherent in this task, suggesting the need for further solutions.
更多查看译文
关键词
Entity linking,finance,knowledge base
AI 理解论文
溯源树
样例
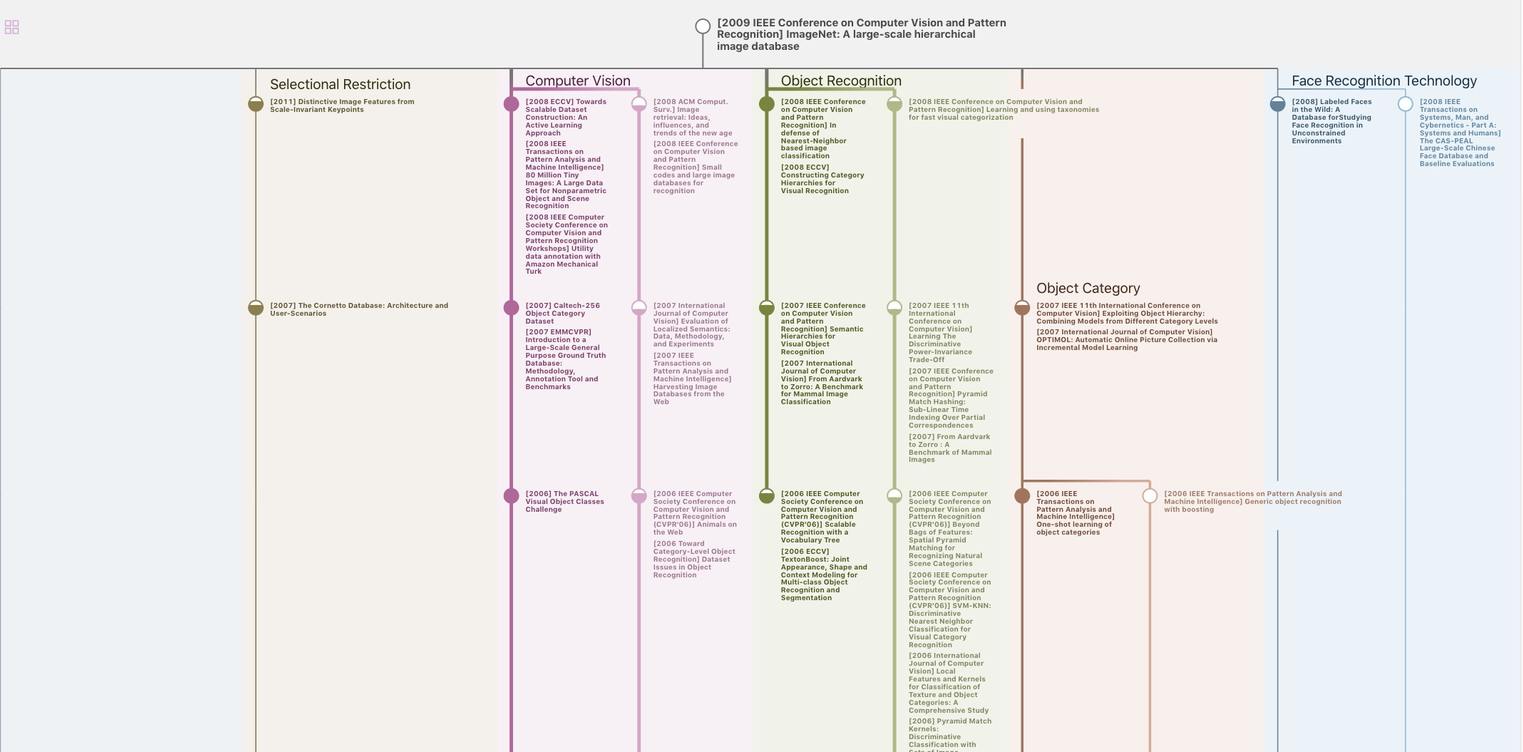
生成溯源树,研究论文发展脉络
Chat Paper
正在生成论文摘要