Nonparametric formulation of polynomial chaos expansion based on least-square support-vector machines
ENGINEERING APPLICATIONS OF ARTIFICIAL INTELLIGENCE(2024)
摘要
This paper introduces an innovative data -driven approach to uncertainty quantification (UQ) in complex engineering designs based on polynomial chaos expansion (PCE) and least-square support-vector machine (LSSVM). While PCE is a prevalent UQ method, its reliance on a predefined model form poses limitations in the model complexity, training accuracy, and efficiency in high-dimensional settings. To overcome this, we propose a nonparametric reformulation that draws equivalence with the kernel-based LSSVM. Leveraging special implicit kernels based on Hermite or Legendre polynomials, our method achieves accurate predictions with reduced training data. The model is trained through the dual space LSSVM formulation, thereby exploiting the so-called "kernel trick", while PCE coefficients up to an arbitrary order are efficiently obtained posttraining. Validation encompasses a diverse range of scenarios, including standard benchmarks and real-life applications in electrical engineering involving up to 26 independent random input parameters. The proposed "PCE-LSSVM"method exhibits superior performance over traditional PCE implementations. It achieves up to 7 times smaller root-mean-square error and up to 10 times lower dispersion across various training datasets, showcasing superior performance even with a significantly reduced number of training samples. Notably, this approach is 2 to 50 times more efficient compared to alternative approaches such as ordinary least square regression or Gaussian quadratures. Overall, PCE-LSSVM emerges as a powerful and efficient tool for accurate UQ in complex engineering systems.
更多查看译文
关键词
Kernel,Least-square support-vector machines,Machine learning,Polynomial chaos,Uncertainty quantification
AI 理解论文
溯源树
样例
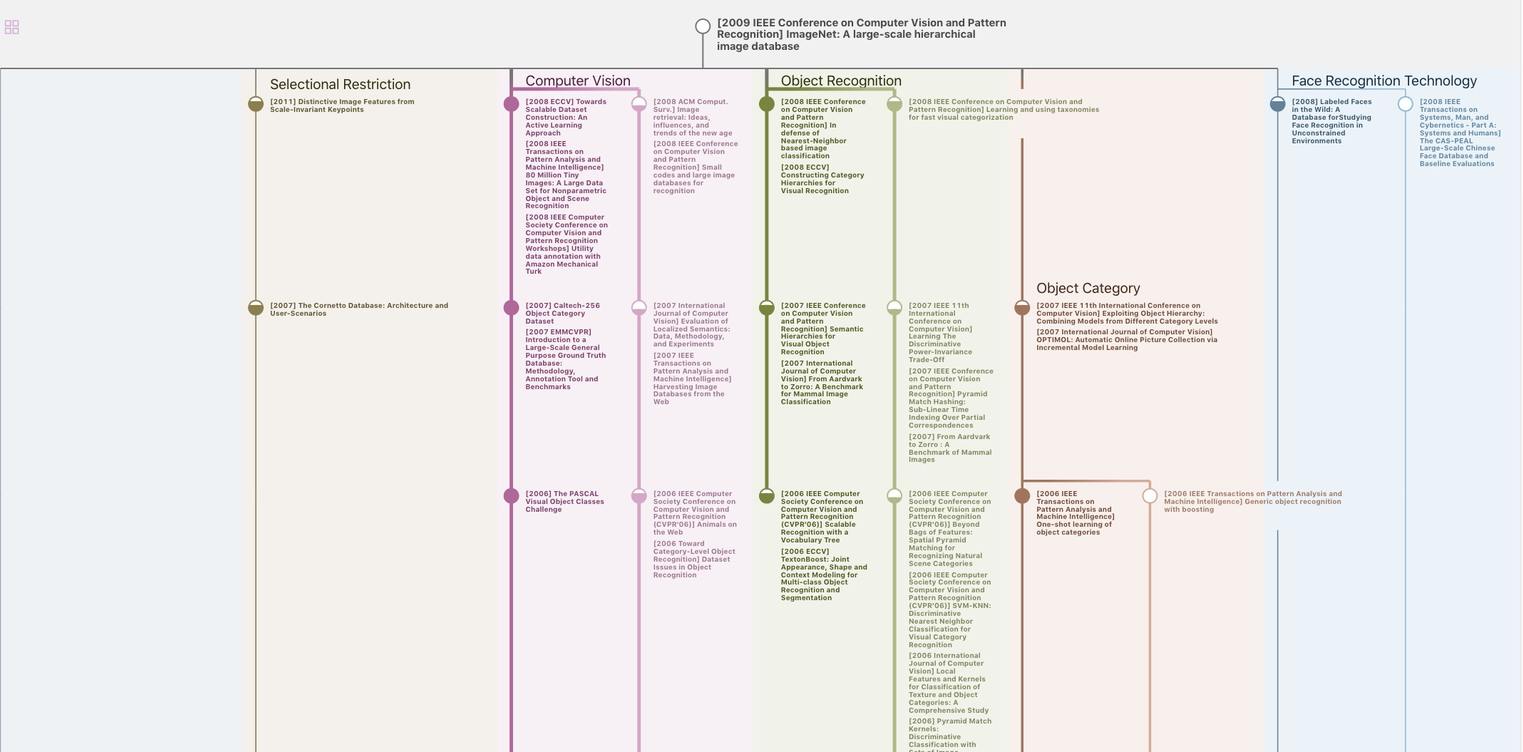
生成溯源树,研究论文发展脉络
Chat Paper
正在生成论文摘要