Advancing athlete development: how percentile comparison methods can identify youth developmental profiles (PCMs)
Journal of Science and Medicine in Sport(2024)
Abstract
Objectives
Inter-individual developmental differences confound the capability to accurately evaluate youth athletic performance, highlighting the need for considerate methodology and analytical approaches. The present study demonstrated how Percentile Comparison Methods (PCMs) were developed, tested, and applied to identify athlete developmental profiles in Australian youth swimming.
Methods
Participants were N = 866 female 100-m (m) Front-Crawl 100-m swimmers, aged 9–15 years, competing at 36 Australian regional-national level long course (50-m) events. At respective events, swim performance time was collated alongside, age, date of birth, and anthropometric measures to identify age group, relative age, and maturity status. Quadratic relative age and maturity status with 100-m performance regression trendlines were generated. Then, individual swim performances at a given relative age or maturity status were converted into percentile rank distributions and compared with raw (unadjusted) annual age-group performance percentile ranks.
Results
At a cohort level, initial testing confirmed relative age and maturity-adjusted percentile rankings were associated with general rank improvements for relatively younger and later maturing swimmers compared to raw ranks (and vice versa). When assessing individual swimmer PCM plots, where three percentile rank scores were compared and rank change threshold criteria applied, five PCM profile types were identified, namely: ‘Early Developing’ (19 %); ‘Later Developing’ (18 %); ‘Consistent’ (15 %); ‘Mixed’ (38 %) and ‘Counteracting’ (10 %). PCM plots helped identify developmentally (dis-)advantaged swimmers; specific factors leading to (dis-)advantage, and likely onward development trajectories.
Conclusions
Overall and with practical considerations, PCMs can improve the validity of youth athletic performance evaluation as well as inform athlete development programming.
MoreTranslated text
Key words
Maturation,Relative age effects,Skill evaluation,Talent identification,Sport performance
AI Read Science
Must-Reading Tree
Example
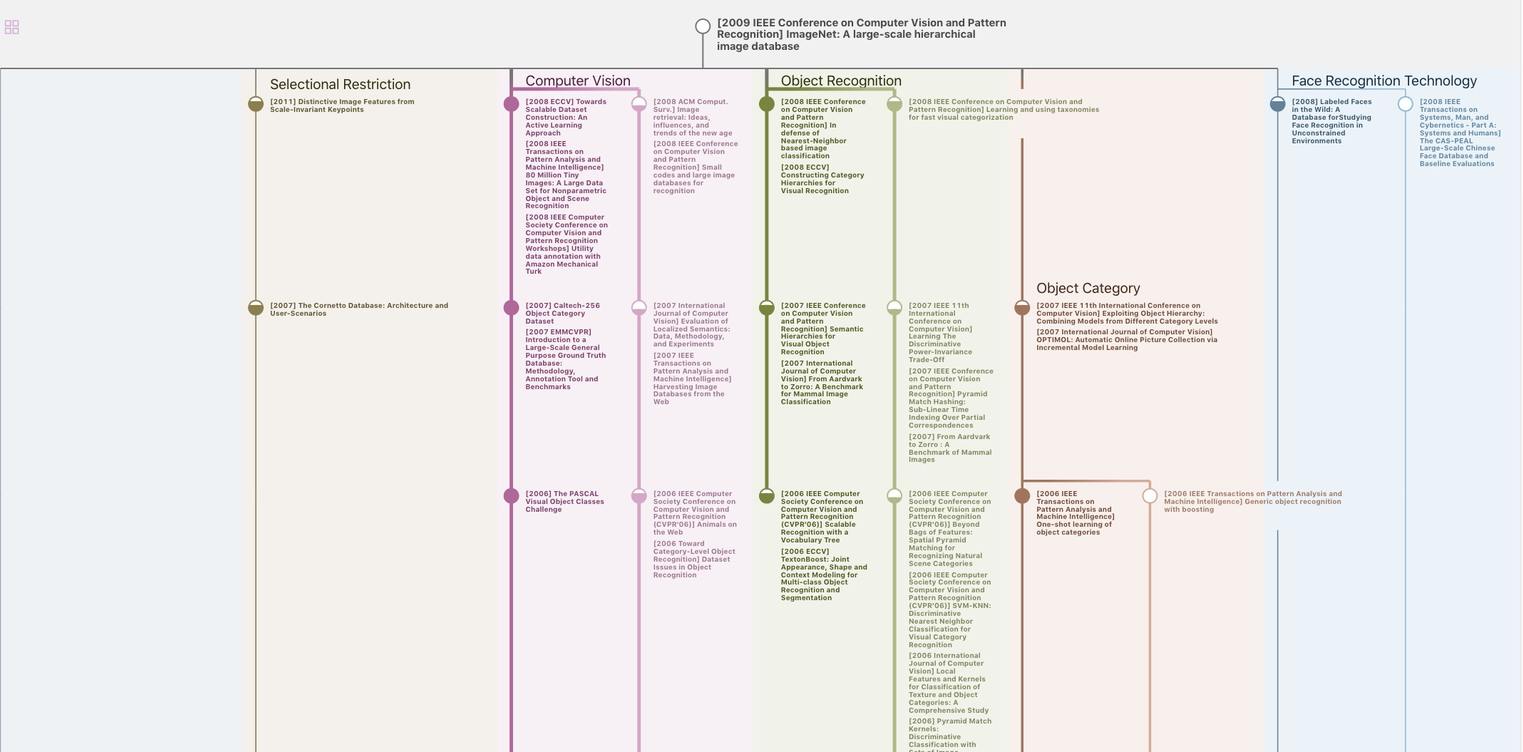
Generate MRT to find the research sequence of this paper
Chat Paper
Summary is being generated by the instructions you defined