Forecasting Hospital Room and Ward Occupancy Using Static and Dynamic Information Concurrently: Retrospective Single-Center Cohort Study
JMIR MEDICAL INFORMATICS(2024)
摘要
Background: Predicting the bed occupancy rate (BOR) is essential for efficient hospital resource management, long-term budget planning, and patient care planning. Although macro -level BOR prediction for the entire hospital is crucial, predicting occupancy at a detailed level, such as specific wards and rooms, is more practical and useful for hospital scheduling. Objective: The aim of this study was to develop a web -based support tool that allows hospital administrators to grasp the BOR for each ward and room according to different time periods. Methods: We trained time -series models based on long short-term memory (LSTM) using individual bed data aggregated hourly each day to predict the BOR for each ward and room in the hospital. Ward training involved 2 models with 7- and 30 -day time windows, and room training involved models with 3- and 7 -day time windows for shorter -term planning. To further improve prediction performance, we added 2 models trained by concatenating dynamic data with static data representing room -specific details. Results: We confirmed the results of a total of 12 models using bidirectional long short-term memory (Bi-LSTM) and LSTM, and the model based on Bi-LSTM showed better performance. The ward -level prediction model had a mean absolute error (MAE) of 0.067, mean square error (MSE) of 0.009, root mean square error (RMSE) of 0.094, and R2 score of 0.544. Among the room -level prediction models, the model that combined static data exhibited superior performance, with a MAE of 0.129, MSE of 0.050, RMSE of 0.227, and R2 score of 0.600. Model results can be displayed on an electronic dashboard for easy access via the web. Conclusions: We have proposed predictive BOR models for individual wards and rooms that demonstrate high performance. The results can be visualized through a web -based dashboard, aiding hospital administrators in bed operation planning. This contributes to resource optimization and the reduction of hospital resource use.
更多查看译文
关键词
hospital bed occupancy,electronic medical records,time series forecasting,short-term memory,combining static and dynamic variables
AI 理解论文
溯源树
样例
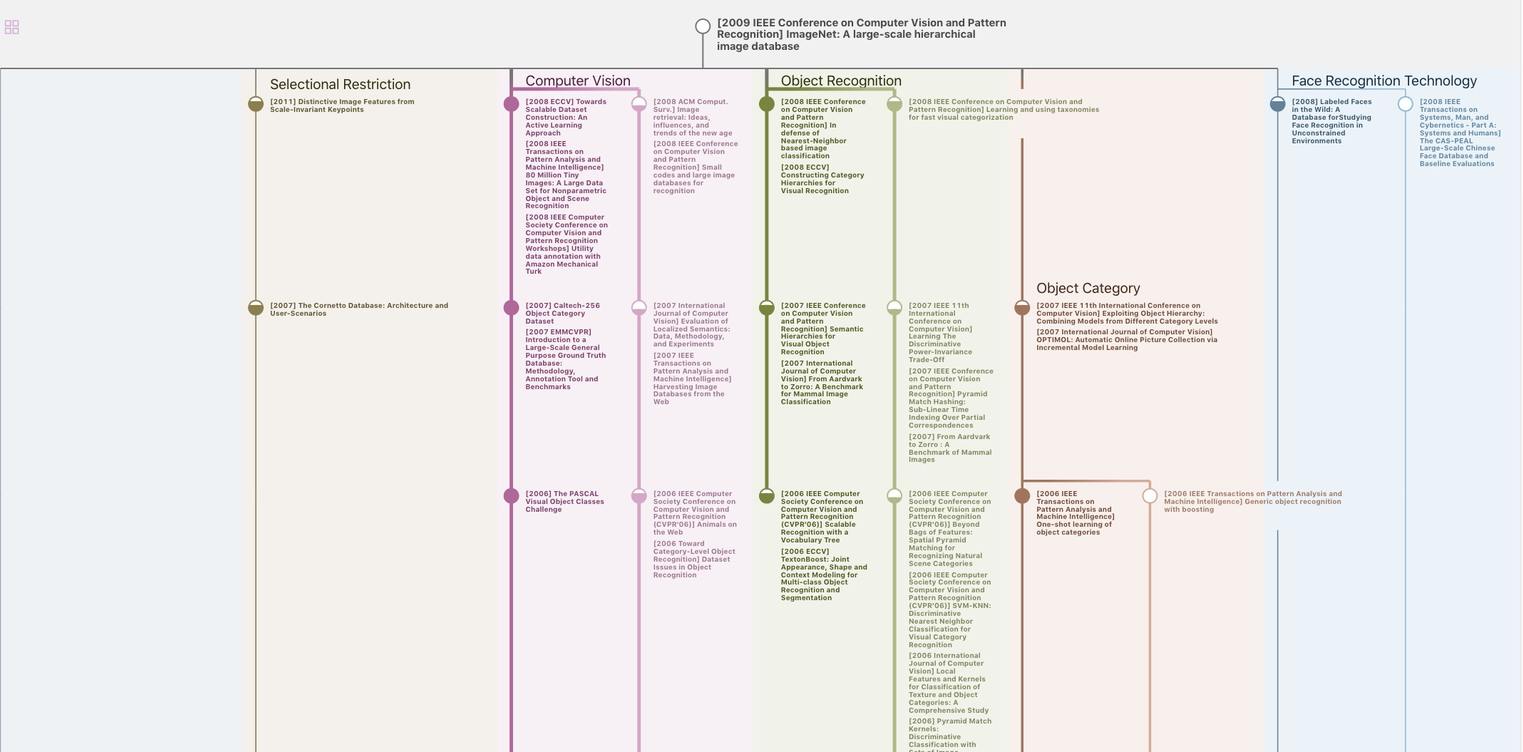
生成溯源树,研究论文发展脉络
Chat Paper
正在生成论文摘要