Causality-Driven Intra-class Non-equilibrium Label-Specific Features Learning
Neural Processing Letters(2024)
摘要
In multi-label learning, label-specific feature learning can effectively avoid some ineffectual features that interfere with the classification performance of the model. However, most of the existing label-specific feature learning algorithms improve the performance of the model for classification by constraining the solution space through label correlation. The non-equilibrium of the label distribution not only leads to some spurious correlations mixed in with the calculated label correlations but also diminishes the performance of the classification model. Causal learning can improve the classification performance and robustness of the model by capturing real causal relationships from limited data. Based on this, this paper proposes a causality-driven intra-class non-equilibrium label-specific features learning, named CNSF. Firstly, the causal relationship between the labels is learned by the Peter-Clark algorithm. Secondly, the label density of all instances is calculated by the intra-class non-equilibrium method, which is used to relieve the non-equilibrium distribution of original labels. Then, the correlation of the density matrix is calculated using cosine similarity and combined with causality to construct the causal density correlation matrix, to solve the problem of spurious correlation mixed in the label correlation obtained by traditional methods. Finally, the causal density correlation matrix is used to induce label-specific feature learning. Compared with eight state-of-the-art multi-label algorithms on thirteen datasets, the experimental results prove the reasonability and effectiveness of the algorithms in this paper.
更多查看译文
关键词
Label-specific feature learning,Causal relationship,Intra-class non-equilibrium,Density matrix,Label correlation
AI 理解论文
溯源树
样例
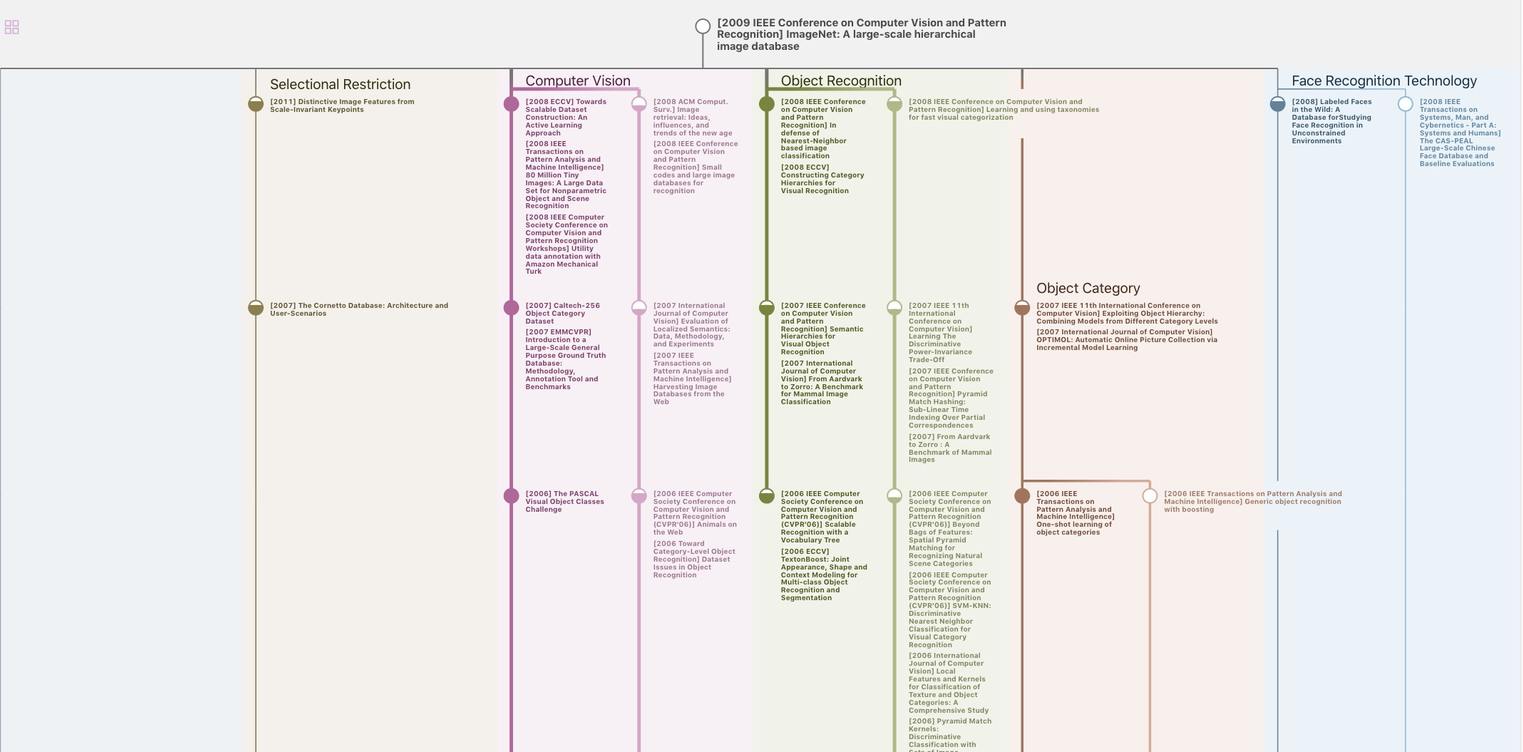
生成溯源树,研究论文发展脉络
Chat Paper
正在生成论文摘要