MiniPFL: Mini federations for hierarchical personalized federated learning
Future Generation Computer Systems(2024)
摘要
Personalized federated learning trains personalized models tailored to meet individual client’s specific data distributions. However, global models often introduce irrelevant information into personalized models, reducing communication efficiency and accuracy. We propose MiniPFL, a bi-component framework that selectively prioritizes valuable clients for personalized learning. The first component, a shallow layer holds information that is similar across clients, making it ideal for managing traditional federated aggregations. The second component, a deep layer, harbors more personalized information and allows identifying beneficial clients through mini-federations. Extensive experiments on CIFAR-10, CIFAR-100, and Tiny-Imagenet datasets using the Resnet18 architecture in Pytorch demonstrated that MiniPFL reduces communication rounds by 30% and increases accuracy by 2.7% compared to state-of-the-art methods.
更多查看译文
关键词
Federated learning,Two-stage layered approach,Hierarchical architecture,Heterogeneous data distribution,Personalized models
AI 理解论文
溯源树
样例
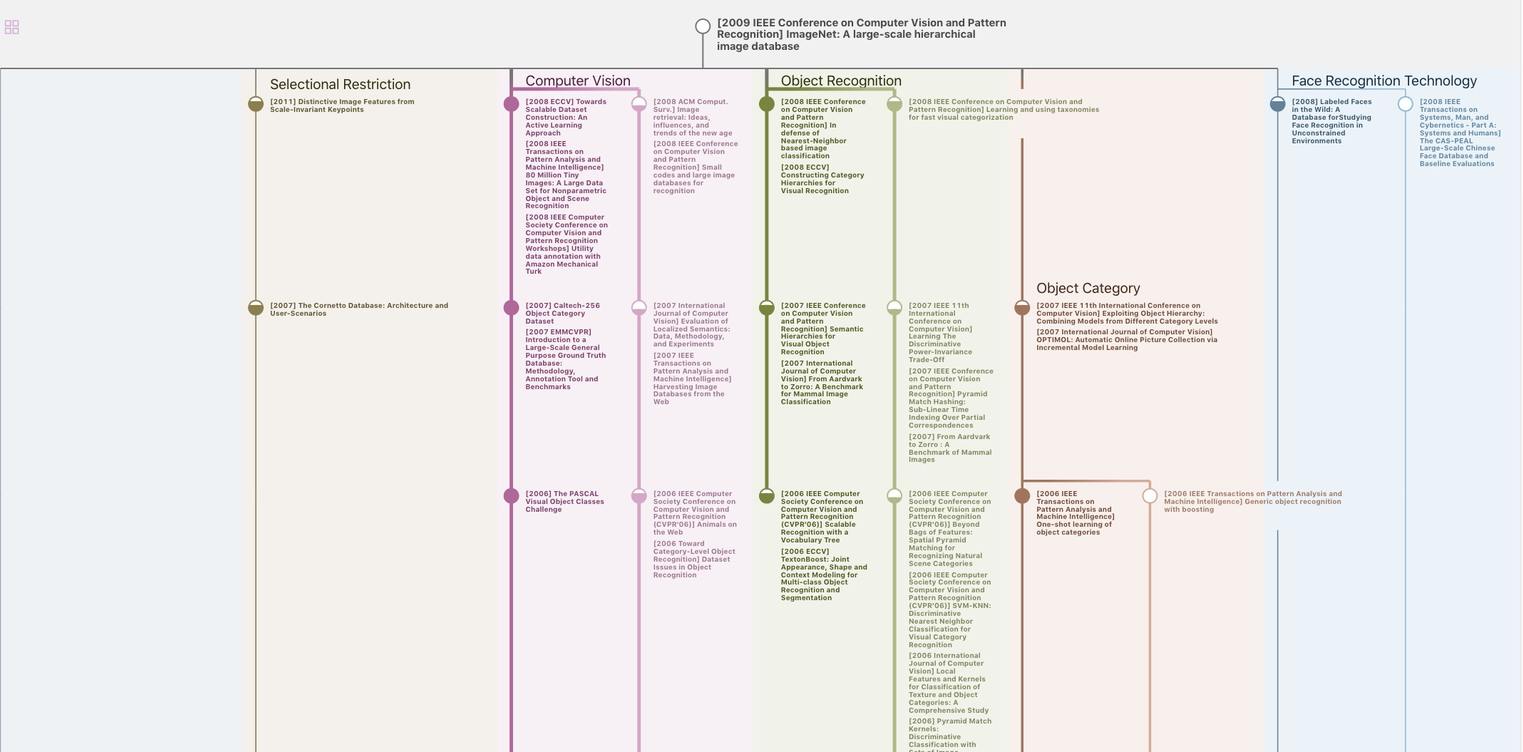
生成溯源树,研究论文发展脉络
Chat Paper
正在生成论文摘要