Smart Soft Sensor for Froth flotation quality monitoring using pre-trained feature extractor and Vision Transformer ViT.
SITIS(2023)
Abstract
Smart sensor technologies have gained prominence in the field of machine vision-based applications. Within this context, the utilization of machine vision systems for monitoring and controlling flotation plants has become increasingly prevalent. This paper addresses the critical need for accurate froth image classification in the development of an online machine vision-based control system. This paper explores the recent advancements in machine learning, with a focus on deep learning techniques. Specifically, it introduces the application of a Vision Transformer (VIT) model as an alternative to the traditional Convolutional Neural Network (CNN) for classifying froth images collected from an industrial lead flotation circuit. The results of this research demonstrate the remarkable performance of the ViT model, achieving an impressive overall accuracy rate of 96% when combined with pre-trained feature extractor models. This achievement surpasses the capabilities of conventional artificial neural network (CNN) classifiers of the state of art in terms of both accuracy and computational efficiency. These findings underscore the promising potential of deep learning neural networks in froth image analysis, highlighting their significance in the advancement of smart sensor-based machine vision systems within the field of flotation plant control.
MoreTranslated text
AI Read Science
Must-Reading Tree
Example
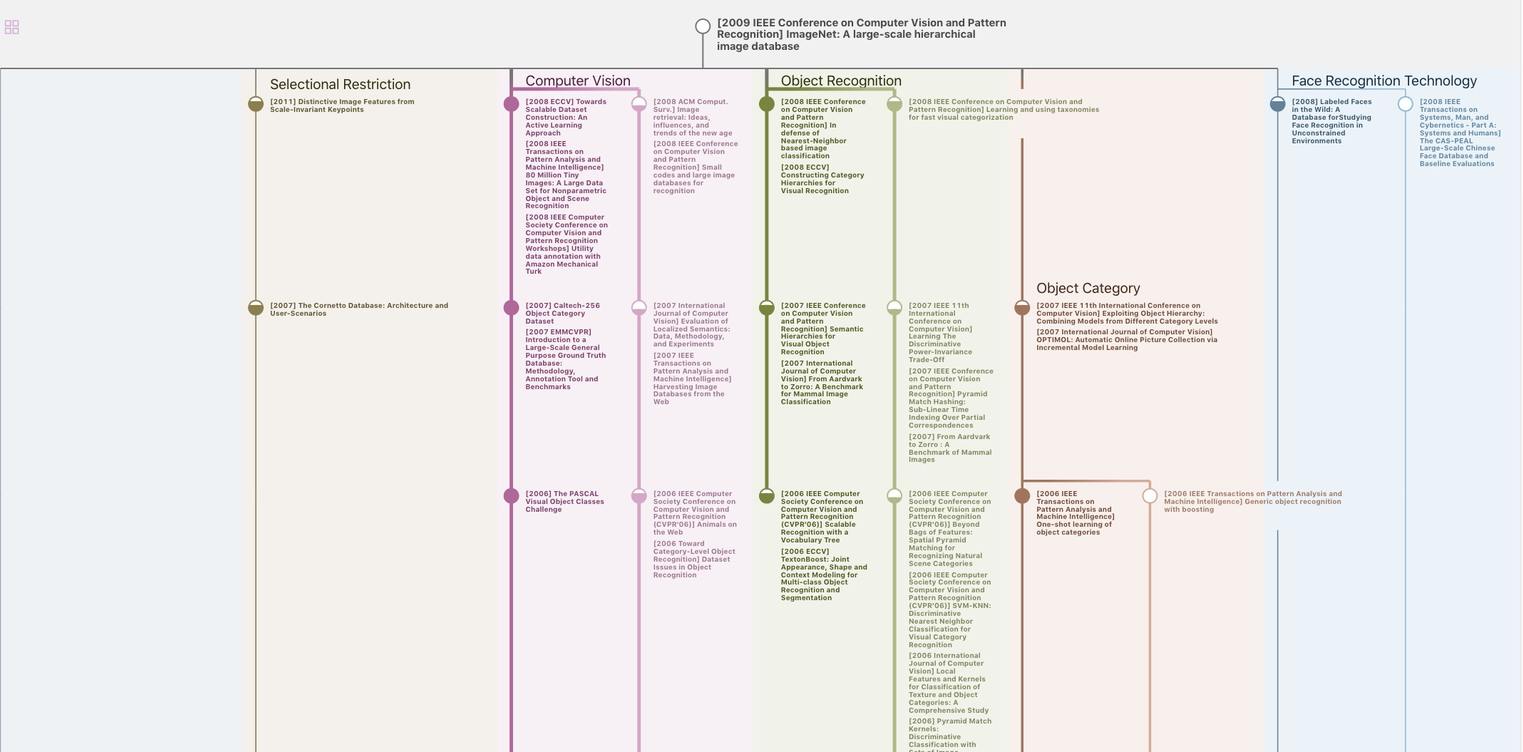
Generate MRT to find the research sequence of this paper
Chat Paper
Summary is being generated by the instructions you defined