Online neural sliding mode control with guaranteed stability
International Journal of Control(2024)
Abstract
Model-based control systems allow engineers to leverage known system information in controllers, often resulting in improved control accuracy or robustness. However, these system models can change over time, be difficult to obtain for complex systems, or fail to capture higher-order effects in the system. In this work, we use a highly robust model-based control methodology, sliding mode control, without the need for an a priori model. We use a neural network to learn an approximate system model fully online, with no pretraining. The neural network term is found to increase control accuracy while alleviating chatter, the primary drawback of conventional sliding mode control. The controller is verified by simulating a non-planar 3 degree-of-freedom robotic arm picking up a load, and the proposed controller is shown to outperform conventional model-based sliding mode control, especially when the built-in load margin on the end effector in the conventional model-based controller is exceeded.
MoreTranslated text
Key words
Adaptive control,neural networks,learning-based control,online learning,sliding mode control
AI Read Science
Must-Reading Tree
Example
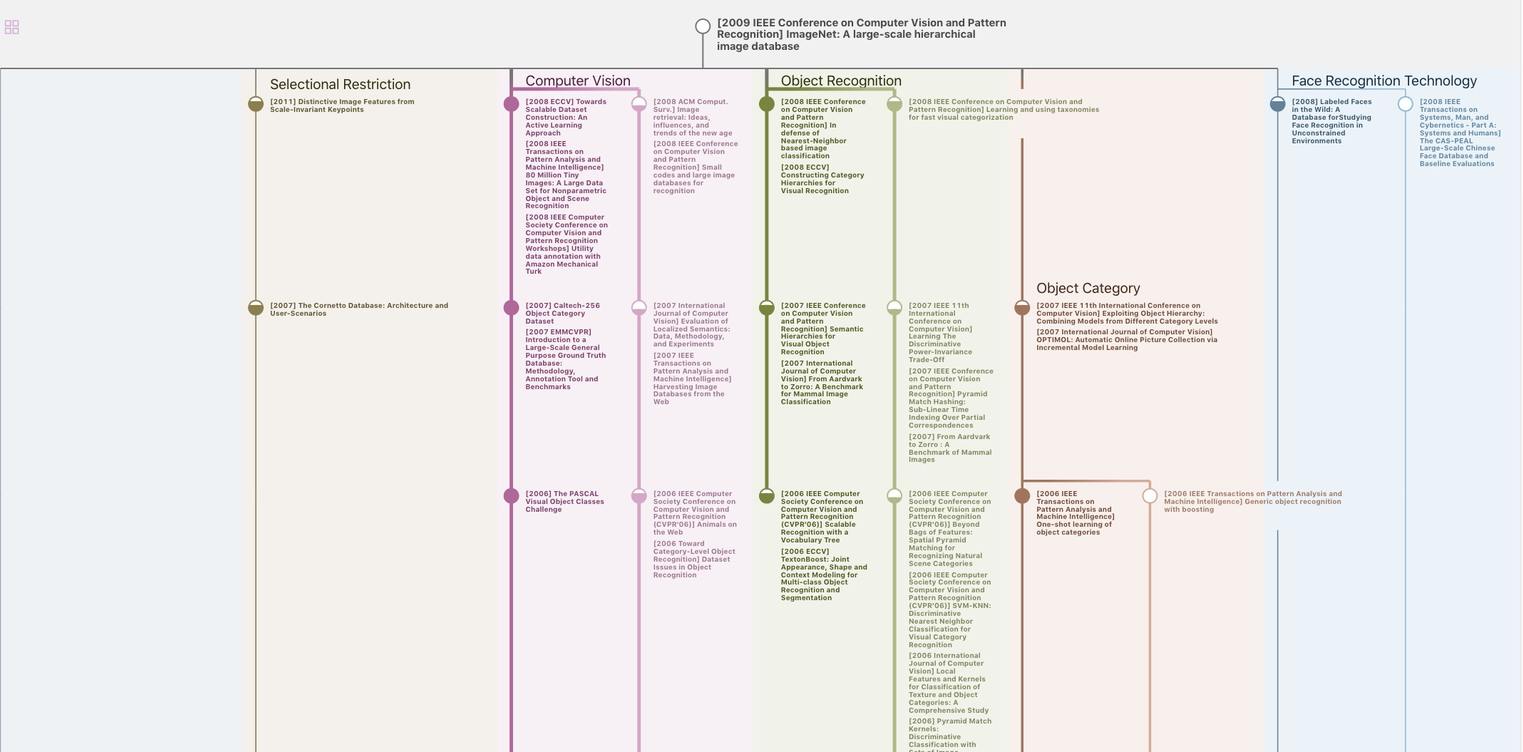
Generate MRT to find the research sequence of this paper
Chat Paper
Summary is being generated by the instructions you defined