Enhancing malware detection performance: leveraging K-Nearest Neighbors with Firefly Optimization Algorithm
Multimedia Tools and Applications(2024)
摘要
Malware detection plays a crucial role in ensuring robust cybersecurity amidst the ever-evolving cyber threats. This research paper delves into the realm of machine learning (ML) algorithms for malware detection, with a specific emphasis on the K-Nearest Neighbors (KNN) algorithm, utilizing tailored parameter settings and the Firefly Optimization Algorithm (FOA). The study leverages the MalMem-2022 dataset to assess the efficacy of KNN and KNN with FOA in malware detection. The impact of parameter tuning and feature selection on classification is elucidated by comparing the performance of both approaches. Encouragingly, the results reveal promising advancements in one of the multiclass classification scenarios when employing KNN with FOA, yielding noteworthy enhancements in Accuracy, Recall, Precision, Matthews Correlation Coefficient, TNR, and F1-score by 2.65
更多查看译文
关键词
Malware detection,K-Nearest Neighbors,Machine learning,Firefly algorithm
AI 理解论文
溯源树
样例
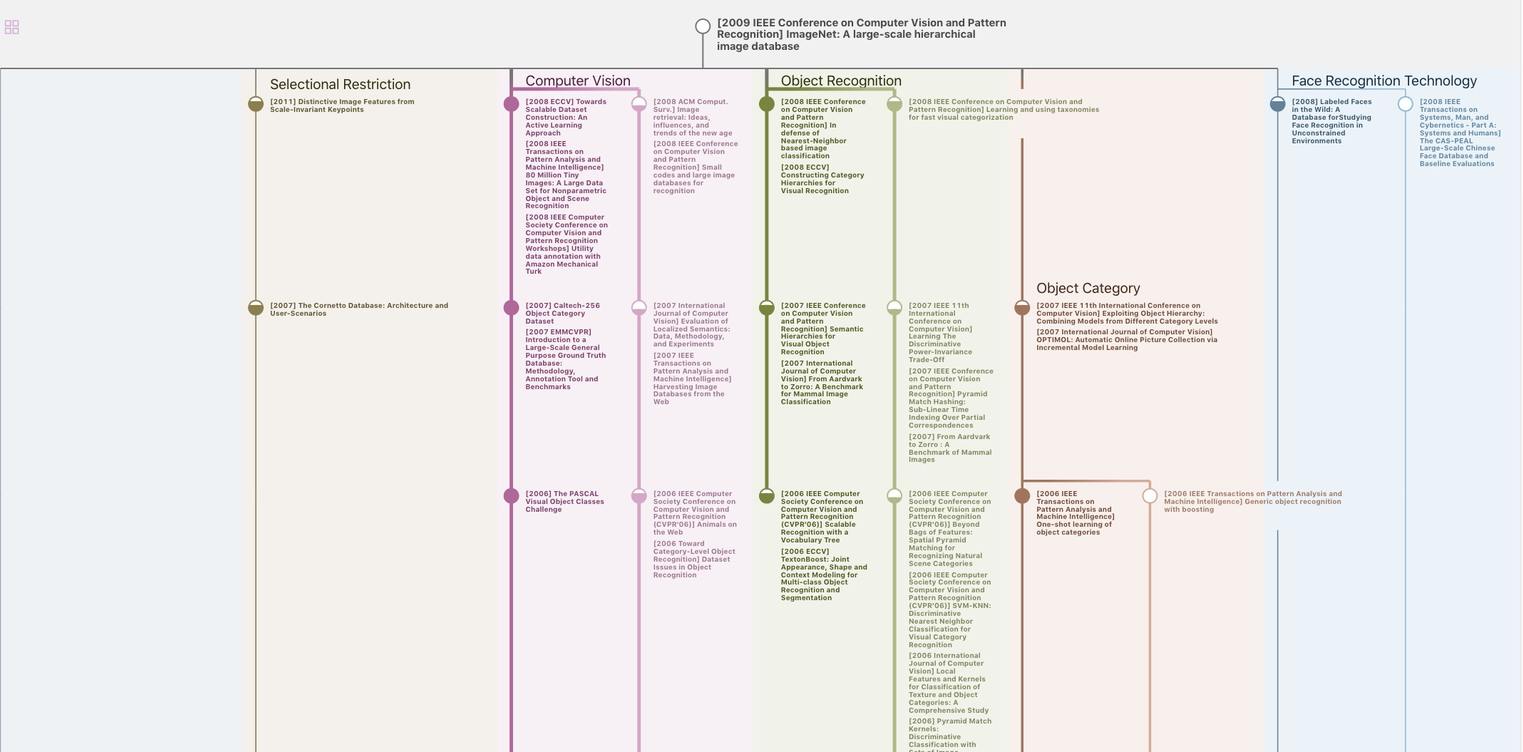
生成溯源树,研究论文发展脉络
Chat Paper
正在生成论文摘要