Detecting Semantic Uncertainty By Learning Hedge Cues In Sentences Using An Hmm
SOCIAL MEDIA CONTENT ANALYSIS: NATURAL LANGUAGE PROCESSING AND BEYOND(2014)
摘要
Detecting speculative assertions is essential to distinguish semantically uncertain information from the factual ones in text. This is critical to the trustworthiness of many intelligent systems that are based on information retrieval and natural language processing techniques, such as question answering or information extraction. We empirically explore three fundamental issues of uncertainty detection: (1) the predictive ability of different learning methods on this task; (2) whether using unlabeled data can lead to a more accurate model; and (3) whether closed-domain training or cross-domain training is better. For these purposes, we adopt two statistical learning approaches to this problem: the commonly used bag-of-words model based on Naive Bayes, and the sequence labeling approach using a Hidden Markov Model (HMM). We empirically compare between our two approaches as well as externally compare with prior results on the CoNLL-2010 Shared Task 1.Overall, our results are promising: (1) on Wikipedia and biomedical datasets, the HMM model improves over Naive Bayes up to 17.4% and 29.0%, respectively, in terms of absolute F score; (2) compared to CoNLL-2010 systems, our best HMM model achieves 62.9% F score with MLE parameter estimation and 64.0% with EM parameter estimation on Wikipedia dataset, both outperforming the best result (60.2%) of the CoNLL-2010 systems, but our results on the biomedical dataset are less impressive; (3) when the expression ability of a model (e.g., Naive Bayes) is not strong enough, cross-domain training is helpful, and when a model is powerful (e.g., HMM), cross-domain training may produce biased parameters; and (4) under Maximum Likelihood Estimation, combining the unlabeled examples with the labeled helps.This chapter has been published as a conference paper in the SIGIR 2014 Workshop on Semantic Matching in Information Retrieval (SMIR 2014) [11].
更多查看译文
AI 理解论文
溯源树
样例
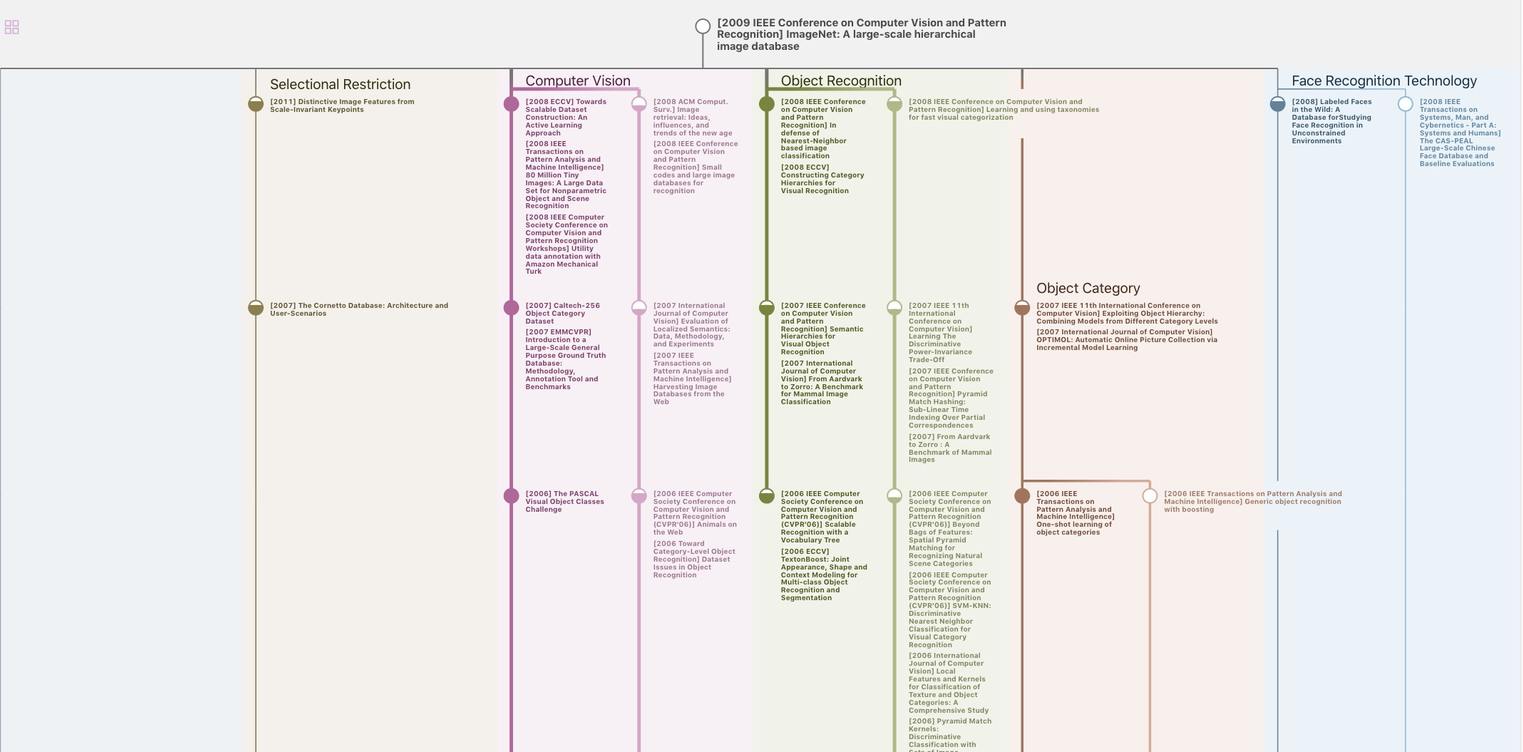
生成溯源树,研究论文发展脉络
Chat Paper
正在生成论文摘要