Prediction and analysis etching model of anti-glare glass roughness based on machine learning method
CHEMICAL ENGINEERING RESEARCH & DESIGN(2024)
Abstract
Anti-glare glass, renowned for its exceptional anti-glare properties, has attracted substantial research interest for its wide application in the electronic displays. Glass etching is the key step of the anti-glare glass production, but the formula optimization of this process depends on a numerous factors. In this work, the research focus is on optimizing the composition of etching solution and duration of etching to achieve a desired roughness, recorded at 137.80 nm in our experiment. This study also introduces an innovative approach that integrates experimental etching data with machine learning model predictions. The etch dataset was collected from the experimental etching data, using the etch component and etching duration as the featured inputs, with the resultant glass surface roughness as the target output. Aided by the Random Forest algorithm, how these etching variables influence surface roughness were analyzed and predicted. The accuracy and feasibility of this method are verified by experimental validation, allowing accurate predictions of glass surface roughness. The R 2 of the model reaches 0.9165, and RSME is only 22.6690. In addition, the feature importance analysis shows that sorbitol and etching time are the most important factors affecting the roughness, contributing 56.6% and 32.3% to the model ' s accuracy, respectively.
MoreTranslated text
Key words
Anti-glare glass roughness,Glass etching,Formula optimization,Machine learning,Model prediction
AI Read Science
Must-Reading Tree
Example
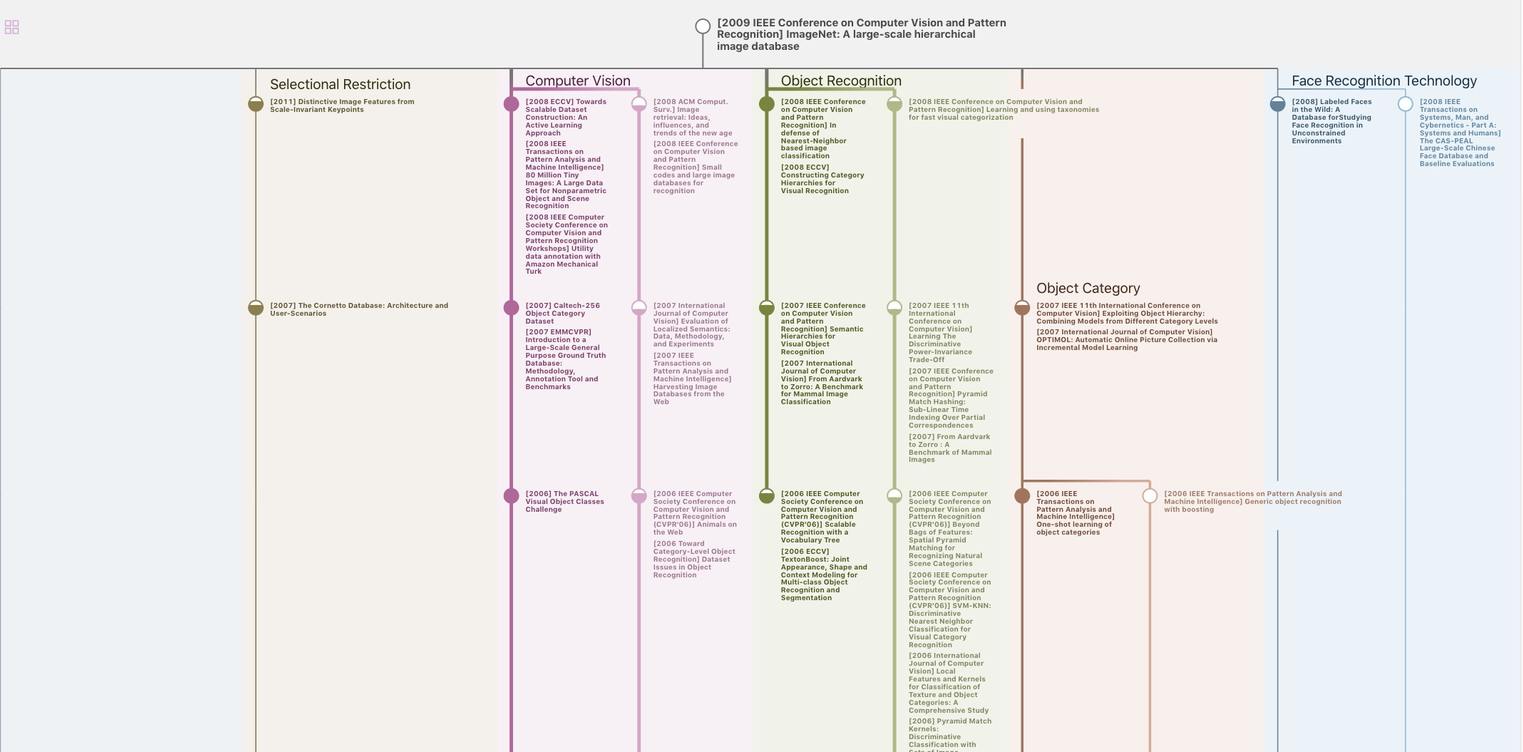
Generate MRT to find the research sequence of this paper
Chat Paper
Summary is being generated by the instructions you defined